Explaining Machine Learning Classifiers through Diverse Counterfactual Explanations
Explaining Machine Learning Classifiers through Diverse Counterfactual Explanations
R. Mothilal; A. Sharma; C. Tan
Research Track - FAT*2020, Barcelona, January 27th to 30th, 2020
https://dl.acm.org/doi/abs/10.1145/3351095.3372850
Видео Explaining Machine Learning Classifiers through Diverse Counterfactual Explanations канала ACM FAccT Conference
R. Mothilal; A. Sharma; C. Tan
Research Track - FAT*2020, Barcelona, January 27th to 30th, 2020
https://dl.acm.org/doi/abs/10.1145/3351095.3372850
Видео Explaining Machine Learning Classifiers through Diverse Counterfactual Explanations канала ACM FAccT Conference
Показать
Комментарии отсутствуют
Информация о видео
Другие видео канала


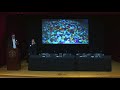
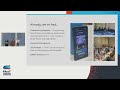
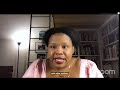
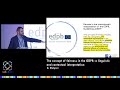
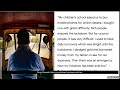

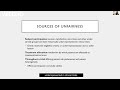
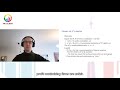
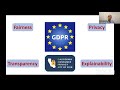
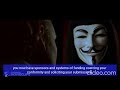
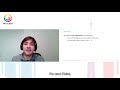
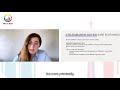
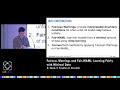
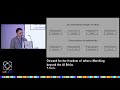
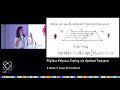
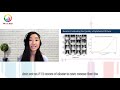
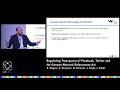
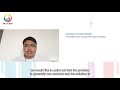
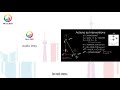