Miles Stoudenmire: Introduction to Tensor Networks for Machine Learning.
Miles Stoudenmire (Flatiron Institute)
Talk given at CMAC2020
https://research.kent.ac.uk/pqm/condensed-matter-physics-in-the-cities-2020/schedule/
Abstract:
Tensor networks are a tool to store and compute with tensors having many indices, which would otherwise be exponentially costly. While in physics the prototypical use of tensor networks is for approximating many-body wavefunctions, it has been appreciated over the last decade that tensor networks such as matrix product states (MPS) are much more general tools. One emerging application of tensor networks is for machine learning, where they can be used for a variety of tasks such as supervised learning, generative modeling, and compression of neural network parameters. Compared to other machine learning approaches, tensor networks offer advantages such as scalability, more sophisticated training algorithms, and theoretical clarity. After reviewing the basics of tensor networks, I will introduce the setting of machine learning and explain how tensor networks can be used for machine learning tasks, showing exemplary results for both classical (non-physics) applications and physics applications.
Видео Miles Stoudenmire: Introduction to Tensor Networks for Machine Learning. канала Condensed Matter Physics In the City
Talk given at CMAC2020
https://research.kent.ac.uk/pqm/condensed-matter-physics-in-the-cities-2020/schedule/
Abstract:
Tensor networks are a tool to store and compute with tensors having many indices, which would otherwise be exponentially costly. While in physics the prototypical use of tensor networks is for approximating many-body wavefunctions, it has been appreciated over the last decade that tensor networks such as matrix product states (MPS) are much more general tools. One emerging application of tensor networks is for machine learning, where they can be used for a variety of tasks such as supervised learning, generative modeling, and compression of neural network parameters. Compared to other machine learning approaches, tensor networks offer advantages such as scalability, more sophisticated training algorithms, and theoretical clarity. After reviewing the basics of tensor networks, I will introduce the setting of machine learning and explain how tensor networks can be used for machine learning tasks, showing exemplary results for both classical (non-physics) applications and physics applications.
Видео Miles Stoudenmire: Introduction to Tensor Networks for Machine Learning. канала Condensed Matter Physics In the City
Показать
Комментарии отсутствуют
Информация о видео
18 июня 2020 г. 6:47:36
01:14:01
Другие видео канала
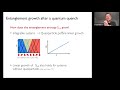
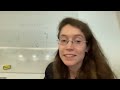
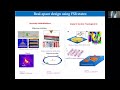
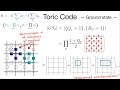
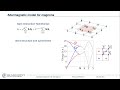
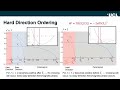
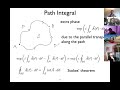
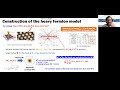



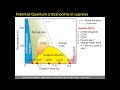
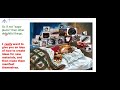
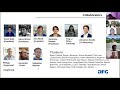
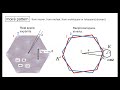

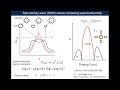
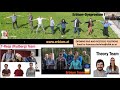
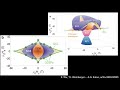
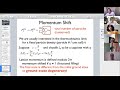