Liliana Borcea - Waveform inversion via reduced order modeling
Prof. Liliana Borcea from the the University of Michigan speaking in the UW Data-driven methods in science and engineering seminar on Mar. 4, 2022.
Sign up for notifications of future talks: https://mailman11.u.washington.edu/mailman/listinfo/datadriven-seminar
Abstract: This talk is concerned with the following inverse problem for the wave equation: Determine the variable wave speed from data gathered by a collection of sensors, which emit probing signals and measure the generated backscattered waves. Inverse backscattering is an interdisciplinary field driven by applications in geophysical exploration, radar imaging, non-destructive evaluation of materials, etc. There are two types of methods:(1) Qualitative (imaging) methods, which address the simpler problem of locating reflective structures in a known host medium. (2) Quantitative methods, also known as velocity estimation. Typically, velocity estimation is formulated as a PDE constrained optimization, where the data are fit in the least squares sense by the wave computed at the search wave speed. The increase in computing power has lead to growing interest in this approach, but there is a fundamental impediment, which manifests especially for high frequency data: The objective function is not convex and has numerous local minima even in the absence of noise. The main goal of the talk is to introduce a novel approach to velocity estimation, based on a reduced order model (ROM) of the wave operator. The ROM is called data driven because it is obtained from the measurements made at the sensors. The mapping between these measurements and the ROM is nonlinear, and yet the ROM can be computed efficiently using methods from numerical linear algebra. More importantly, the ROM can be used to define a better objective function for velocity estimation, so that gradient based optimization can succeed even for a poor initial guess.
Видео Liliana Borcea - Waveform inversion via reduced order modeling канала Physics Informed Machine Learning
Sign up for notifications of future talks: https://mailman11.u.washington.edu/mailman/listinfo/datadriven-seminar
Abstract: This talk is concerned with the following inverse problem for the wave equation: Determine the variable wave speed from data gathered by a collection of sensors, which emit probing signals and measure the generated backscattered waves. Inverse backscattering is an interdisciplinary field driven by applications in geophysical exploration, radar imaging, non-destructive evaluation of materials, etc. There are two types of methods:(1) Qualitative (imaging) methods, which address the simpler problem of locating reflective structures in a known host medium. (2) Quantitative methods, also known as velocity estimation. Typically, velocity estimation is formulated as a PDE constrained optimization, where the data are fit in the least squares sense by the wave computed at the search wave speed. The increase in computing power has lead to growing interest in this approach, but there is a fundamental impediment, which manifests especially for high frequency data: The objective function is not convex and has numerous local minima even in the absence of noise. The main goal of the talk is to introduce a novel approach to velocity estimation, based on a reduced order model (ROM) of the wave operator. The ROM is called data driven because it is obtained from the measurements made at the sensors. The mapping between these measurements and the ROM is nonlinear, and yet the ROM can be computed efficiently using methods from numerical linear algebra. More importantly, the ROM can be used to define a better objective function for velocity estimation, so that gradient based optimization can succeed even for a poor initial guess.
Видео Liliana Borcea - Waveform inversion via reduced order modeling канала Physics Informed Machine Learning
Показать
Комментарии отсутствуют
Информация о видео
11 марта 2022 г. 22:03:47
01:08:22
Другие видео канала
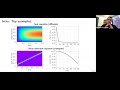
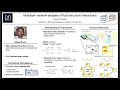
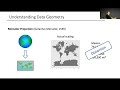
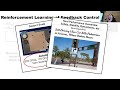
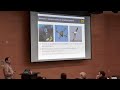
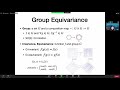
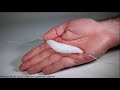

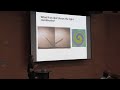
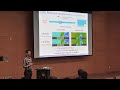

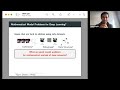
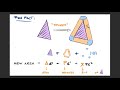
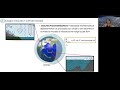
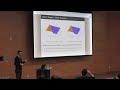
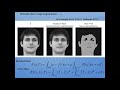
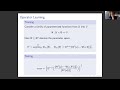
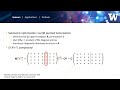
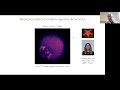
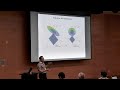
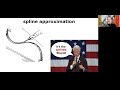