Detecting Hallucinated Content in Conditional Neural Sequence Generation (NLP Paper Walkthrough)
#textgeneration #nlp #languagemodel
Current days Text Generation system often generates false information, be it text summarization, machine translation, etc. This research from Carnegie Mellon University & Facebook talks about detecting such Hallucinations at token-level in Conditional Neural Text Generation systems using pre-trained language models trained over synthetic data.
⏩ Abstract: Neural sequence models can generate highly fluent sentences, but recent studies have also shown that they are also prone to hallucinate additional content not supported by the input. These variety of fluent but wrong outputs are particularly problematic, as it will not be possible for users to tell they are being presented incorrect content. To detect these errors, we propose a task to predict whether each token in the output sequence is hallucinated (not contained in the input) and collect new manually annotated evaluation sets for this task. We also introduce a method for learning to detect hallucinations using pre-trained language models fine tuned on synthetic data that includes automatically inserted hallucinations Experiments on machine translation (MT) and abstractive summarization demonstrate that our proposed approach consistently outperforms strong baselines on all benchmark datasets. We further demonstrate how to use the token-level hallucination labels to define a fine-grained loss over the target sequence in low-resource MT and achieve significant improvements over strong baseline methods. We also apply our method to word-level quality estimation for MT and show its effectiveness in both supervised and unsupervised settings. Codes and data available at this https URL.
Please feel free to share out the content and subscribe to my channel :)
⏩ Subscribe - https://youtube.com/channel/UCoz8NrwgL7U9535VNc0mRPA?sub_confirmation=1
⏩ OUTLINE:
0:00 - Abstract and Background
02:18 - Token level hallucination (Extrinsic and Intrinsic)
04:18 - Human Assessment of hallucinations
05:20 - Token level hallucination detection
06:02 - Synthetic Data Creation
08:15 - Label assignment
09:31 - Fine Tuning pre-trained language model on synthetic data
⏩ Paper Title: Detecting Hallucinated Content in Conditional Neural Sequence Generation
⏩ Paper: https://arxiv.org/abs/2011.02593
⏩ Code: https://github.com/violet-zct/fairseq-detect-hallucination
⏩ Author: Chunting Zhou, Graham Neubig, Jiatao Gu, Mona Diab, Paco Guzman, Luke Zettlemoyer, Marjan Ghazvininejad
⏩ Organisation: Carnegie Mellon University, Facebook AI Research
⏩ IMPORTANT LINKS
BART Paper Walkthrough: https://www.youtube.com/watch?v=BGWpNQHIcs4
Evaluating Text Generation Systems Paper Walkthrough: https://www.youtube.com/watch?v=-CIlz-5um7U
Entity-level factual inconsistency in Text Summarization: https://www.youtube.com/watch?v=P9wr8IBfDQs
*********************************************
If you want to support me financially which totally optional and voluntary ❤️
You can consider buying me chai ( because i don't drink coffee :) ) at https://www.buymeacoffee.com/TechvizCoffee
*********************************************
⏩ Youtube - https://www.youtube.com/c/TechVizTheDataScienceGuy
⏩ LinkedIn - https://linkedin.com/in/prakhar21
⏩ Medium - https://medium.com/@prakhar.mishra
⏩ GitHub - https://github.com/prakhar21
⏩ Twitter - https://twitter.com/rattller
*********************************************
Tools I use for making videos :)
⏩ iPad - https://tinyurl.com/y39p6pwc
⏩ Apple Pencil - https://tinyurl.com/y5rk8txn
⏩ GoodNotes - https://tinyurl.com/y627cfsa
#techviz #datascienceguy #machinelearning #machinetranslation #textsummarization
Видео Detecting Hallucinated Content in Conditional Neural Sequence Generation (NLP Paper Walkthrough) канала TechViz - The Data Science Guy
Current days Text Generation system often generates false information, be it text summarization, machine translation, etc. This research from Carnegie Mellon University & Facebook talks about detecting such Hallucinations at token-level in Conditional Neural Text Generation systems using pre-trained language models trained over synthetic data.
