Organize, Track, and Evaluate ML Training Runs With Amazon SageMaker Experiments
Training an ML model typically entails many iterations to isolate and measure the impact of multiple variables. In this video, learn how Amazon SagMaker Experiments can help you and track these iterations within the visual interface of SageMaker Studio.
Learn more about Amazon SageMaker at https://go.aws/3fZ2QS1
Subscribe:
More AWS videos http://bit.ly/2O3zS75
More AWS events videos http://bit.ly/316g9t4
#AWS #MachineLearning
Видео Organize, Track, and Evaluate ML Training Runs With Amazon SageMaker Experiments канала Amazon Web Services
Learn more about Amazon SageMaker at https://go.aws/3fZ2QS1
Subscribe:
More AWS videos http://bit.ly/2O3zS75
More AWS events videos http://bit.ly/316g9t4
#AWS #MachineLearning
Видео Organize, Track, and Evaluate ML Training Runs With Amazon SageMaker Experiments канала Amazon Web Services
Показать
Комментарии отсутствуют
Информация о видео
Другие видео канала
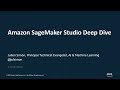
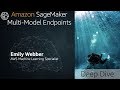

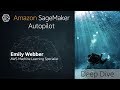

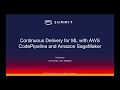
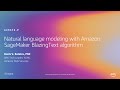
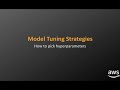
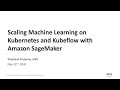
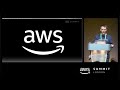
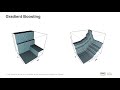
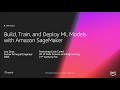

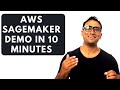

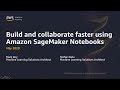
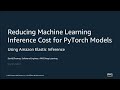

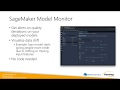