Tutorial 3A Hands on Explainable Recommender Systems with Knowledge Graphs
RecSys 2021 RecSys 2022 Hands on Explainable Recommender Systems with Knowledge Graphs
by Giacomo Balloccu (University of Cagliari, Italy), Ludovico Boratto (University of Cagliari, Italy),
Gianni Fenu (University of Cagliari, Italy), and Mirko Marras (University of Cagliari, Italy)
Regulations, such as the European General Data Protection Regulation (GDPR), call for a right to explanation, meaning that, under certain conditions, it is mandatory by law to generate awareness for the users on how a model behaves. Explanations have been also proved to have benefits from a business perspective, by increasing trust in the system, helping the users make a decision faster, and persuading a user to try and buy. A notable class of decision-support systems that urges supporting explanations are recommender systems, which often act as black boxes. Concerted efforts have been devoted to challenge these black boxes to make recommendation a transparent social process, by augmenting traditional models representing user-product interactions with external knowledge, often modeled as knowledge graphs, about the products and the users.
The goal of this tutorial is to present the RecSys community with recent advances on explainable recommender systems with knowledge graphs. We will first introduce conceptual foundations, by surveying the state of the art and describing real-world examples of how knowledge graphs are being integrated into the recommendation pipeline. This tutorial will continue with a systematic presentation of algorithmic solutions to model, integrate, train, and assess a recommender system with knowledge graphs, with particular attention to the explainability perspective. A practical part will then provide a series of concrete implementations, leveraging open-source tools and public datasets; in this part, tutorial participants will be engaged in the design of explanations accompanying the recommendations and in articulating their impact. We conclude this tutorial by analyzing emerging open issues and future directions in this vibrant research area.
Website: https://explainablerecsys.github.io/recsys2022/
Видео Tutorial 3A Hands on Explainable Recommender Systems with Knowledge Graphs канала ACM RecSys
by Giacomo Balloccu (University of Cagliari, Italy), Ludovico Boratto (University of Cagliari, Italy),
Gianni Fenu (University of Cagliari, Italy), and Mirko Marras (University of Cagliari, Italy)
Regulations, such as the European General Data Protection Regulation (GDPR), call for a right to explanation, meaning that, under certain conditions, it is mandatory by law to generate awareness for the users on how a model behaves. Explanations have been also proved to have benefits from a business perspective, by increasing trust in the system, helping the users make a decision faster, and persuading a user to try and buy. A notable class of decision-support systems that urges supporting explanations are recommender systems, which often act as black boxes. Concerted efforts have been devoted to challenge these black boxes to make recommendation a transparent social process, by augmenting traditional models representing user-product interactions with external knowledge, often modeled as knowledge graphs, about the products and the users.
The goal of this tutorial is to present the RecSys community with recent advances on explainable recommender systems with knowledge graphs. We will first introduce conceptual foundations, by surveying the state of the art and describing real-world examples of how knowledge graphs are being integrated into the recommendation pipeline. This tutorial will continue with a systematic presentation of algorithmic solutions to model, integrate, train, and assess a recommender system with knowledge graphs, with particular attention to the explainability perspective. A practical part will then provide a series of concrete implementations, leveraging open-source tools and public datasets; in this part, tutorial participants will be engaged in the design of explanations accompanying the recommendations and in articulating their impact. We conclude this tutorial by analyzing emerging open issues and future directions in this vibrant research area.
Website: https://explainablerecsys.github.io/recsys2022/
Видео Tutorial 3A Hands on Explainable Recommender Systems with Knowledge Graphs канала ACM RecSys
Показать
Комментарии отсутствуют
Информация о видео
Другие видео канала
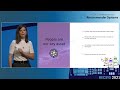
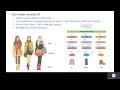
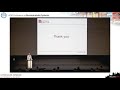
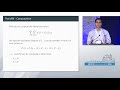
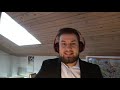
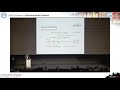
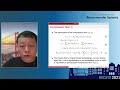
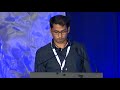
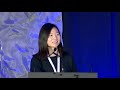
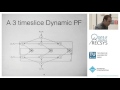
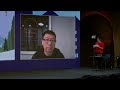
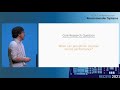
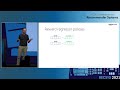
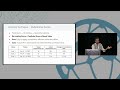
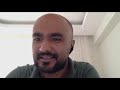
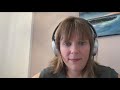
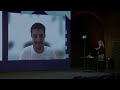


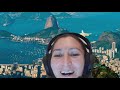
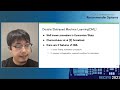