[POPL'23] Top-Down Synthesis for Library Learning
[POPL'23] Top-Down Synthesis for Library Learning
Matt Bowers, Theo X. Olausson, Lionel Wong, Gabriel Grand, Joshua B. Tenenbaum, Kevin Ellis, Armando Solar-Lezama
This paper introduces \textit{corpus-guided top-down synthesis} as a mechanism for synthesizing library functions that capture common functionality from a corpus of programs in a domain specific language (DSL). The algorithm builds abstractions directly from initial DSL primitives, using syntactic pattern matching of intermediate abstractions to intelligently prune the search space and guide the algorithm towards abstractions that maximally capture shared structures in the corpus. We present an implementation of the approach in a tool called \textsc{Stitch} and evaluate it against the state-of-the-art deductive library learning algorithm from DreamCoder. Our evaluation shows that \textsc{Stitch} is 3-4 orders of magnitude faster and uses 2 orders of magnitude less memory while maintaining comparable or better library quality (as measured by compressivity). We also demonstrate \textsc{Stitch}'s scalability on corpora containing hundreds of complex programs that are intractable with prior deductive approaches and show empirically that it is robust to terminating the search procedure early—further allowing it to scale to challenging datasets by means of early stopping.
Видео [POPL'23] Top-Down Synthesis for Library Learning канала ACM SIGPLAN
Matt Bowers, Theo X. Olausson, Lionel Wong, Gabriel Grand, Joshua B. Tenenbaum, Kevin Ellis, Armando Solar-Lezama
This paper introduces \textit{corpus-guided top-down synthesis} as a mechanism for synthesizing library functions that capture common functionality from a corpus of programs in a domain specific language (DSL). The algorithm builds abstractions directly from initial DSL primitives, using syntactic pattern matching of intermediate abstractions to intelligently prune the search space and guide the algorithm towards abstractions that maximally capture shared structures in the corpus. We present an implementation of the approach in a tool called \textsc{Stitch} and evaluate it against the state-of-the-art deductive library learning algorithm from DreamCoder. Our evaluation shows that \textsc{Stitch} is 3-4 orders of magnitude faster and uses 2 orders of magnitude less memory while maintaining comparable or better library quality (as measured by compressivity). We also demonstrate \textsc{Stitch}'s scalability on corpora containing hundreds of complex programs that are intractable with prior deductive approaches and show empirically that it is robust to terminating the search procedure early—further allowing it to scale to challenging datasets by means of early stopping.
Видео [POPL'23] Top-Down Synthesis for Library Learning канала ACM SIGPLAN
Показать
Комментарии отсутствуют
Информация о видео
Другие видео канала
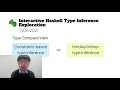
![[OCaml'22] Efficient “out of heap” pointers for multicore OCaml](https://i.ytimg.com/vi/eIQf1bRl8_k/default.jpg)
![[PriSC'22] Composing Secure Compilers](https://i.ytimg.com/vi/OtR_6Paehms/default.jpg)

![[SLE] Property-Based Testing: Climbing the Stairway to Verification](https://i.ytimg.com/vi/QGOnUm5HBmo/default.jpg)
![[ML'22] Efficient and Scalable Parallel Functional Programming Through Disentanglement](https://i.ytimg.com/vi/IMPF-Vz1YqI/default.jpg)
![[CPP'23] CompCert: a journey through the landscape of mechanized semantics for verified co...](https://i.ytimg.com/vi/9KhagyiGQ_A/default.jpg)
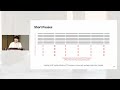
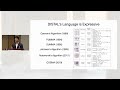
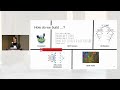
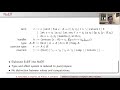
![[ICFP'22] Safe Couplings: Coupled Refinement Types](https://i.ytimg.com/vi/4LV8a8cz_w0/default.jpg)
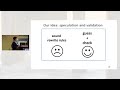
![[ICFP'22] Beyond Relooper: Recursive Translation of Unstructured Control Flow to Structu…](https://i.ytimg.com/vi/qAeEWKr9wfU/default.jpg)
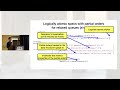
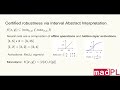
![[POPL'22] Layered and Object-Based Game Semantics](https://i.ytimg.com/vi/OK2RnP2hL6g/default.jpg)
![[POPL'22] Verified Tensor-Program Optimization Via High-Level Scheduling Rewrites](https://i.ytimg.com/vi/f2NEU1ENt6A/default.jpg)
![[VMCAI'22] Making PROGRESS in Property Directed Reachability](https://i.ytimg.com/vi/IexW7nvkwwM/default.jpg)
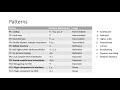
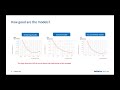