Robust Superpixel-Guided Attentional Adversarial Attack
Authors: Xiaoyi Dong, Jiangfan Han, Dongdong Chen, Jiayang Liu, Huanyu Bian, Zehua Ma, Hongsheng Li, Xiaogang Wang, Weiming Zhang, Nenghai Yu
Deep Neural Networks are vulnerable to adversarial samples, which can fool classifiers by adding small perturbations onto the original image. Since the pioneering optimization-based adversarial attack method, many following methods have been proposed in the past several years. However most of these methods add perturbations in a \textit{``pixel-wise"} and \textit{``global"} way. Firstly, because of the contradiction between the local smoothness of natural images and the noisy property of these adversarial perturbations, this \textit{``pixel-wise"} way makes these methods not robust to image processing based defense methods and steganalysis based detection methods. Secondly, we find adding perturbations to the background is less useful than to the salient object, thus the \textit{``global"} way is also not optimal. Based on these two considerations, we propose the first robust superpixel-guided attentional adversarial attack method. Specifically, the adversarial perturbations are only added to the salient regions and guaranteed to be same within each superpixel. Through extensive experiments, we demonstrate our method can preserve the attack ability even in this highly constrained modification space. More importantly, compared to existing methods, it is significantly more robust to image processing based defense and steganalysis based detection.
Видео Robust Superpixel-Guided Attentional Adversarial Attack канала ComputerVisionFoundation Videos
Deep Neural Networks are vulnerable to adversarial samples, which can fool classifiers by adding small perturbations onto the original image. Since the pioneering optimization-based adversarial attack method, many following methods have been proposed in the past several years. However most of these methods add perturbations in a \textit{``pixel-wise"} and \textit{``global"} way. Firstly, because of the contradiction between the local smoothness of natural images and the noisy property of these adversarial perturbations, this \textit{``pixel-wise"} way makes these methods not robust to image processing based defense methods and steganalysis based detection methods. Secondly, we find adding perturbations to the background is less useful than to the salient object, thus the \textit{``global"} way is also not optimal. Based on these two considerations, we propose the first robust superpixel-guided attentional adversarial attack method. Specifically, the adversarial perturbations are only added to the salient regions and guaranteed to be same within each superpixel. Through extensive experiments, we demonstrate our method can preserve the attack ability even in this highly constrained modification space. More importantly, compared to existing methods, it is significantly more robust to image processing based defense and steganalysis based detection.
Видео Robust Superpixel-Guided Attentional Adversarial Attack канала ComputerVisionFoundation Videos
Показать
Комментарии отсутствуют
Информация о видео
14 мая 2021 г. 20:26:51
00:01:01
Другие видео канала
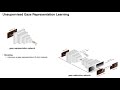
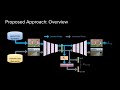
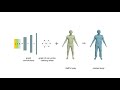
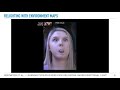
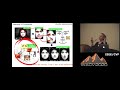
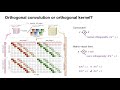
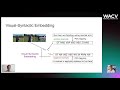

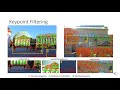

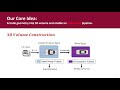
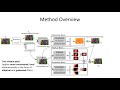
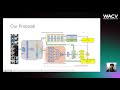
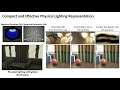
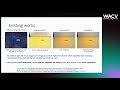
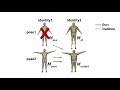
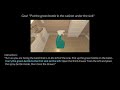
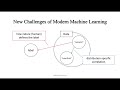
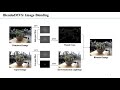
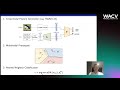
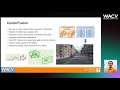