Hyperbolic Deep Learning [in Russian]
Slides: http://bayesgroup.github.io/bmml_sem/2020/Kochurov_Hyperbolic%20Deep%20Learning.pdf
Chat: https://drive.google.com/file/d/1n4afS4WvHwGDsiaUHPWDuddc5Px_BGva/view?usp=sharing
Hyperbolic Deep Learning gained attention due to its ability to work with and represent hierarchical relations. However, we do not yet have enough tools to work in non-Euclidean space. Several works present proof of concept results on various tasks: word embeddings, text classification, node classification, link prediction, and others. Methods discussed include Hyperbolic GloVe, GRU, VAE, graph embeddings, and graph neural networks. These works introduce new concepts and link Euclidean models to their Hyperbolic extensions. While having fairly simple baselines, they provide some evidence where Hyperbolic geometry might be more suitable.
Видео Hyperbolic Deep Learning [in Russian] канала BayesGroup.ru
Chat: https://drive.google.com/file/d/1n4afS4WvHwGDsiaUHPWDuddc5Px_BGva/view?usp=sharing
Hyperbolic Deep Learning gained attention due to its ability to work with and represent hierarchical relations. However, we do not yet have enough tools to work in non-Euclidean space. Several works present proof of concept results on various tasks: word embeddings, text classification, node classification, link prediction, and others. Methods discussed include Hyperbolic GloVe, GRU, VAE, graph embeddings, and graph neural networks. These works introduce new concepts and link Euclidean models to their Hyperbolic extensions. While having fairly simple baselines, they provide some evidence where Hyperbolic geometry might be more suitable.
Видео Hyperbolic Deep Learning [in Russian] канала BayesGroup.ru
Показать
Комментарии отсутствуют
Информация о видео
Другие видео канала
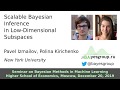
![Autoformer and Autoregressive Denoising Diffusion Models for Time Series Forecasting [in Russian]](https://i.ytimg.com/vi/8ASpS53J-PQ/default.jpg)
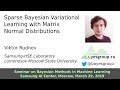
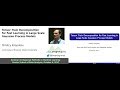
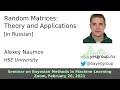
![Neural Program Synthesis, part 2 [in Russian]](https://i.ytimg.com/vi/3T49dB6dG4g/default.jpg)
![Tensor Programs, part 2 [in Russian]](https://i.ytimg.com/vi/AQ-JCTxWU9M/default.jpg)
![Discovering Faster Matrix Multiplication Algorithms with Reinforcement Learning [in Russian]](https://i.ytimg.com/vi/znLybIpcaLQ/default.jpg)
![Learning Differential Equations that are easy to solve [in Russian]](https://i.ytimg.com/vi/2W-wrXBc7oo/default.jpg)
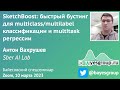
![On Power Laws in Deep Ensembles [in Russian]](https://i.ytimg.com/vi/lPku_0tq0Ho/default.jpg)
![[DeepBayes2019]: Day 5, Sponsor talk](https://i.ytimg.com/vi/X8vYS0oLgL4/default.jpg)
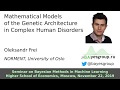
![Controlling GANs Latent Space [in Russian]](https://i.ytimg.com/vi/5lEJw4VagcA/default.jpg)
![[DeepBayes2019]: Day 2, practical session 2. Variational autoencoders](https://i.ytimg.com/vi/3MhSNduBwMw/default.jpg)
![Domain Adaptation of GANs [in Russian]](https://i.ytimg.com/vi/2GfaUtp_8R8/default.jpg)
![[DeepBayes2019]: Day 5, Lecture 1. Markov Chain Monte Carlo](https://i.ytimg.com/vi/Q_Bi2H9NKzc/default.jpg)
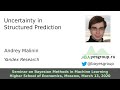
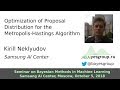
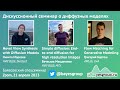