Alex Byaly: Causal Graphs for Procedural Generation
Causal graphs are models that describe possible causal relationships between things. They're typically used during inference: given some source of data, how can we explain the patterns we see? This talk is about doing the opposite. Instead of using them to learn about data, we'll generate it.
This is useful because a natural-seeming choice when procedurally generating data leads to something really strange. Imagine you roll some attributes independently, say hometown and favorite food. If you look at the results in Seattle, the favorite foods end up the same as for Tokyo. There is no relationship between the attributes. In the real world scientific studies have to be careful so their results aren't undermined by selection bias. If you only survey middle aged women in Nebraska your results might not generalize. A causal graph can introduce these kinds of relationships into generated content.
Lead-in music generated by Sonat Uzun.
Видео Alex Byaly: Causal Graphs for Procedural Generation канала Roguelike Celebration
This is useful because a natural-seeming choice when procedurally generating data leads to something really strange. Imagine you roll some attributes independently, say hometown and favorite food. If you look at the results in Seattle, the favorite foods end up the same as for Tokyo. There is no relationship between the attributes. In the real world scientific studies have to be careful so their results aren't undermined by selection bias. If you only survey middle aged women in Nebraska your results might not generalize. A causal graph can introduce these kinds of relationships into generated content.
Lead-in music generated by Sonat Uzun.
Видео Alex Byaly: Causal Graphs for Procedural Generation канала Roguelike Celebration
Показать
Комментарии отсутствуют
Информация о видео
Другие видео канала
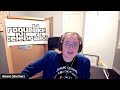
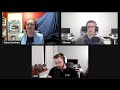
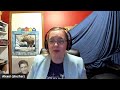

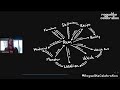

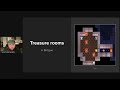
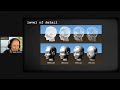
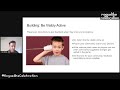
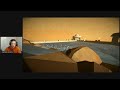
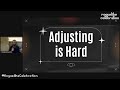
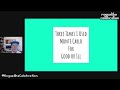

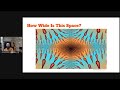
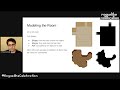

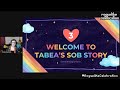
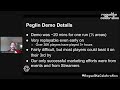
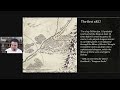
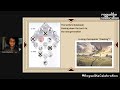