Homography Estimation with RANSAC📊
RANSAC vs Other Algorithms Which is Better for Computer Vision
I Mastered RANSAC in Computer Vision and Found Out Its POWER
WARNING Using RANSAC Incorrectly Could Cost You Precious Time
What Will Computer Vision Look Like in 10 Years with RANSAC
Exploring RANSAC in Computer Vision: Understanding its Voting Scheme and Assumptions for Robust Model Fitting.
[00:03](https://www.youtube.com/watch?v=hOupLt9aPL8&t=3) Introduction to RANSAC in computer vision.
- RANSAC, or Random Sample Consensus, is a robust method for model fitting in the presence of outliers.
- The philosophy of RANSAC involves iteratively selecting random subsets of data to improve the accuracy of model estimation.
[00:12](https://www.youtube.com/watch?v=hOupLt9aPL8&t=12) Understanding the RANSAC philosophy and its voting mechanism.
- RANSAC employs a voting scheme where subsets of data points are randomly selected to form hypotheses.
- The algorithm assesses consensus by comparing the standard deviation of the inner noise for each hypothesis.
[00:20](https://www.youtube.com/watch?v=hOupLt9aPL8&t=20) Outlier features disrupt model voting in RANSAC's strategy.
- Outlier features in data tend to vote inconsistently, which hampers identifying a reliable model within RANSAC.
- RANSAC relies on having enough consensus among valid features to effectively determine and validate a robust model.
[00:28](https://www.youtube.com/watch?v=hOupLt9aPL8&t=28) RANSAC requires a minimum number of points and noise parameters for effective hypothesis testing.
- Selecting the minimal elemental subset is crucial for forming reliable hypotheses in RANSAC.
- Users must define the standard deviation of noise beforehand to ensure proper algorithm performance.
[00:36](https://www.youtube.com/watch?v=hOupLt9aPL8&t=36) Challenges in achieving consistent feature agreement for model fitting.
- The effectiveness of features varies across different models, impacting overall accuracy.
- A sufficient quantity of features is crucial for establishing a reliable model.
[00:44](https://www.youtube.com/watch?v=hOupLt9aPL8&t=44) RANSAC uses random sampling for hypothesizing data subsets.
- The voting scheme involves selecting a random sample of data points to form a hypothesis.
- This method helps identify reliable data patterns despite the presence of outliers.
[00:54](https://www.youtube.com/watch?v=hOupLt9aPL8&t=54) RANSAC effectively ignores outliers by using minimal samples.
- RANSAC targets subsets of data to find a model that best fits, thereby reducing the influence of outliers.
- Users must specify the standard deviation of noise in advance to improve model accuracy.
[01:02](https://www.youtube.com/watch?v=hOupLt9aPL8&t=62) RANSAC effectively fits models despite noisy data.
- It isolates the best data points to minimize the influence of outliers.
- RANSAC iteratively selects random subsets of data to identify a robust model.
**Basic Philosophy of RANSAC**
- RANSAC stands for Random Sample Consensus, a model used in computer vision to estimate parameters of a mathematical model from a set of observed data.
- The method employs a voting scheme where a minimal number of points are randomly selected to create hypotheses for model fitting.
- Before executing the algorithm, the user must specify the standard deviation of the inline noise, which represents variations in actual data.
**Key Assumptions of RANSAC**
- Assumption One: Outlier features in the dataset do not consistently support any single model, which helps RANSAC focus on fitting the best model.
- Assumption Two: There exists a sufficient number of inlier features that can agree on a good model, ensuring robustness in the model estimation process.
- These assumptions are crucial for the effectiveness of RANSAC in distinguishing between inliers and outliers.
**Functionality of RANSAC**
- The algorithm randomly samples a small subset of available data points to hypothesize a model, which minimizes the influence of outliers.
- RANSAC iteratively refines the model by evaluating how well the random samples fit the data, thereby selecting the best-fitting model based on consensus.
- The focus on minimal samples allows RANSAC to efficiently handle datasets with a high fraction of outliers, making it widely applicable in various computer vision tasks.
Видео Homography Estimation with RANSAC📊 канала Talent Navigator
I Mastered RANSAC in Computer Vision and Found Out Its POWER
WARNING Using RANSAC Incorrectly Could Cost You Precious Time
What Will Computer Vision Look Like in 10 Years with RANSAC
Exploring RANSAC in Computer Vision: Understanding its Voting Scheme and Assumptions for Robust Model Fitting.
[00:03](https://www.youtube.com/watch?v=hOupLt9aPL8&t=3) Introduction to RANSAC in computer vision.
- RANSAC, or Random Sample Consensus, is a robust method for model fitting in the presence of outliers.
- The philosophy of RANSAC involves iteratively selecting random subsets of data to improve the accuracy of model estimation.
