EVE - Explainable Vector Embeddings - DRT S2E12
00:53 Intro to the topic of explainable vector embeddings
2:30 GDPR as the initial motivation to work on explainable embeddings
3:26 How do you introduce semantics into decision making
4:40 How can strcutured knowledge (eg. taxonomies) interact with free text in analysis
7:00 Right to explanation, GDPR, ML, and revival of the phd work
10:41 Going beyond just a score for semantic relatedness without incuring huge computational cost
14:20 Semantic relatedness is not enough for explanation - the mode of relationship is also important (eg. can be inferred from links among wikipedia pages)
15:55 How does EVE work? TrustRank (sparse version of pagerank)
18:43 What is a concrete example of using EVE?
21:09 Three NLP tasks that the EVE paper examines (discrimination, clustering, ranking)
23:40 How are the embeddings (EVE) constructed? (comparison to Word2Vec)
29:20 EVE's performance; and where would you use strcutured data in conjunction with free text in language modelling?
34:29 How would EVE interact with newer models like Transformers?
39:03 EVE naturally works with graphical data, but would it apply to tabular data?
42:04 Amir's mandatory rant
43:55 Wrap up and verdicts
Key Takeaways:
1. The original problem being addressed was bringing semantics to decision-making, with the use of semantic web concepts and techniques to add context to unstructured text analysis in classification tasks.
2. The motivation for this approach came from the background in information retrieval and the desire to apply semantic web ideas to large volumes of free text data.
3. The development of knowledge in building semantic models during the PhD led to the application of this knowledge in addressing the right to explanation requirements of the GDPR in relation to Wikipedia.
4. The initial hypothesis was to use relatedness score to tackle a problem related to association between concepts, but this hypothesis did not pan out.
5. Introducing explainablity to semantic relatedness exactly requires managing the memory required to retain the entire graph structure of Wikipedia.
6. The main issue with the equation Atif had proposed during their PhD was that it did not explain why two concepts were related.
7. EVE uses a pre-determined data structure like Wikipedia graph to introduce explainabilty by leveraging sparse vectors reperesenting all possible entity categories
8. To determine the applicability of the new approach, three tasks were chosen to evaluate explainability, including discrimination test for topic modeling, clustering for grouping related items, and information retrieval for sorting out results related to a specific query.
9. It was discovered that even though the model may not always be accurate, the ability for the machine to explain its reasoning can lead to better improvement strategies
10. The use of embeddings in certain tasks can outperform other methods through the explanation provided.
11. Specifically, the use of explainable AI (XAI) in the context of information retrieval can be beneficial in identifying where the model is failing and fine-tuning large language models to tackle edge cases better.
12. The trend of over-parameterized models with a large number of parameters, using large amounts of data and computation, and ignoring the need for structure in input data, raises concerns about the usability of these models for average users and the assumption that the model will figure out the structure on its own.
Видео EVE - Explainable Vector Embeddings - DRT S2E12 канала ML Explained - Aggregate Intellect - AI.SCIENCE
2:30 GDPR as the initial motivation to work on explainable embeddings
3:26 How do you introduce semantics into decision making
4:40 How can strcutured knowledge (eg. taxonomies) interact with free text in analysis
7:00 Right to explanation, GDPR, ML, and revival of the phd work
10:41 Going beyond just a score for semantic relatedness without incuring huge computational cost
14:20 Semantic relatedness is not enough for explanation - the mode of relationship is also important (eg. can be inferred from links among wikipedia pages)
15:55 How does EVE work? TrustRank (sparse version of pagerank)
18:43 What is a concrete example of using EVE?
21:09 Three NLP tasks that the EVE paper examines (discrimination, clustering, ranking)
23:40 How are the embeddings (EVE) constructed? (comparison to Word2Vec)
29:20 EVE's performance; and where would you use strcutured data in conjunction with free text in language modelling?
34:29 How would EVE interact with newer models like Transformers?
39:03 EVE naturally works with graphical data, but would it apply to tabular data?
42:04 Amir's mandatory rant
43:55 Wrap up and verdicts
Key Takeaways:
1. The original problem being addressed was bringing semantics to decision-making, with the use of semantic web concepts and techniques to add context to unstructured text analysis in classification tasks.
2. The motivation for this approach came from the background in information retrieval and the desire to apply semantic web ideas to large volumes of free text data.
3. The development of knowledge in building semantic models during the PhD led to the application of this knowledge in addressing the right to explanation requirements of the GDPR in relation to Wikipedia.
4. The initial hypothesis was to use relatedness score to tackle a problem related to association between concepts, but this hypothesis did not pan out.
5. Introducing explainablity to semantic relatedness exactly requires managing the memory required to retain the entire graph structure of Wikipedia.
6. The main issue with the equation Atif had proposed during their PhD was that it did not explain why two concepts were related.
7. EVE uses a pre-determined data structure like Wikipedia graph to introduce explainabilty by leveraging sparse vectors reperesenting all possible entity categories
8. To determine the applicability of the new approach, three tasks were chosen to evaluate explainability, including discrimination test for topic modeling, clustering for grouping related items, and information retrieval for sorting out results related to a specific query.
9. It was discovered that even though the model may not always be accurate, the ability for the machine to explain its reasoning can lead to better improvement strategies
10. The use of embeddings in certain tasks can outperform other methods through the explanation provided.
11. Specifically, the use of explainable AI (XAI) in the context of information retrieval can be beneficial in identifying where the model is failing and fine-tuning large language models to tackle edge cases better.
12. The trend of over-parameterized models with a large number of parameters, using large amounts of data and computation, and ignoring the need for structure in input data, raises concerns about the usability of these models for average users and the assumption that the model will figure out the structure on its own.
Видео EVE - Explainable Vector Embeddings - DRT S2E12 канала ML Explained - Aggregate Intellect - AI.SCIENCE
Показать
Комментарии отсутствуют
Информация о видео
25 января 2023 г. 18:22:28
00:50:30
Другие видео канала
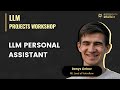
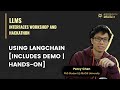
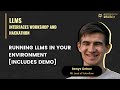
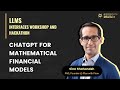
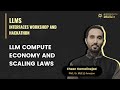
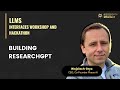
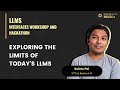

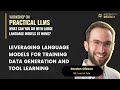
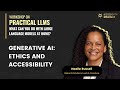
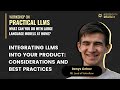
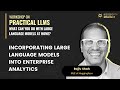
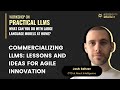
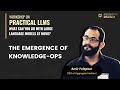
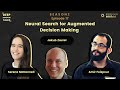
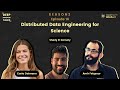
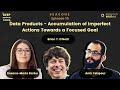
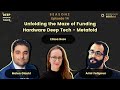
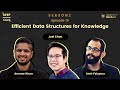
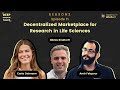