Trustworthy AI: Adversarially (non-)Robust ML | Nicholas Carlini Google AI | AI FOR GOOD DISCOVERY
One of the key limitations of deep-learning is its inability to generalize to new domains. The focus of this talk is on adversarial examples; inputs constructed by an adversary to mislead a machine-learning model. These adversarial examples can, for example, cause self-driving cars to misrecognize street signs or misidentify pedestrians.
This talk introduces how adversarial examples are generated and why they are so easy to find. Then, we consider recent attempts at increasing the robustness of neural networks. Across recent papers, we have studied several dozen defences proposed at top machine-learning and security conferences and found that almost all can be evaded and offer nearly no improvement on top of the undefended baselines. Worryingly, our most recent breaks require no new attack ideas and merely re-use earlier attack approaches.
General robustness is still a challenge for deep-learning and one that will require extensive work to solve.
SHOWNOTES
5:00 Start of presentation: Adversarially (non-)robust machine learning
5:26 How powerful is AI and how useful is it?
6:40 What if you deploy a model in the real world and there is an adversary?
9:06 When do we need machine learning models to be robust?
11:45 How do we generate adversarial examples?
18:20 Let's defend against adversarial examples
20:40 How are adversarial attacks today compared to 2018?
24:00 How are adversarial attacks reused?
26:40 The problem of adversarial attacks is methodological
33:30 What do adversarial loss functions look like?
36:50 What’s next in fighting adversarial attacks?
39:00 Can the status of fighting against adversarial attacks be compared with the status of cryptography in the 1990s?
44:57 Claim: we are crypto pre-Shannon
48:26 Brief conclusion
49:30 Question: Does the bottom-up approach of neural networks have something to do with the vulnerability?
54:28 Question: Is there a trade-off between robustness and accuracy?
57:48 Question: Can explainability help defend against adversarial attacks?
59:13 Question: What is one of the most promising approaches to fight against adversarial attacks?
1:02:00 Question: Can we get rid of some of the vulnerability of neural networks by moving towards more generative models?
1:06:22 Question: Can we optimise robustness with the probability that an attack can happen?
WHAT IS TRUSTWORTHY AI SERIES?
Artificial Intelligence (AI) systems have steadily grown in complexity, gaining predictivity often at the expense of interpretability, robustness and trustworthiness. Deep neural networks are a prime example of this development. While reaching “superhuman” performances in various complex tasks, these models are susceptible to errors when confronted with tiny (adversarial) variations of the input – variations which are either not noticeable or can be handled reliably by humans. This expert talk series will discuss these challenges of current AI technology and will present new research aiming at overcoming these limitations and developing AI systems which can be certified to be trustworthy and robust.
Website: https://aiforgood.itu.int/
Twitter: https://twitter.com/ITU_AIForGood
LinkedIn Page: https://www.linkedin.com/company/26511907
LinkedIn Group: https://www.linkedin.com/groups/8567748
Instagram: https://www.instagram.com/aiforgood
Facebook: https://www.facebook.com/AIforGood
Видео Trustworthy AI: Adversarially (non-)Robust ML | Nicholas Carlini Google AI | AI FOR GOOD DISCOVERY канала AI for Good
This talk introduces how adversarial examples are generated and why they are so easy to find. Then, we consider recent attempts at increasing the robustness of neural networks. Across recent papers, we have studied several dozen defences proposed at top machine-learning and security conferences and found that almost all can be evaded and offer nearly no improvement on top of the undefended baselines. Worryingly, our most recent breaks require no new attack ideas and merely re-use earlier attack approaches.
General robustness is still a challenge for deep-learning and one that will require extensive work to solve.
SHOWNOTES
5:00 Start of presentation: Adversarially (non-)robust machine learning
5:26 How powerful is AI and how useful is it?
6:40 What if you deploy a model in the real world and there is an adversary?
9:06 When do we need machine learning models to be robust?
11:45 How do we generate adversarial examples?
18:20 Let's defend against adversarial examples
20:40 How are adversarial attacks today compared to 2018?
24:00 How are adversarial attacks reused?
26:40 The problem of adversarial attacks is methodological
33:30 What do adversarial loss functions look like?
36:50 What’s next in fighting adversarial attacks?
39:00 Can the status of fighting against adversarial attacks be compared with the status of cryptography in the 1990s?
44:57 Claim: we are crypto pre-Shannon
48:26 Brief conclusion
49:30 Question: Does the bottom-up approach of neural networks have something to do with the vulnerability?
54:28 Question: Is there a trade-off between robustness and accuracy?
57:48 Question: Can explainability help defend against adversarial attacks?
59:13 Question: What is one of the most promising approaches to fight against adversarial attacks?
1:02:00 Question: Can we get rid of some of the vulnerability of neural networks by moving towards more generative models?
1:06:22 Question: Can we optimise robustness with the probability that an attack can happen?
WHAT IS TRUSTWORTHY AI SERIES?
Artificial Intelligence (AI) systems have steadily grown in complexity, gaining predictivity often at the expense of interpretability, robustness and trustworthiness. Deep neural networks are a prime example of this development. While reaching “superhuman” performances in various complex tasks, these models are susceptible to errors when confronted with tiny (adversarial) variations of the input – variations which are either not noticeable or can be handled reliably by humans. This expert talk series will discuss these challenges of current AI technology and will present new research aiming at overcoming these limitations and developing AI systems which can be certified to be trustworthy and robust.
Website: https://aiforgood.itu.int/
Twitter: https://twitter.com/ITU_AIForGood
LinkedIn Page: https://www.linkedin.com/company/26511907
LinkedIn Group: https://www.linkedin.com/groups/8567748
Instagram: https://www.instagram.com/aiforgood
Facebook: https://www.facebook.com/AIforGood
Видео Trustworthy AI: Adversarially (non-)Robust ML | Nicholas Carlini Google AI | AI FOR GOOD DISCOVERY канала AI for Good
Показать
Комментарии отсутствуют
Информация о видео
Другие видео канала
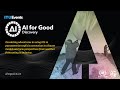
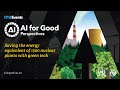
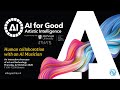
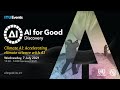
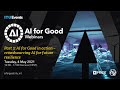
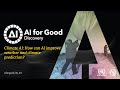
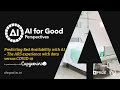
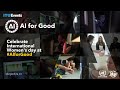
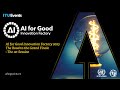
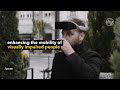
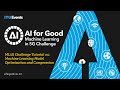
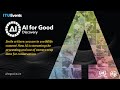
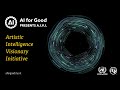
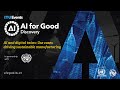
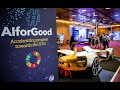
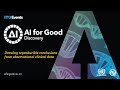

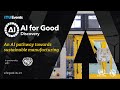
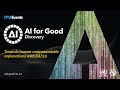
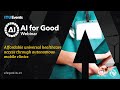
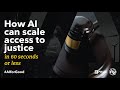