Self-Attention with Relative Position Representations – Paper explained
We help you wrap your head around relative positional embeddings as they were first introduced in the “Self-Attention with Relative Position Representations” paper.
➡️ AI Coffee Break Merch! 🛍️ https://aicoffeebreak.creator-spring.com/
Related videos:
📺 Positional embeddings explained: https://youtu.be/1biZfFLPRSY
📺 Concatenated, learned positional encodings: https://youtu.be/M2ToEXF6Olw
📺 Transformer explained: https://youtu.be/FWFA4DGuzSc
Papers:
📄 Shaw, Peter, Jakob Uszkoreit, and Ashish Vaswani. "Self-Attention with Relative Position Representations." In Proceedings of the 2018 Conference of the North American Chapter of the Association for Computational Linguistics: Human Language Technologies, Volume 2 (Short Papers), pp. 464-468. 2018. https://arxiv.org/pdf/1803.02155.pdf
📄 Vaswani, Ashish, Noam Shazeer, Niki Parmar, Jakob Uszkoreit, Llion Jones, Aidan N. Gomez, Łukasz Kaiser, and Illia Polosukhin. "Attention is all you need." In Advances in neural information processing systems, pp. 5998-6008. 2017. https://proceedings.neurips.cc/paper/2017/file/3f5ee243547dee91fbd053c1c4a845aa-Paper.pdf
💻 Implementation for Relative Position Embeddings: https://github.com/AliHaiderAhmad001/Self-Attention-with-Relative-Position-Representations
Outline:
00:00 Relative positional representations
02:15 How do they work?
07:59 Benefits of relative vs. absolute positional encodings
Music 🎵 : Holi Day Riddim - Konrad OldMoney
✍️ Arabic Subtitles by Ali Haidar Ahmad https://www.linkedin.com/in/ali-ahmad-0706a51bb/ .
▀▀▀▀▀▀▀▀▀▀▀▀▀▀▀▀▀▀▀▀▀▀▀▀▀▀
🔥 Optionally, pay us a coffee to help with our Coffee Bean production! ☕
Patreon: https://www.patreon.com/AICoffeeBreak
Ko-fi: https://ko-fi.com/aicoffeebreak
▀▀▀▀▀▀▀▀▀▀▀▀▀▀▀▀▀▀▀▀▀▀▀▀▀▀
🔗 Links:
AICoffeeBreakQuiz: https://www.youtube.com/c/AICoffeeBreak/community
Twitter: https://twitter.com/AICoffeeBreak
Reddit: https://www.reddit.com/r/AICoffeeBreak/
YouTube: https://www.youtube.com/AICoffeeBreak
#AICoffeeBreak #MsCoffeeBean #MachineLearning #AI #research
Видео Self-Attention with Relative Position Representations – Paper explained канала AI Coffee Break with Letitia
➡️ AI Coffee Break Merch! 🛍️ https://aicoffeebreak.creator-spring.com/
Related videos:
📺 Positional embeddings explained: https://youtu.be/1biZfFLPRSY
📺 Concatenated, learned positional encodings: https://youtu.be/M2ToEXF6Olw
📺 Transformer explained: https://youtu.be/FWFA4DGuzSc
Papers:
📄 Shaw, Peter, Jakob Uszkoreit, and Ashish Vaswani. "Self-Attention with Relative Position Representations." In Proceedings of the 2018 Conference of the North American Chapter of the Association for Computational Linguistics: Human Language Technologies, Volume 2 (Short Papers), pp. 464-468. 2018. https://arxiv.org/pdf/1803.02155.pdf
📄 Vaswani, Ashish, Noam Shazeer, Niki Parmar, Jakob Uszkoreit, Llion Jones, Aidan N. Gomez, Łukasz Kaiser, and Illia Polosukhin. "Attention is all you need." In Advances in neural information processing systems, pp. 5998-6008. 2017. https://proceedings.neurips.cc/paper/2017/file/3f5ee243547dee91fbd053c1c4a845aa-Paper.pdf
💻 Implementation for Relative Position Embeddings: https://github.com/AliHaiderAhmad001/Self-Attention-with-Relative-Position-Representations
Outline:
00:00 Relative positional representations
02:15 How do they work?
07:59 Benefits of relative vs. absolute positional encodings
Music 🎵 : Holi Day Riddim - Konrad OldMoney
✍️ Arabic Subtitles by Ali Haidar Ahmad https://www.linkedin.com/in/ali-ahmad-0706a51bb/ .
▀▀▀▀▀▀▀▀▀▀▀▀▀▀▀▀▀▀▀▀▀▀▀▀▀▀
🔥 Optionally, pay us a coffee to help with our Coffee Bean production! ☕
Patreon: https://www.patreon.com/AICoffeeBreak
Ko-fi: https://ko-fi.com/aicoffeebreak
▀▀▀▀▀▀▀▀▀▀▀▀▀▀▀▀▀▀▀▀▀▀▀▀▀▀
🔗 Links:
AICoffeeBreakQuiz: https://www.youtube.com/c/AICoffeeBreak/community
Twitter: https://twitter.com/AICoffeeBreak
Reddit: https://www.reddit.com/r/AICoffeeBreak/
YouTube: https://www.youtube.com/AICoffeeBreak
#AICoffeeBreak #MsCoffeeBean #MachineLearning #AI #research
Видео Self-Attention with Relative Position Representations – Paper explained канала AI Coffee Break with Letitia
Показать
Комментарии отсутствуют
Информация о видео
31 июля 2021 г. 23:15:49
00:10:18
Другие видео канала
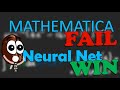
![[Quiz] Eigenfaces, Domain adaptation, Causality, Manifold Hypothesis, Denoising Autoencoder](https://i.ytimg.com/vi/yPXNQ6Ig7hQ/default.jpg)
![[RANT] Adversarial attack on OpenAI’s CLIP? Are we the fools or the foolers?](https://i.ytimg.com/vi/xqdHfLrevuo/default.jpg)
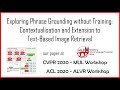
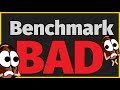
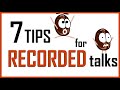
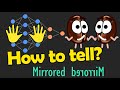
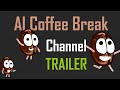
![[Quiz] Interpretable ML, VQ-VAE w/o Quantization / infinite codebook, Pearson’s, PointClouds](https://i.ytimg.com/vi/KS7UlN9SCg4/default.jpg)

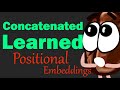
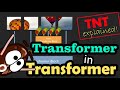

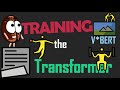
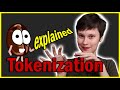
![[Quiz] Regularization in Deep Learning, Lipschitz continuity, Gradient regularization](https://i.ytimg.com/vi/zAyDhZFup9k/default.jpg)
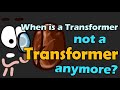
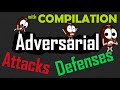
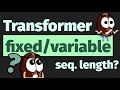
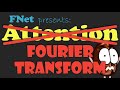
