Virginia Smith - A General Framework for Communication-Efficient Distributed... - MLconf SF 2016
Presentation slides: http://www.slideshare.net/SessionsEvents/virginia-smith-researcher-uc-berkeley-at-mlconf-sf-2016
A General Framework for Communication-Efficient Distributed Optimization: Communication remains the most significant bottleneck in the performance of distributed optimization algorithms for large-scale machine learning. In light of this, we propose a general framework, CoCoA, that uses local computation in a primal-dual setting to dramatically reduce the amount of necessary communication. Our framework enjoys strong convergence guarantees and exhibits state-of-the-art empirical performance in the distributed setting. We demonstrate this performance with extensive experiments in Apache Spark, achieving speedups of up to 50x compared to leading distributed methods on common machine learning objectives.
Видео Virginia Smith - A General Framework for Communication-Efficient Distributed... - MLconf SF 2016 канала MLconf
A General Framework for Communication-Efficient Distributed Optimization: Communication remains the most significant bottleneck in the performance of distributed optimization algorithms for large-scale machine learning. In light of this, we propose a general framework, CoCoA, that uses local computation in a primal-dual setting to dramatically reduce the amount of necessary communication. Our framework enjoys strong convergence guarantees and exhibits state-of-the-art empirical performance in the distributed setting. We demonstrate this performance with extensive experiments in Apache Spark, achieving speedups of up to 50x compared to leading distributed methods on common machine learning objectives.
Видео Virginia Smith - A General Framework for Communication-Efficient Distributed... - MLconf SF 2016 канала MLconf
Показать
Комментарии отсутствуют
Информация о видео
Другие видео канала

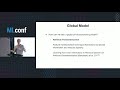
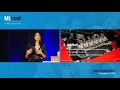
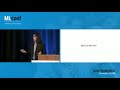


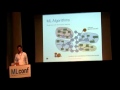
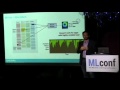
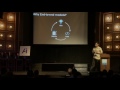
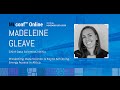

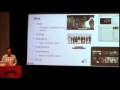
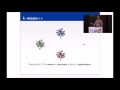

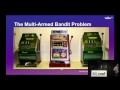
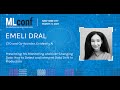

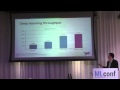
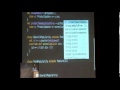
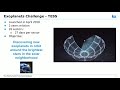