Shawn Scully: Production and Beyond: Deploying and Managing Machine Learning Models
PyData NYC 2015
Machine learning has become the key component in building intelligence-infused applications. However, as companies increase the number of such deployments, the number of machine learning models that need to be created, maintained, monitored, tracked, and improved grow at a tremendous pace. This growth has lead to a huge (and well-documented) accumulation of technical debt.
Developing a machine learning application is an iterative process that involves building multiple models over a dataset. The dataset itself evolves over time as new features and new data points are collected. Furthermore, once deployed, the models require updates over time. Changes in models and datasets become difficult to track over time, and one can quickly lose track of which version of the model used which data and why it was subsequently replaced.
In this talk, we outline some of the key challenges in large-scale deployments of many interacting machine learning models. We then describe a methodology for management, monitoring, and optimization of such models in production, which helps mitigate the technical debt.
In particular, we demonstrate how to:
Track models and versions, and visualize their quality over time
Track the provenance of models and datasets, and quantify how changes in data impact the models being served
Optimize model ensembles in real time, based on changing data, and provide alerts when such ensembles no longer provide the desired accuracy. 00:00 Welcome!
00:10 Help us add time stamps or captions to this video! See the description for details.
Want to help add timestamps to our YouTube videos to help with discoverability? Find out more here: https://github.com/numfocus/YouTubeVideoTimestamps
Видео Shawn Scully: Production and Beyond: Deploying and Managing Machine Learning Models канала PyData
Machine learning has become the key component in building intelligence-infused applications. However, as companies increase the number of such deployments, the number of machine learning models that need to be created, maintained, monitored, tracked, and improved grow at a tremendous pace. This growth has lead to a huge (and well-documented) accumulation of technical debt.
Developing a machine learning application is an iterative process that involves building multiple models over a dataset. The dataset itself evolves over time as new features and new data points are collected. Furthermore, once deployed, the models require updates over time. Changes in models and datasets become difficult to track over time, and one can quickly lose track of which version of the model used which data and why it was subsequently replaced.
In this talk, we outline some of the key challenges in large-scale deployments of many interacting machine learning models. We then describe a methodology for management, monitoring, and optimization of such models in production, which helps mitigate the technical debt.
In particular, we demonstrate how to:
Track models and versions, and visualize their quality over time
Track the provenance of models and datasets, and quantify how changes in data impact the models being served
Optimize model ensembles in real time, based on changing data, and provide alerts when such ensembles no longer provide the desired accuracy. 00:00 Welcome!
00:10 Help us add time stamps or captions to this video! See the description for details.
Want to help add timestamps to our YouTube videos to help with discoverability? Find out more here: https://github.com/numfocus/YouTubeVideoTimestamps
Видео Shawn Scully: Production and Beyond: Deploying and Managing Machine Learning Models канала PyData
Показать
Комментарии отсутствуют
Информация о видео
Другие видео канала
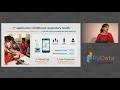
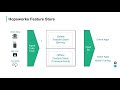
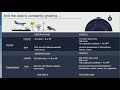
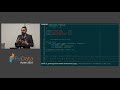
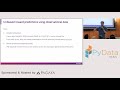
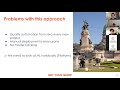
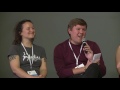
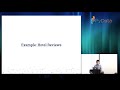
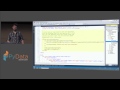
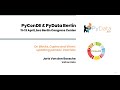

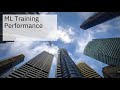

![Allen Downey: Bayesian Decision Analysis [Tutorial] | PyData Global 2022](https://i.ytimg.com/vi/fsdbneHgi58/default.jpg)
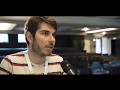
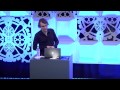


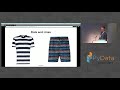
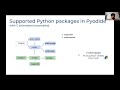
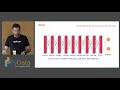