Machine Learning Explainability & Bias Detection with Watson OpenScale
So you've built a model.
It's deployed.
Now what?
How do you know if it's performing well?
How do you keep track of predictions?
Better yet, how do you explain them?
In this video, you'll learn how to do exactly that using Watson OpenScale. in 20ish minutes I'll walk you through how to leverage Watson OpenScale for machine learning explainability, debiasing and drift detection.
In this video you'll learn how to:
1. Setting up Watson OpenScale
2. Viewing Model Performance Metrics like Accuracy, AUC, Precision
3. Debiasing Machine Learning Predictions
4. Explaining and Interpret Machine Learning Model Predictions
Links Mentioned
IBM Cloud Register: https://cloud.ibm.com/registration
Watson OpenScale: https://cloud.ibm.com/catalog/services/watson-openscale
Chapters
0:00 - Start
0:27 - Explainer
1:26 - How it Works
2:03 - Setup Watson OpenScale
6:21 - Evaluating Model Performance
12:30 - Mitigating and Detecting Bias in ML Models
14:39 - Explaining and Interpreting Predictions
17:09 - What-If Scenario Modelling using OpenScale
19:23 - Tracking Model Quality
20:19 - Evaluating Model and Data Drift
22:47 - Wrap Up
Oh, and don't forget to connect with me!
LinkedIn: https://bit.ly/324Epgo
Facebook: https://bit.ly/3mB1sZD
GitHub: https://bit.ly/3mDJllD
Patreon: https://bit.ly/2OCn3UW
Join the Discussion on Discord: https://bit.ly/3dQiZsV
Happy coding!
Nick
P.s. Let me know how you go and drop a comment if you need a hand!
Видео Machine Learning Explainability & Bias Detection with Watson OpenScale канала Nicholas Renotte
It's deployed.
Now what?
How do you know if it's performing well?
How do you keep track of predictions?
Better yet, how do you explain them?
In this video, you'll learn how to do exactly that using Watson OpenScale. in 20ish minutes I'll walk you through how to leverage Watson OpenScale for machine learning explainability, debiasing and drift detection.
In this video you'll learn how to:
1. Setting up Watson OpenScale
2. Viewing Model Performance Metrics like Accuracy, AUC, Precision
3. Debiasing Machine Learning Predictions
4. Explaining and Interpret Machine Learning Model Predictions
Links Mentioned
IBM Cloud Register: https://cloud.ibm.com/registration
Watson OpenScale: https://cloud.ibm.com/catalog/services/watson-openscale
Chapters
0:00 - Start
0:27 - Explainer
1:26 - How it Works
2:03 - Setup Watson OpenScale
6:21 - Evaluating Model Performance
12:30 - Mitigating and Detecting Bias in ML Models
14:39 - Explaining and Interpreting Predictions
17:09 - What-If Scenario Modelling using OpenScale
19:23 - Tracking Model Quality
20:19 - Evaluating Model and Data Drift
22:47 - Wrap Up
Oh, and don't forget to connect with me!
LinkedIn: https://bit.ly/324Epgo
Facebook: https://bit.ly/3mB1sZD
GitHub: https://bit.ly/3mDJllD
Patreon: https://bit.ly/2OCn3UW
Join the Discussion on Discord: https://bit.ly/3dQiZsV
Happy coding!
Nick
P.s. Let me know how you go and drop a comment if you need a hand!
Видео Machine Learning Explainability & Bias Detection with Watson OpenScale канала Nicholas Renotte
Показать
Комментарии отсутствуют
Информация о видео
Другие видео канала
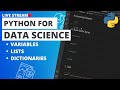
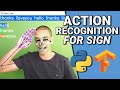
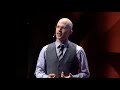
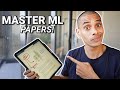
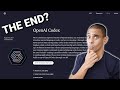
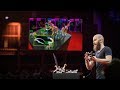
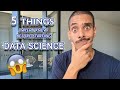
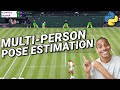
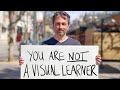
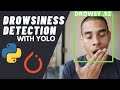
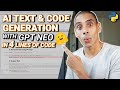
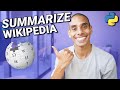
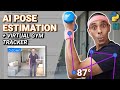
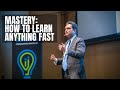
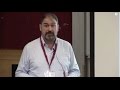
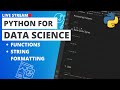
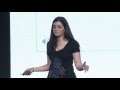

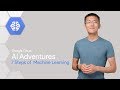
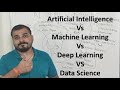