[00:12](https://www.youtube.com/watch?v=hOupLt9aPL8&t=12) Understanding the RANSAC philosophy and its voting mechanism.
- RANSAC employs a voting scheme where subsets of data points are randomly selected to form hypotheses.
- The algorithm assesses consensus by comparing the standard deviation of the inner noise for each hypothesis.
[00:20](https://www.youtube.com/watch?v=hOupLt9aPL8&t=20) Outlier features disrupt model voting in RANSAC's strategy.
- Outlier features in data tend to vote inconsistently, which hampers identifying a reliable model within RANSAC.
- RANSAC relies on having enough consensus among valid features to effectively determine and validate a robust model.
[00:28](https://www.youtube.com/watch?v=hOupLt9aPL8&t=28) RANSAC requires a minimum number of points and noise parameters for effective hypothesis testing.
- Selecting the minimal elemental subset is crucial for forming reliable hypotheses in RANSAC.
- Users must define the standard deviation of noise beforehand to ensure proper algorithm performance.
[00:36](https://www.youtube.com/watch?v=hOupLt9aPL8&t=36) Challenges in achieving consistent feature agreement for model fitting.
- The effectiveness of features varies across different models, impacting overall accuracy.
- A sufficient quantity of features is crucial for establishing a reliable model.
[00:44](https://www.youtube.com/watch?v=hOupLt9aPL8&t=44) RANSAC uses random sampling for hypothesizing data subsets.
- The voting scheme involves selecting a random sample of data points to form a hypothesis.
- This method helps identify reliable data patterns despite the presence of outliers.
[00:54](https://www.youtube.com/watch?v=hOupLt9aPL8&t=54) RANSAC effectively ignores outliers by using minimal samples.
- RANSAC targets subsets of data to find a model that best fits, thereby reducing the influence of outliers.
- Users must specify the standard deviation of noise in advance to improve model accuracy.
[01:02](https://www.youtube.com/watch?v=hOupLt9aPL8&t=62) RANSAC effectively fits models despite noisy data.
- It isolates the best data points to minimize the influence of outliers.
- RANSAC iteratively selects random subsets of data to identify a robust model.
**Basic Philosophy of RANSAC**
- RANSAC stands for Random Sample Consensus, a model used in computer vision to estimate parameters of a mathematical model from a set of observed data.
- The method employs a voting scheme where a minimal number of points are randomly selected to create hypotheses for model fitting.
- Before executing the algorithm, the user must specify the standard deviation of the inline noise, which represents variations in actual data.
**Key Assumptions of RANSAC**
- Assumption One: Outlier features in the dataset do not consistently support any single model, which helps RANSAC focus on fitting the best model.
- Assumption Two: There exists a sufficient number of inlier features that can agree on a good model, ensuring robustness in the model estimation process.
- These assumptions are crucial for the effectiveness of RANSAC in distinguishing between inliers and outliers.
**Functionality of RANSAC**
- The algorithm randomly samples a small subset of available data points to hypothesize a model, which minimizes the influence of outliers.
- RANSAC iteratively refines the model by evaluating how well the random samples fit the data, thereby selecting the best-fitting model based on consensus.
- The focus on minimal samples allows RANSAC to efficiently handle datasets with a high fraction of outliers, making it widely applicable in various computer vision tasks.
Видео Homography Estimation with RANSAC📊 канала Talent Navigator
RANSAC RANSAC algorithm computer vision robust model fitting outlier detection feature matching image processing AI in vision Random Sample Consensus model estimation visual computing machine learning hypothesis testing computer vision 2025 deep learning noise reduction AI models vision algorithms CVPR image alignment data fitting inlier outlier separation visual AI error tolerance models RANSAC tutorial RANSAC explained cv summary
Комментарии отсутствуют
Информация о видео
16 апреля 2025 г. 7:00:46
00:02:12
Другие видео канала
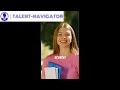
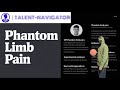
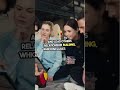
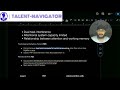
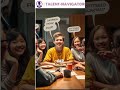
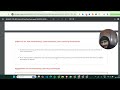
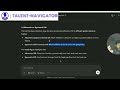
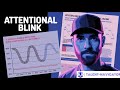
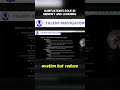
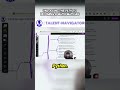

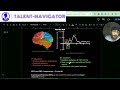


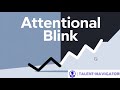
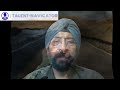
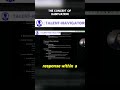
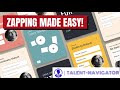
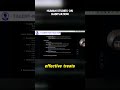
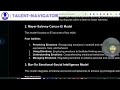