Controlling GANs Latent Space [in Russian]
Speaker: Vadim Titov, MIPT, AIRI
Slides: https://github.com/bayesgroup/bayesgroup.github.io/blob/master/bmml_sem/2021/Titov_Ganlatent.pdf
Modern GAN architectures generate highly realistic images in a variety of domains. Much recent works have focused on understanding how its latent space is connected with generated image semantic. It is discovered that there exist meaningful latent manipulations that allow to semantically edit image. Many proposed methods include supervision from pretrained models which is a strong limitation. This weakness could be eliminated by unsupervised methods that have their own disadvantages.
In this talk we will describe the main directions (supervised, unsupervised, text-guided) and current state-of-the-art methods of semantic image manipulation through GAN latent space.
Видео Controlling GANs Latent Space [in Russian] канала BayesGroup.ru
Slides: https://github.com/bayesgroup/bayesgroup.github.io/blob/master/bmml_sem/2021/Titov_Ganlatent.pdf
Modern GAN architectures generate highly realistic images in a variety of domains. Much recent works have focused on understanding how its latent space is connected with generated image semantic. It is discovered that there exist meaningful latent manipulations that allow to semantically edit image. Many proposed methods include supervision from pretrained models which is a strong limitation. This weakness could be eliminated by unsupervised methods that have their own disadvantages.
In this talk we will describe the main directions (supervised, unsupervised, text-guided) and current state-of-the-art methods of semantic image manipulation through GAN latent space.
Видео Controlling GANs Latent Space [in Russian] канала BayesGroup.ru
Показать
Информация о видео
15 февраля 2022 г. 10:30:00
00:59:27
Другие видео канала
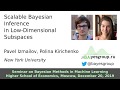
![Autoformer and Autoregressive Denoising Diffusion Models for Time Series Forecasting [in Russian]](https://i.ytimg.com/vi/8ASpS53J-PQ/default.jpg)
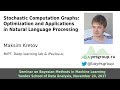
![[DeepBayes2018]: Day 2, lecture 4. Discrete latent variables](https://i.ytimg.com/vi/-KzvHc16HlM/default.jpg)
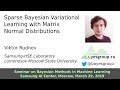
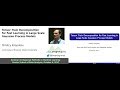
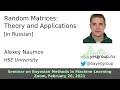
![Neural Program Synthesis, part 2 [in Russian]](https://i.ytimg.com/vi/3T49dB6dG4g/default.jpg)
![Hyperbolic Deep Learning [in Russian]](https://i.ytimg.com/vi/AoD_DUlMlAQ/default.jpg)
![Tensor Programs, part 2 [in Russian]](https://i.ytimg.com/vi/AQ-JCTxWU9M/default.jpg)
![Discovering Faster Matrix Multiplication Algorithms with Reinforcement Learning [in Russian]](https://i.ytimg.com/vi/znLybIpcaLQ/default.jpg)
![Learning Differential Equations that are easy to solve [in Russian]](https://i.ytimg.com/vi/2W-wrXBc7oo/default.jpg)
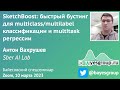
![[DeepBayes2018]: Day 3, Practical session 5. Distributional reinforcement learning](https://i.ytimg.com/vi/_Ve8Wd3Uyos/default.jpg)
![On Power Laws in Deep Ensembles [in Russian]](https://i.ytimg.com/vi/lPku_0tq0Ho/default.jpg)
![[DeepBayes2019]: Day 5, Sponsor talk](https://i.ytimg.com/vi/X8vYS0oLgL4/default.jpg)
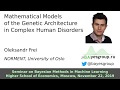
![[DeepBayes2019]: Day 2, practical session 2. Variational autoencoders](https://i.ytimg.com/vi/3MhSNduBwMw/default.jpg)

![Domain Adaptation of GANs [in Russian]](https://i.ytimg.com/vi/2GfaUtp_8R8/default.jpg)