Tin Nguyen: "Sensitivity of MCMC-based analyses to small-data removal"
Talk Title: Sensitivity of MCMC-based analyses to small-data removal
Thesis Committee: Tamara Broderick, Ashia Wilson, and Stefanie Jegelka
Talk Abstract: If the conclusion of a data analysis is sensitive to dropping very few data points, that conclusion might hinge on the particular data at hand rather than representing a more broadly applicable truth. How could we check whether this sensitivity holds? One idea is to consider every small subset of data, drop it from the dataset, and re-run our analysis. But running MCMC to approximate a Bayesian posterior is already very expensive; running multiple times is prohibitive, and the number of re-runs needed here is combinatorially large. Recent work proposes a fast and accurate approximation to find the worst-case dropped data subset, but that work was developed for problems based on estimating equations --- and does not directly handle Bayesian posterior approximations using MCMC. We make two principal contributions in the present work. We adapt the existing data-dropping approximation to estimators computed via MCMC. Observing that Monte Carlo errors induce variability in the approximation, we use a variant of the bootstrap to quantify this uncertainty. We demonstrate how to use our approximation in practice to determine whether there is non-robustness in a problem. Empirically, our method is accurate in simple models, such as linear regression. In models with complicated structure, such as hierarchical models, the performance of our method is mixed.
Видео Tin Nguyen: "Sensitivity of MCMC-based analyses to small-data removal" канала Tamara Broderick
Thesis Committee: Tamara Broderick, Ashia Wilson, and Stefanie Jegelka
Talk Abstract: If the conclusion of a data analysis is sensitive to dropping very few data points, that conclusion might hinge on the particular data at hand rather than representing a more broadly applicable truth. How could we check whether this sensitivity holds? One idea is to consider every small subset of data, drop it from the dataset, and re-run our analysis. But running MCMC to approximate a Bayesian posterior is already very expensive; running multiple times is prohibitive, and the number of re-runs needed here is combinatorially large. Recent work proposes a fast and accurate approximation to find the worst-case dropped data subset, but that work was developed for problems based on estimating equations --- and does not directly handle Bayesian posterior approximations using MCMC. We make two principal contributions in the present work. We adapt the existing data-dropping approximation to estimators computed via MCMC. Observing that Monte Carlo errors induce variability in the approximation, we use a variant of the bootstrap to quantify this uncertainty. We demonstrate how to use our approximation in practice to determine whether there is non-robustness in a problem. Empirically, our method is accurate in simple models, such as linear regression. In models with complicated structure, such as hierarchical models, the performance of our method is mixed.
Видео Tin Nguyen: "Sensitivity of MCMC-based analyses to small-data removal" канала Tamara Broderick
Показать
Комментарии отсутствуют
Информация о видео
Другие видео канала
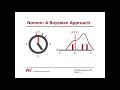
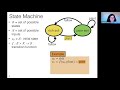

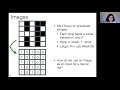
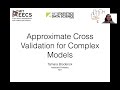
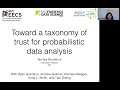
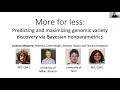
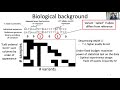
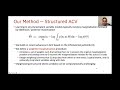
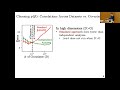
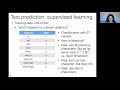

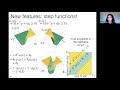
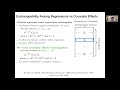
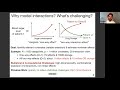
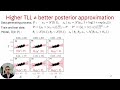
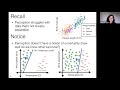

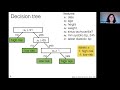
