Neural Search for Augmented Decision Making - Zeta Alpha - DRT S2E17
01:54 where did Jakub's journey begin
03:21 NLP in the 90's
04:19 Jakub's Resume analysis company
06:54 Selling TextKernel and starting Zeta Alpha
09:44 Initial assumption: deep learning researchers need a tool to keep track of advances in their field
11:27 Putting together a team to build a technical knowledge management product
15:31 Rise of large language models, augmented decision making, and enterprise search
20:07 Does finding documents for people (search) solve the problem that users have?
22:37 Reading papers is a nightmare (and how GPT3 et al can start to tackle this)
25:38 Knowledge management as a human-machine-interface problem
29:47 Differences between what academic and industrial audience would want
32:49 Multi-task nature of large language models to rescue?
35:49 Are language models alone enough?
39:22 What's down the pipe for Zeta Alpha?
41:12 Verdicts and wrap up
KEY TAKEAWAYS
1: Jakub is an NLP expert who's been working on neural information retrieval since the early 90s using probabilistic machine learning methods. He founded Textkernel, a resume analysis company that used machine learning on unstructured data before switching to large neural models like LSTMs in 2015-2016 before he exited in 2019.
2: Jakub's new company, Zeta Alpha, aims to connect people with knowledge in general. They provide a platform for discovering new papers, organizing knowledge, and sharing notes with colleagues. The initial audience was deep learning researchers, but the platform is useful for anyone in a fragmented world of scientific discovery who wants to avoid reinventing the wheel.
3: Neural search is at the core of using AI for better decision-making, as it enables people to discover knowledge and information easier. In the last few years, there has been significant progress in neural search, with accuracies of relevance of search tripled in some domains.
4: Transformer-based language representations have bridged the gap of natural language search and limited keyword-based search achieving performances that are actually useful even when people don't know what they are searching for; especially important for researchers or decision-makers.
5: Neural search can embed a query in a vector space using large language models and connect it easily with passages of text or entities like people using algos like approximate nearest neighbor search. Personalized recommendations based on the user's reading history can help people make better sense of search results.
6: To improve user adoption in enterprise, the workflow needs to be turned around to bring the information to people where they usually sit in tools like email or Slack, rather than having them go to a search engine and type all kinds of queries. As an ML developer, it's important to consider the user workflow and interaction with the system.
7: Collaboration between machines and humans is necessary for users to trust the answers from the system. Transparency is crucial, and traceability to documents is important for research applications. Language models are evolving to incorporate retrieval components for efficient indexing and referencing back to original material.
8: Advanced academic work is often done by large tech companies, and open-source data sets are available for building foundation layers for applications. Achieving high accuracy for specific domains or languages may require closing performance gaps and using generative models to create synthetic training data.
9: Large language models can capture syntax very well, but interfacing with other data structures like graphs may be necessary in niche technical areas or where there are complicated semantic relationships. Some large language models can even translate intents/questions into actual programs (e.g., SQL queries or Python scripts) to facilitate that.
10: Building and maintaining a large knowledge representation may be too costly for most companies, and progress in machine learning is fast enough to make those investments unnecessary. There will be companies that handle publicly available knowledge as a foundational layer with abilities to fine tune to each client's needs.
11: It is exciting to see an ecosystem of products evolving that are reimagining knowledge work, representation of knowledge, and intellectual property. Changing the scientific publishing system is challenging since it is tied to other aspects of the economy, but there are companies in the AI and decentralized science space that are making a difference.
12: Knowledge is a shared and communal commodity, and any solution that doesn't involve grassroots and community-focused efforts will be incomplete. Any platform in this space needs to foster a sense of community and collaboration, augmented by AI and other technologies.
Видео Neural Search for Augmented Decision Making - Zeta Alpha - DRT S2E17 канала ML Explained - Aggregate Intellect - AI.SCIENCE
03:21 NLP in the 90's
04:19 Jakub's Resume analysis company
06:54 Selling TextKernel and starting Zeta Alpha
09:44 Initial assumption: deep learning researchers need a tool to keep track of advances in their field
11:27 Putting together a team to build a technical knowledge management product
15:31 Rise of large language models, augmented decision making, and enterprise search
20:07 Does finding documents for people (search) solve the problem that users have?
22:37 Reading papers is a nightmare (and how GPT3 et al can start to tackle this)
25:38 Knowledge management as a human-machine-interface problem
29:47 Differences between what academic and industrial audience would want
32:49 Multi-task nature of large language models to rescue?
