Data BAD | What Will it Take to Fix Benchmarking for NLU?
The Coffee Bean explains and comments the sobering take of the paper "What Will it Take to Fix Benchmarking in Natural Language Understanding?"
See more videos from Ms. Coffee Bean about natural language understanding:
📺 The road to NLU: https://youtube.com/playlist?list=PLpZBeKTZRGPMjF-Ob-NYjaTtewbMNXKcU
► Thanks to our Patrons who support us in Tier 2, 3, 4: 🙏
donor, Dres. Trost GbR, Yannik Schneider
➡️ AI Coffee Break Merch! 🛍️ https://aicoffeebreak.creator-spring.com/
Paper:
📜 Bowman, Samuel R., and George E. Dahl. "What Will it Take to Fix Benchmarking in Natural Language Understanding?." arXiv preprint arXiv:2104.02145 (2021). https://arxiv.org/abs/2104.02145
🔗 SuperGLUE: https://super.gluebenchmark.com/tasks
🔗 WiC: The Word-in-Context Dataset (English): https://pilehvar.github.io/wic/
Outline:
00:00 NLU Benchmarking – Motivation
01:04 How to measure NLU advances?
02:31 Why is NLU benchmarking broken?
04:43 What are the fixes?
▀▀▀▀▀▀▀▀▀▀▀▀▀▀▀▀▀▀▀▀▀▀▀▀▀▀
🔥 Optionally, pay us a coffee to help with our Coffee Bean production! ☕
Patreon: https://www.patreon.com/AICoffeeBreak
Ko-fi: https://ko-fi.com/aicoffeebreak
▀▀▀▀▀▀▀▀▀▀▀▀▀▀▀▀▀▀▀▀▀▀▀▀▀▀
🔗 Links:
AICoffeeBreakQuiz: https://www.youtube.com/c/AICoffeeBreak/community
Twitter: https://twitter.com/AICoffeeBreak
Reddit: https://www.reddit.com/r/AICoffeeBreak/
YouTube: https://www.youtube.com/AICoffeeBreak
#AICoffeeBreak #MsCoffeeBean #MachineLearning #AI #research
Видео Data BAD | What Will it Take to Fix Benchmarking for NLU? канала AI Coffee Break with Letitia
See more videos from Ms. Coffee Bean about natural language understanding:
📺 The road to NLU: https://youtube.com/playlist?list=PLpZBeKTZRGPMjF-Ob-NYjaTtewbMNXKcU
► Thanks to our Patrons who support us in Tier 2, 3, 4: 🙏
donor, Dres. Trost GbR, Yannik Schneider
➡️ AI Coffee Break Merch! 🛍️ https://aicoffeebreak.creator-spring.com/
Paper:
📜 Bowman, Samuel R., and George E. Dahl. "What Will it Take to Fix Benchmarking in Natural Language Understanding?." arXiv preprint arXiv:2104.02145 (2021). https://arxiv.org/abs/2104.02145
🔗 SuperGLUE: https://super.gluebenchmark.com/tasks
🔗 WiC: The Word-in-Context Dataset (English): https://pilehvar.github.io/wic/
Outline:
00:00 NLU Benchmarking – Motivation
01:04 How to measure NLU advances?
02:31 Why is NLU benchmarking broken?
04:43 What are the fixes?
▀▀▀▀▀▀▀▀▀▀▀▀▀▀▀▀▀▀▀▀▀▀▀▀▀▀
🔥 Optionally, pay us a coffee to help with our Coffee Bean production! ☕
Patreon: https://www.patreon.com/AICoffeeBreak
Ko-fi: https://ko-fi.com/aicoffeebreak
▀▀▀▀▀▀▀▀▀▀▀▀▀▀▀▀▀▀▀▀▀▀▀▀▀▀
🔗 Links:
AICoffeeBreakQuiz: https://www.youtube.com/c/AICoffeeBreak/community
Twitter: https://twitter.com/AICoffeeBreak
Reddit: https://www.reddit.com/r/AICoffeeBreak/
YouTube: https://www.youtube.com/AICoffeeBreak
#AICoffeeBreak #MsCoffeeBean #MachineLearning #AI #research
Видео Data BAD | What Will it Take to Fix Benchmarking for NLU? канала AI Coffee Break with Letitia
Показать
Комментарии отсутствуют
Информация о видео
10 октября 2021 г. 17:00:12
00:12:56
Другие видео канала
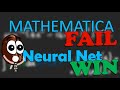
![[Quiz] Eigenfaces, Domain adaptation, Causality, Manifold Hypothesis, Denoising Autoencoder](https://i.ytimg.com/vi/yPXNQ6Ig7hQ/default.jpg)
![[RANT] Adversarial attack on OpenAI’s CLIP? Are we the fools or the foolers?](https://i.ytimg.com/vi/xqdHfLrevuo/default.jpg)
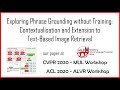
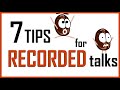
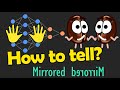
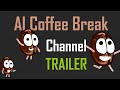
![[Quiz] Interpretable ML, VQ-VAE w/o Quantization / infinite codebook, Pearson’s, PointClouds](https://i.ytimg.com/vi/KS7UlN9SCg4/default.jpg)

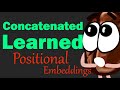
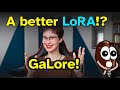
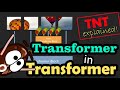

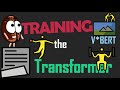
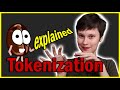
![[Quiz] Regularization in Deep Learning, Lipschitz continuity, Gradient regularization](https://i.ytimg.com/vi/zAyDhZFup9k/default.jpg)
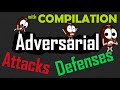
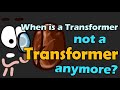
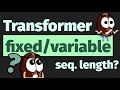
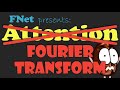