Anomaly Detection: Algorithms, Explanations, Applications
Anomaly detection is important for data cleaning, cybersecurity, and robust AI systems. This talk will review recent work in our group on (a) benchmarking existing algorithms, (b) developing a theoretical understanding of their behavior, (c) explaining anomaly "alarms" to a data analyst, and (d) interactively re-ranking candidate anomalies in response to analyst feedback. Then the talk will describe two applications: (a) detecting and diagnosing sensor failures in weather networks and (b) open category detection in supervised learning.
See more at https://www.microsoft.com/en-us/research/video/anomaly-detection-algorithms-explanations-applications/
Видео Anomaly Detection: Algorithms, Explanations, Applications канала Microsoft Research
See more at https://www.microsoft.com/en-us/research/video/anomaly-detection-algorithms-explanations-applications/
Видео Anomaly Detection: Algorithms, Explanations, Applications канала Microsoft Research
Показать
Комментарии отсутствуют
Информация о видео
Другие видео канала
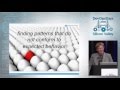
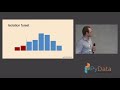
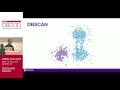
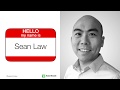
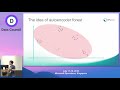
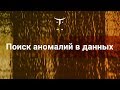
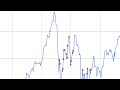
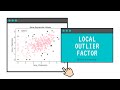
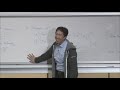
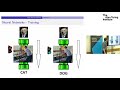
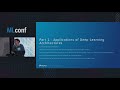
![Lecture 15.1 — Anomaly Detection Problem | Motivation — [ Machine Learning | Andrew Ng ]](https://i.ytimg.com/vi/086OcT-5DYI/default.jpg)
![Lecture 15.2 — Anomaly Detection | Gaussian Distribution — [ Machine Learning | Andrew Ng ]](https://i.ytimg.com/vi/mh6rAYA0e7Q/default.jpg)
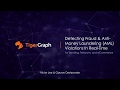
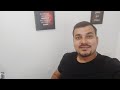
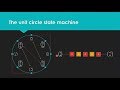
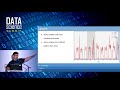
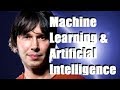
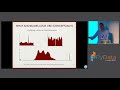
