Sector-specific forecasting of volatility from Twitter sentiment
Speakers: Shiruo Liang, Joe Wee Sham, Yiming Song (CSUREMM Group 2)
Abstract: Volatility is a highly useful metric that informs investors about risk and potential returns. In this paper, we examine the usefulness of Twitter sentiment data when applied to the prediction of volatility across 11 different market sec- tors. We first compute the Pearson correlation and test for Granger causality and find statistically significant relationships between market sentiment and volatility for certain sectors, notably the technology and consumer discretionary sectors. We then apply these findings to four different machine learning models. We compare cross-model performance and find that a long short-term memory (LSTM) model outperforms all other models (linear regression, decision tree, XGBoost) when pre- dicting future volatility, with an order-of-magnitude lower mean absolute percentage error, both with and without sentiment data input. The LSTM model is also the only model that has a statistically significant improvement upon inclusion of sentiment data, suggesting that the true underlying relationship between sentiment and future volatility is highly complex and difficult to capture with conventional machine learning models that rely on regression alone.
Видео Sector-specific forecasting of volatility from Twitter sentiment канала Columbia University Department of Mathematics
Abstract: Volatility is a highly useful metric that informs investors about risk and potential returns. In this paper, we examine the usefulness of Twitter sentiment data when applied to the prediction of volatility across 11 different market sec- tors. We first compute the Pearson correlation and test for Granger causality and find statistically significant relationships between market sentiment and volatility for certain sectors, notably the technology and consumer discretionary sectors. We then apply these findings to four different machine learning models. We compare cross-model performance and find that a long short-term memory (LSTM) model outperforms all other models (linear regression, decision tree, XGBoost) when pre- dicting future volatility, with an order-of-magnitude lower mean absolute percentage error, both with and without sentiment data input. The LSTM model is also the only model that has a statistically significant improvement upon inclusion of sentiment data, suggesting that the true underlying relationship between sentiment and future volatility is highly complex and difficult to capture with conventional machine learning models that rely on regression alone.
Видео Sector-specific forecasting of volatility from Twitter sentiment канала Columbia University Department of Mathematics
Показать
Комментарии отсутствуют
Информация о видео
10 августа 2023 г. 18:30:28
00:11:37
Другие видео канала

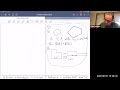
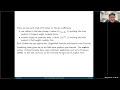

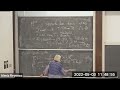
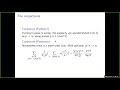

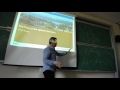
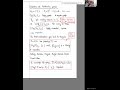
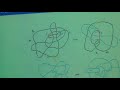
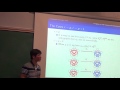
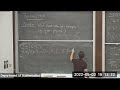
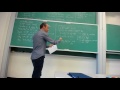
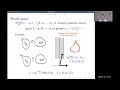
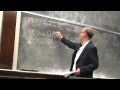
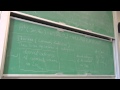
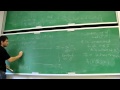
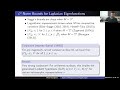

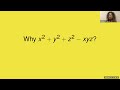
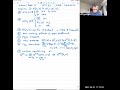