Signal and Image Classification - Stephane Mallat Technion lecture
Signal and Image Classification - lecture by Stephane Mallat of École Normale Supérieure, Paris at the TCE conference 2014 at Technion-Israel Institute of Technology
Signals and images are very high dimensional with a considerable variability. Their classification is facing a curse of dimensionality, which potentially requires a number of training examples which grows exponentially with the signal dimension. To avoid this wall, we need to find structures allowing to reduce the problem dimensionality. We shall first review the main techniques to do so, and then focus on deep neural networks which currently provide state of the art results in most areas of signal and image classification. Dimensionality reduction can be achieved by learning invariants relatively to the action of unknown groups. For complex problems, we must first increase the representation dimensionality in order to separate variables governing the classification problem. Wavelet transforms and multirate filter banks play an important role by separating variabilities at different scales. Invariants are then computed with supervised linear operators. They reduce the signal dimensionality, depending upon the classification task. Multiple applications will be shown on audio and medical signals, as well as images.
Видео Signal and Image Classification - Stephane Mallat Technion lecture канала Technion
Signals and images are very high dimensional with a considerable variability. Their classification is facing a curse of dimensionality, which potentially requires a number of training examples which grows exponentially with the signal dimension. To avoid this wall, we need to find structures allowing to reduce the problem dimensionality. We shall first review the main techniques to do so, and then focus on deep neural networks which currently provide state of the art results in most areas of signal and image classification. Dimensionality reduction can be achieved by learning invariants relatively to the action of unknown groups. For complex problems, we must first increase the representation dimensionality in order to separate variables governing the classification problem. Wavelet transforms and multirate filter banks play an important role by separating variabilities at different scales. Invariants are then computed with supervised linear operators. They reduce the signal dimensionality, depending upon the classification task. Multiple applications will be shown on audio and medical signals, as well as images.
Видео Signal and Image Classification - Stephane Mallat Technion lecture канала Technion
Показать
Комментарии отсутствуют
Информация о видео
Другие видео канала
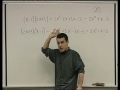
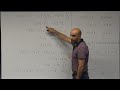

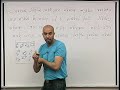
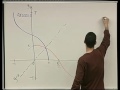
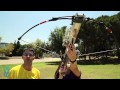
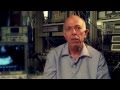
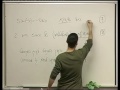
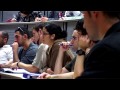
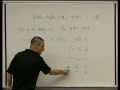
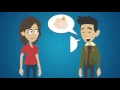
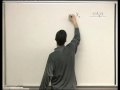
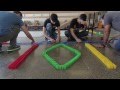
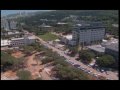
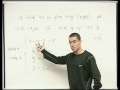

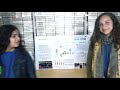
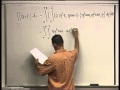
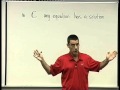
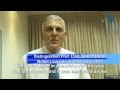
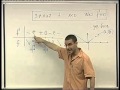