RecSys 2016: Paper Session 11 - Personalized Recommendations using Knowledge Graphs
Rose Catherine, William Cohen
https://doi.org/10.1145/2959100.2959131
Improving the performance of recommender systems using knowledge graphs is an important task. There have been many hybrid systems proposed in the past that use a mix of content-based and collaborative filtering techniques to boost the performance. More recently, some work has focused on recommendations that use external knowledge graphs (KGs) to supplement content-based recommendation. In this paper, we investigate three methods for making KG based recommendations using a general-purpose probabilistic logic system called ProPPR. The simplest of the models, EntitySim, uses only the links of the graph. We then extend the model to TypeSim that also uses the types of the entities to boost its generalization capabilities. Next, we develop a graph based latent factor model, GraphLF, which combines the strengths of latent factorization with graphs. We compare our approaches to a recently proposed state-of-the-art graph recommendation method on two large datasets, Yelp and MovieLens-100K. The experiments illustrate that our approaches can give large performance improvements. Additionally, we demonstrate that knowledge graphs give maximum advantage when the dataset is sparse, and gradually become redundant as more training data becomes available, and hence are most useful in cold-start settings.
Видео RecSys 2016: Paper Session 11 - Personalized Recommendations using Knowledge Graphs канала ACM RecSys
https://doi.org/10.1145/2959100.2959131
Improving the performance of recommender systems using knowledge graphs is an important task. There have been many hybrid systems proposed in the past that use a mix of content-based and collaborative filtering techniques to boost the performance. More recently, some work has focused on recommendations that use external knowledge graphs (KGs) to supplement content-based recommendation. In this paper, we investigate three methods for making KG based recommendations using a general-purpose probabilistic logic system called ProPPR. The simplest of the models, EntitySim, uses only the links of the graph. We then extend the model to TypeSim that also uses the types of the entities to boost its generalization capabilities. Next, we develop a graph based latent factor model, GraphLF, which combines the strengths of latent factorization with graphs. We compare our approaches to a recently proposed state-of-the-art graph recommendation method on two large datasets, Yelp and MovieLens-100K. The experiments illustrate that our approaches can give large performance improvements. Additionally, we demonstrate that knowledge graphs give maximum advantage when the dataset is sparse, and gradually become redundant as more training data becomes available, and hence are most useful in cold-start settings.
Видео RecSys 2016: Paper Session 11 - Personalized Recommendations using Knowledge Graphs канала ACM RecSys
Показать
Комментарии отсутствуют
Информация о видео
Другие видео канала
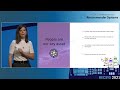
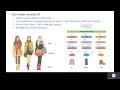
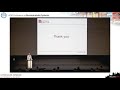
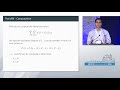
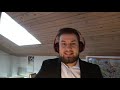
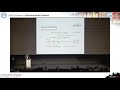
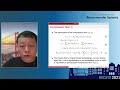
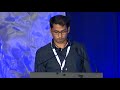
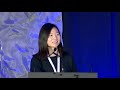
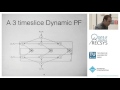
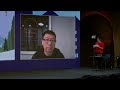
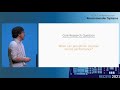
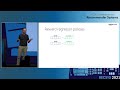
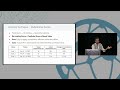
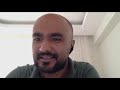
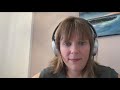
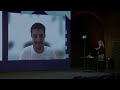


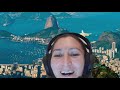
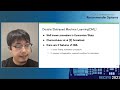