Building ML/Data Platform on Top of Kubernetes // Julien Bisconti // MLOps Coffee Sessions #86
MLOps Coffee Sessions #86 with Julien Bisconti, Building ML/Data Platform on Top of Kubernetes.
// Abstract
When building a platform, a good start would be to define the goals and features of that platform, knowing it will evolve. Kubernetes is established as the de facto standard for scalable platforms but it is not a fully-fledged data platform.
Do ML engineers have to learn and use Kubernetes directly?
They probably shouldn't. So it is up to the data engineering team to provide the tools and abstraction necessary to allow ML engineers to do their work.
The time, effort, and knowledge it takes to build a data platform is already quite an achievement. When it is built, one has to maintain it, monitor it, train people to on-call rotation, implement escalation policies and disaster recovery, optimize for usage and costs, secure it and build a whole ecosystem of tools around it (front-end, CLI, dashboards).
That cost might be too high and time-consuming for some companies to consider building their own ML platform as opposed to cloud offering alternatives. Note that cloud offerings still require some of those points but most of the work is already done.
// Bio
Julien is a software engineer turned Site Reliability Engineer. He is a Google developer expert, certified Data Engineer on Google Cloud and Kubernetes Administrator, mentor for Woman Developer Academy and Google For Startups program. He is working on building and maintaining data/ML platform.
// Related Links
https://portal.superwise.ai/
Crossing the River by Feeling the Stones • Simon Wardley • GOTO 2018: https://www.youtube.com/watch?v=2IW9L1uNMCs
--------------- ✌️Connect With Us ✌️ -------------
Join our slack community: https://go.mlops.community/slack
Follow us on Twitter: @mlopscommunity
Sign up for the next meetup: https://go.mlops.community/register
Catch all episodes, blogs, newsletter and more: https://mlops.community/
Connect with Demetrios on LinkedIn: https://www.linkedin.com/in/dpbrinkm/
Connect with Vishnu on LinkedIn: https://www.linkedin.com/in/vrachakonda/
Connect with Julien on LinkedIn: https://www.linkedin.com/in/julienbisconti/
Timestamps:
[00:00] French intro by Julien
[00:32] Introduction to Julien Bisconti
[03:35] Arriving at the non-technical side process of MLOps
[06:06] Envious of people with technological problems
[07:27] People problem bandwidth conversation
[11:04] Atomic decision making
[14:20] Advice to developers either to buy or build in their career potential
[18:23] Jobs board - https://mlops.pallet.xyz/jobs
[21:28] Chaos engineering
[26:33] Role of chaos engineering in building production machine learning systems
[32:59] Core challenge of MLOps
[37:04] Standardization on an industry level
[40:30] Reconciliation of trade-offs using Vertex and Sagemaker
[45:21] Crossing the River by Feeling the Stones talk by Simon Wardley
[47:22] Wrap up
Видео Building ML/Data Platform on Top of Kubernetes // Julien Bisconti // MLOps Coffee Sessions #86 канала MLOps.community
// Abstract
When building a platform, a good start would be to define the goals and features of that platform, knowing it will evolve. Kubernetes is established as the de facto standard for scalable platforms but it is not a fully-fledged data platform.
Do ML engineers have to learn and use Kubernetes directly?
They probably shouldn't. So it is up to the data engineering team to provide the tools and abstraction necessary to allow ML engineers to do their work.
The time, effort, and knowledge it takes to build a data platform is already quite an achievement. When it is built, one has to maintain it, monitor it, train people to on-call rotation, implement escalation policies and disaster recovery, optimize for usage and costs, secure it and build a whole ecosystem of tools around it (front-end, CLI, dashboards).
That cost might be too high and time-consuming for some companies to consider building their own ML platform as opposed to cloud offering alternatives. Note that cloud offerings still require some of those points but most of the work is already done.
// Bio
Julien is a software engineer turned Site Reliability Engineer. He is a Google developer expert, certified Data Engineer on Google Cloud and Kubernetes Administrator, mentor for Woman Developer Academy and Google For Startups program. He is working on building and maintaining data/ML platform.
// Related Links
https://portal.superwise.ai/
Crossing the River by Feeling the Stones • Simon Wardley • GOTO 2018: https://www.youtube.com/watch?v=2IW9L1uNMCs
--------------- ✌️Connect With Us ✌️ -------------
Join our slack community: https://go.mlops.community/slack
Follow us on Twitter: @mlopscommunity
Sign up for the next meetup: https://go.mlops.community/register
Catch all episodes, blogs, newsletter and more: https://mlops.community/
Connect with Demetrios on LinkedIn: https://www.linkedin.com/in/dpbrinkm/
Connect with Vishnu on LinkedIn: https://www.linkedin.com/in/vrachakonda/
Connect with Julien on LinkedIn: https://www.linkedin.com/in/julienbisconti/
Timestamps:
[00:00] French intro by Julien
[00:32] Introduction to Julien Bisconti
[03:35] Arriving at the non-technical side process of MLOps
[06:06] Envious of people with technological problems
[07:27] People problem bandwidth conversation
[11:04] Atomic decision making
[14:20] Advice to developers either to buy or build in their career potential
[18:23] Jobs board - https://mlops.pallet.xyz/jobs
[21:28] Chaos engineering
[26:33] Role of chaos engineering in building production machine learning systems
[32:59] Core challenge of MLOps
[37:04] Standardization on an industry level
[40:30] Reconciliation of trade-offs using Vertex and Sagemaker
[45:21] Crossing the River by Feeling the Stones talk by Simon Wardley
[47:22] Wrap up
Видео Building ML/Data Platform on Top of Kubernetes // Julien Bisconti // MLOps Coffee Sessions #86 канала MLOps.community
Показать
Комментарии отсутствуют
Информация о видео
Другие видео канала
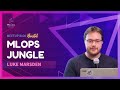
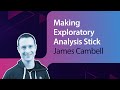

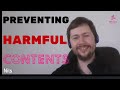
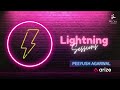

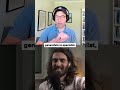
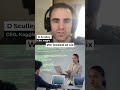

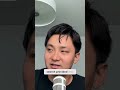
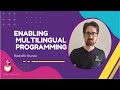
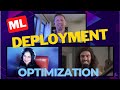
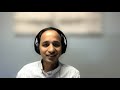

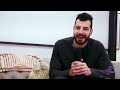
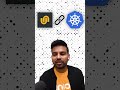
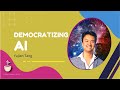

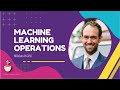
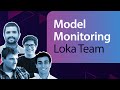