35:49 Are language models alone enough?
39:22 What's down the pipe for Zeta Alpha?
41:12 Verdicts and wrap up
KEY TAKEAWAYS
1: Jakub is an NLP expert who's been working on neural information retrieval since the early 90s using probabilistic machine learning methods. He founded Textkernel, a resume analysis company that used machine learning on unstructured data before switching to large neural models like LSTMs in 2015-2016 before he exited in 2019.
2: Jakub's new company, Zeta Alpha, aims to connect people with knowledge in general. They provide a platform for discovering new papers, organizing knowledge, and sharing notes with colleagues. The initial audience was deep learning researchers, but the platform is useful for anyone in a fragmented world of scientific discovery who wants to avoid reinventing the wheel.
3: Neural search is at the core of using AI for better decision-making, as it enables people to discover knowledge and information easier. In the last few years, there has been significant progress in neural search, with accuracies of relevance of search tripled in some domains.
4: Transformer-based language representations have bridged the gap of natural language search and limited keyword-based search achieving performances that are actually useful even when people don't know what they are searching for; especially important for researchers or decision-makers.
5: Neural search can embed a query in a vector space using large language models and connect it easily with passages of text or entities like people using algos like approximate nearest neighbor search. Personalized recommendations based on the user's reading history can help people make better sense of search results.
6: To improve user adoption in enterprise, the workflow needs to be turned around to bring the information to people where they usually sit in tools like email or Slack, rather than having them go to a search engine and type all kinds of queries. As an ML developer, it's important to consider the user workflow and interaction with the system.
7: Collaboration between machines and humans is necessary for users to trust the answers from the system. Transparency is crucial, and traceability to documents is important for research applications. Language models are evolving to incorporate retrieval components for efficient indexing and referencing back to original material.
8: Advanced academic work is often done by large tech companies, and open-source data sets are available for building foundation layers for applications. Achieving high accuracy for specific domains or languages may require closing performance gaps and using generative models to create synthetic training data.
9: Large language models can capture syntax very well, but interfacing with other data structures like graphs may be necessary in niche technical areas or where there are complicated semantic relationships. Some large language models can even translate intents/questions into actual programs (e.g., SQL queries or Python scripts) to facilitate that.
10: Building and maintaining a large knowledge representation may be too costly for most companies, and progress in machine learning is fast enough to make those investments unnecessary. There will be companies that handle publicly available knowledge as a foundational layer with abilities to fine tune to each client's needs.
11: It is exciting to see an ecosystem of products evolving that are reimagining knowledge work, representation of knowledge, and intellectual property. Changing the scientific publishing system is challenging since it is tied to other aspects of the economy, but there are companies in the AI and decentralized science space that are making a difference.
12: Knowledge is a shared and communal commodity, and any solution that doesn't involve grassroots and community-focused efforts will be incomplete. Any platform in this space needs to foster a sense of community and collaboration, augmented by AI and other technologies.
Видео Neural Search for Augmented Decision Making - Zeta Alpha - DRT S2E17 канала ML Explained - Aggregate Intellect - AI.SCIENCE
Показать
Комментарии отсутствуют
Информация о видео
28 февраля 2023 г. 20:03:16
00:46:44
Другие видео канала
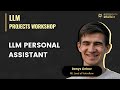
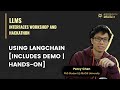
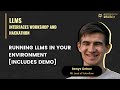
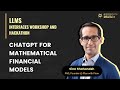
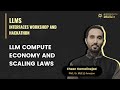
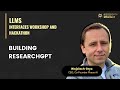
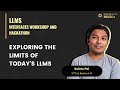

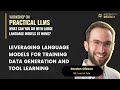
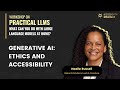
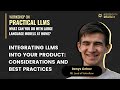
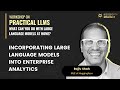
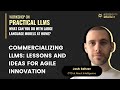
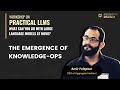
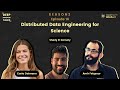
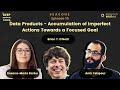
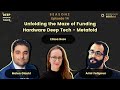
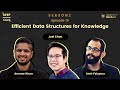
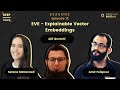
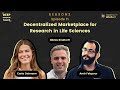