Stanford Seminar - Theories of inference for visual analysis
Jessica Hullman
Northwestern University
December 3, 2021
Research and development in computer science and statistics have produced increasingly sophisticated software interfaces for interactive and exploratory analysis, optimized for easy pattern finding and data exposure. But design philosophies that emphasize exploration over other phases of analysis risk confusing a need for flexibility with a conclusion that exploratory visual analysis is inherently model free and cannot be formalized. I will motivate how without a grounding in theories of human statistical inference, research in exploratory visual analysis can lead to contradictory interface objectives and representations of uncertainty that can discourage users from drawing valid inferences. I will discuss how the concept of a model check can help bridge traditionally exploratory and confirmatory activities, and suggest new directions for software and empirical research around exploratory and visual analysis, including how to facilitate external representation and exploration of candidate models during visual analysis. More broadly, I will motivate formalization of the role of graphics in statistical practice as a counterpoint to relying on intuition or brute force empiricism to guide research and practice.
Learn more about Stanford's Human-Computer Interaction Group: https://hci.stanford.edu
Learn about Stanford's Graduate Certificate in HCI: https://online.stanford.edu/programs/human-computer-interaction-graduate-certificate
View the full playlist: https://www.youtube.com/playlist?list=PLoROMvodv4rMyupDF2O00r19JsmolyXdD&disable_polymer=true
0:00 Introduction
1:02 Visualizations help offload cognition to perception
2:13 State-of-the-art: Optimize for easy pattern finding
8:03 Approaches to visualize distribution or uncertainty
8:47 Error bars/intervals violate expressiveness
10:54 Probability encodings often hard to read accurately
11:59 Frequency based uncertainty visualization
16:21 Evaluation: Effect size judgments
17:43 Mean difference heuristic in effect size judgments
18:40 Sensitivity to visual distance between means
20:14 Investigating the mean difference heuristic
22:18 Uncertainty visualization conditions
27:12 Bayesian cognition to understand belief updating
30:40 How well do visualizations support causal inference?
33:04 How does visualization support learning in analysis?
33:29 Good visualizations facilitate model checks
35:32 Implicit model checks give graphs their meaning
37:03 Graphical inference as Bayesian model check
39:31 Tableau-like system supporting model checks
41:24 From visualization to implied model
44:51 Open questions
46:35 What characterizes good inference problems for vis?
48:08 Bounding the value of a more expressive visualization
#inference
Видео Stanford Seminar - Theories of inference for visual analysis канала Stanford Online
Northwestern University
December 3, 2021
Research and development in computer science and statistics have produced increasingly sophisticated software interfaces for interactive and exploratory analysis, optimized for easy pattern finding and data exposure. But design philosophies that emphasize exploration over other phases of analysis risk confusing a need for flexibility with a conclusion that exploratory visual analysis is inherently model free and cannot be formalized. I will motivate how without a grounding in theories of human statistical inference, research in exploratory visual analysis can lead to contradictory interface objectives and representations of uncertainty that can discourage users from drawing valid inferences. I will discuss how the concept of a model check can help bridge traditionally exploratory and confirmatory activities, and suggest new directions for software and empirical research around exploratory and visual analysis, including how to facilitate external representation and exploration of candidate models during visual analysis. More broadly, I will motivate formalization of the role of graphics in statistical practice as a counterpoint to relying on intuition or brute force empiricism to guide research and practice.
Learn more about Stanford's Human-Computer Interaction Group: https://hci.stanford.edu
Learn about Stanford's Graduate Certificate in HCI: https://online.stanford.edu/programs/human-computer-interaction-graduate-certificate
View the full playlist: https://www.youtube.com/playlist?list=PLoROMvodv4rMyupDF2O00r19JsmolyXdD&disable_polymer=true
0:00 Introduction
1:02 Visualizations help offload cognition to perception
2:13 State-of-the-art: Optimize for easy pattern finding
8:03 Approaches to visualize distribution or uncertainty
8:47 Error bars/intervals violate expressiveness
10:54 Probability encodings often hard to read accurately
11:59 Frequency based uncertainty visualization
16:21 Evaluation: Effect size judgments
17:43 Mean difference heuristic in effect size judgments
18:40 Sensitivity to visual distance between means
20:14 Investigating the mean difference heuristic
22:18 Uncertainty visualization conditions
27:12 Bayesian cognition to understand belief updating
30:40 How well do visualizations support causal inference?
33:04 How does visualization support learning in analysis?
33:29 Good visualizations facilitate model checks
35:32 Implicit model checks give graphs their meaning
37:03 Graphical inference as Bayesian model check
39:31 Tableau-like system supporting model checks
41:24 From visualization to implied model
44:51 Open questions
46:35 What characterizes good inference problems for vis?
48:08 Bounding the value of a more expressive visualization
#inference
Видео Stanford Seminar - Theories of inference for visual analysis канала Stanford Online
Показать
Комментарии отсутствуют
Информация о видео
Другие видео канала
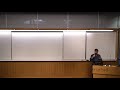
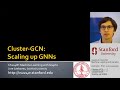
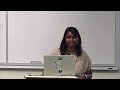
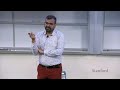
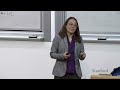
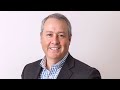
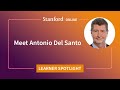
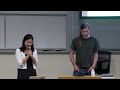
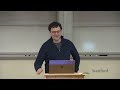
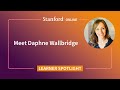
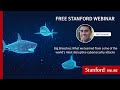
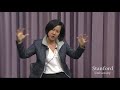
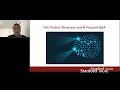
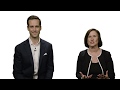
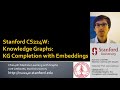
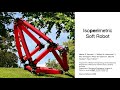

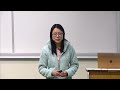
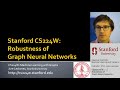
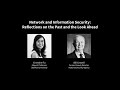
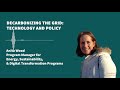