⏩ Abstract: Neural sequence models can generate highly fluent sentences, but recent studies have also shown that they are also prone to hallucinate additional content not supported by the input. These variety of fluent but wrong outputs are particularly problematic, as it will not be possible for users to tell they are being presented incorrect content. To detect these errors, we propose a task to predict whether each token in the output sequence is hallucinated (not contained in the input) and collect new manually annotated evaluation sets for this task. We also introduce a method for learning to detect hallucinations using pre-trained language models fine tuned on synthetic data that includes automatically inserted hallucinations Experiments on machine translation (MT) and abstractive summarization demonstrate that our proposed approach consistently outperforms strong baselines on all benchmark datasets. We further demonstrate how to use the token-level hallucination labels to define a fine-grained loss over the target sequence in low-resource MT and achieve significant improvements over strong baseline methods. We also apply our method to word-level quality estimation for MT and show its effectiveness in both supervised and unsupervised settings. Codes and data available at this https URL.
Please feel free to share out the content and subscribe to my channel :)
⏩ Subscribe - https://youtube.com/channel/UCoz8NrwgL7U9535VNc0mRPA?sub_confirmation=1
⏩ OUTLINE:
0:00 - Abstract and Background
02:18 - Token level hallucination (Extrinsic and Intrinsic)
04:18 - Human Assessment of hallucinations
05:20 - Token level hallucination detection
06:02 - Synthetic Data Creation
08:15 - Label assignment
09:31 - Fine Tuning pre-trained language model on synthetic data
⏩ Paper Title: Detecting Hallucinated Content in Conditional Neural Sequence Generation
⏩ Paper: https://arxiv.org/abs/2011.02593
⏩ Code: https://github.com/violet-zct/fairseq-detect-hallucination
⏩ Author: Chunting Zhou, Graham Neubig, Jiatao Gu, Mona Diab, Paco Guzman, Luke Zettlemoyer, Marjan Ghazvininejad
⏩ Organisation: Carnegie Mellon University, Facebook AI Research
⏩ IMPORTANT LINKS
BART Paper Walkthrough: https://www.youtube.com/watch?v=BGWpNQHIcs4
Evaluating Text Generation Systems Paper Walkthrough: https://www.youtube.com/watch?v=-CIlz-5um7U
Entity-level factual inconsistency in Text Summarization: https://www.youtube.com/watch?v=P9wr8IBfDQs
*********************************************
If you want to support me financially which totally optional and voluntary ❤️
You can consider buying me chai ( because i don't drink coffee :) ) at https://www.buymeacoffee.com/TechvizCoffee
*********************************************
⏩ Youtube - https://www.youtube.com/c/TechVizTheDataScienceGuy
⏩ LinkedIn - https://linkedin.com/in/prakhar21
⏩ Medium - https://medium.com/@prakhar.mishra
⏩ GitHub - https://github.com/prakhar21
⏩ Twitter - https://twitter.com/rattller
*********************************************
Tools I use for making videos :)
⏩ iPad - https://tinyurl.com/y39p6pwc
⏩ Apple Pencil - https://tinyurl.com/y5rk8txn
⏩ GoodNotes - https://tinyurl.com/y627cfsa
#techviz #datascienceguy #machinelearning #machinetranslation #textsummarization
Видео Detecting Hallucinated Content in Conditional Neural Sequence Generation (NLP Paper Walkthrough) канала TechViz - The Data Science Guy
Показать
Комментарии отсутствуют
Информация о видео
13 июня 2021 г. 17:11:08
00:12:25
Другие видео канала

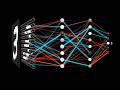
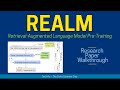

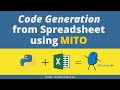
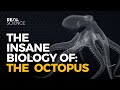
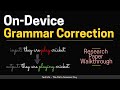
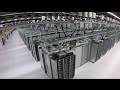
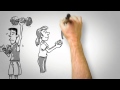
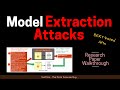
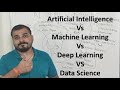
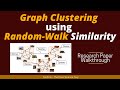
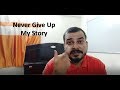
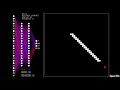
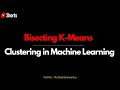

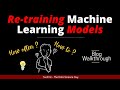
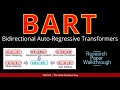
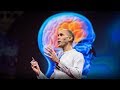
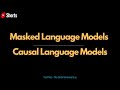