Memory-Based Graph Networks | AISC
Speaker(s): Amir Hosein Khasahmadi
Facilitator(s): Nabila Abraham
Find the recording, slides, and more info at https://ai.science/e/mem-gn-ns-memory-based-graph-networks--WDHBObBp3ILDK6dV0B9U
Motivation / Abstract
Graph neural networks (GNNs) are a class of deep models that operate on data with arbitrary topology represented as graphs. The authors propose an efficient memory layer for GNNs that can jointly learn node representations and but moreover, coarsen the graph representations which methods like GraphSAGE or GCN don't necessarily do. The authors introduce two new networks based on this layer: memory-based GNN (MemGNN) and graph memory network (GMN) that can learn hierarchical graph representations. The experimental results show that the proposed models achieve state-of-the-art results in eight out of nine graph classification and regression benchmarks. We also show that the learned representations could correspond to chemical features in the molecule data.
What was discussed?
- Relationship between GMN/MemGNNs and transformers
- Clustering loss function
- Interpreting the centroids learned
- Global topological embedding versus local topologies
------
#AISC hosts 3-5 live sessions like this on various AI research, engineering, and product topics every week! Visit https://ai.science for more details
Видео Memory-Based Graph Networks | AISC канала LLMs Explained - Aggregate Intellect - AI.SCIENCE
Facilitator(s): Nabila Abraham
Find the recording, slides, and more info at https://ai.science/e/mem-gn-ns-memory-based-graph-networks--WDHBObBp3ILDK6dV0B9U
Motivation / Abstract
Graph neural networks (GNNs) are a class of deep models that operate on data with arbitrary topology represented as graphs. The authors propose an efficient memory layer for GNNs that can jointly learn node representations and but moreover, coarsen the graph representations which methods like GraphSAGE or GCN don't necessarily do. The authors introduce two new networks based on this layer: memory-based GNN (MemGNN) and graph memory network (GMN) that can learn hierarchical graph representations. The experimental results show that the proposed models achieve state-of-the-art results in eight out of nine graph classification and regression benchmarks. We also show that the learned representations could correspond to chemical features in the molecule data.
What was discussed?
- Relationship between GMN/MemGNNs and transformers
- Clustering loss function
- Interpreting the centroids learned
- Global topological embedding versus local topologies
------
#AISC hosts 3-5 live sessions like this on various AI research, engineering, and product topics every week! Visit https://ai.science for more details
Видео Memory-Based Graph Networks | AISC канала LLMs Explained - Aggregate Intellect - AI.SCIENCE
Показать
Комментарии отсутствуют
Информация о видео
26 июня 2020 г. 10:03:27
00:57:53
Другие видео канала
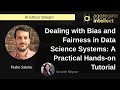

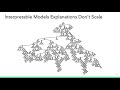
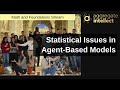
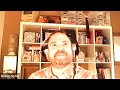
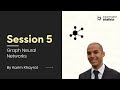


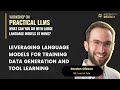
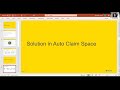
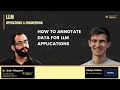
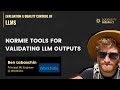
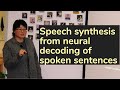
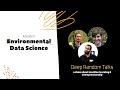
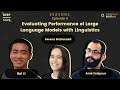

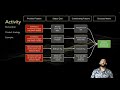
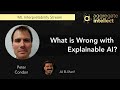
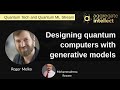
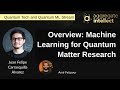