tinyML Research Symposium: TinyRCE Forward Learning under Tiny Constraints
https://www.tinyml.org/event/research-symposium-2023/#schedule
TinyRCE Forward Learning under Tiny Constraints
Danilo PAU, Technical Director, IEEE and ST Fellow, STMicroelectronics
The challenge posed by on-tiny-devices learning targeting ultra-low power embedded devices has recently attracted several machine learning researchers. A typical model learning session requires real time streams of data produced by a plethora of heterogeneous sensors. In such a context, this paper proposes a forward-only learning approach based on a hyper-spherical classifier with the aim to be deployed on microcontrollers and sensors. This algorithm drives the learning process using streams of data associated to categories that shall be learnt by the proposed method. The classifier is fed with features extracted by a convolutional neural network belonging to the extreme learning machine class. The weights of the topology were randomly initialized. Alternatively, it could be trained offline with backpropagation. Its weights then are stored in a tiny read only memory of 76.45 KiBytes. The classifier itself required up to 40.2 KiBytes of RAM to perform a complete on-device learning workload in 0.216sec running on a MCU clocked at 480MHz. A forget mechanism has been introduced to discard redundant neurons, placed into the classifier’s hidden layer, if they would become useless over time. This classifier has been evaluated with a new interleaved training and testing protocol to mimic a forward on-tiny-device learning workload. It has been tested with openly available datasets shaping human activity monitoring (PAMAP2, SHL) and ball-bearing anomaly detection (CWRU) case studies. Experiments have shown that the proposed TinyRCE classifier performed competitively against to a supervised convolutional topology followed by a SoftMax classifier trained with backpropagation procedure on all three datasets.
Видео tinyML Research Symposium: TinyRCE Forward Learning under Tiny Constraints канала The tinyML Foundation
TinyRCE Forward Learning under Tiny Constraints
Danilo PAU, Technical Director, IEEE and ST Fellow, STMicroelectronics
The challenge posed by on-tiny-devices learning targeting ultra-low power embedded devices has recently attracted several machine learning researchers. A typical model learning session requires real time streams of data produced by a plethora of heterogeneous sensors. In such a context, this paper proposes a forward-only learning approach based on a hyper-spherical classifier with the aim to be deployed on microcontrollers and sensors. This algorithm drives the learning process using streams of data associated to categories that shall be learnt by the proposed method. The classifier is fed with features extracted by a convolutional neural network belonging to the extreme learning machine class. The weights of the topology were randomly initialized. Alternatively, it could be trained offline with backpropagation. Its weights then are stored in a tiny read only memory of 76.45 KiBytes. The classifier itself required up to 40.2 KiBytes of RAM to perform a complete on-device learning workload in 0.216sec running on a MCU clocked at 480MHz. A forget mechanism has been introduced to discard redundant neurons, placed into the classifier’s hidden layer, if they would become useless over time. This classifier has been evaluated with a new interleaved training and testing protocol to mimic a forward on-tiny-device learning workload. It has been tested with openly available datasets shaping human activity monitoring (PAMAP2, SHL) and ball-bearing anomaly detection (CWRU) case studies. Experiments have shown that the proposed TinyRCE classifier performed competitively against to a supervised convolutional topology followed by a SoftMax classifier trained with backpropagation procedure on all three datasets.
Видео tinyML Research Symposium: TinyRCE Forward Learning under Tiny Constraints канала The tinyML Foundation
Показать
Комментарии отсутствуют
Информация о видео
Другие видео канала
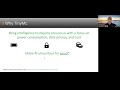
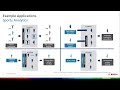
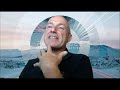
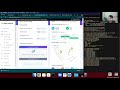
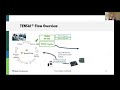
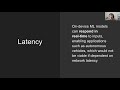
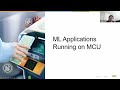
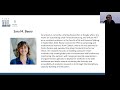

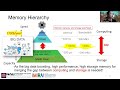
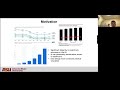
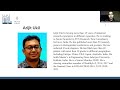
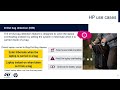
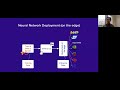
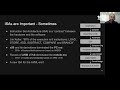
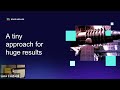
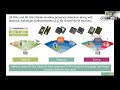
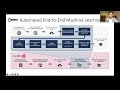
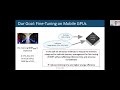
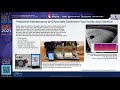
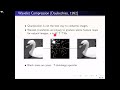