Targeted Adversarial Examples for Black Box Audio Systems
Targeted Adversarial Examples for Black Box Audio Systems
Amog Kamsetty (UC Berkeley)
Presented at the
2nd Deep Learning and Security Workshop
May 23, 2019
at the 2019 IEEE Symposium on Security & Privacy
San Francisco, CA
https://www.ieee-security.org/TC/SP2019/
https://www.ieee-security.org/TC/SPW2019/DLS/
ABSTRACT
The application of deep recurrent networks to audio transcription has led to impressive gains in automatic speech recognition (ASR) systems. Many have demonstrated that small adversarial perturbations can fool deep neural networks into incorrectly predicting a specified target with high confidence. Current work on fooling ASR systems have focused on white-box attacks, in which the model architecture and parameters are known. In this paper, we adopt a black-box approach to adversarial generation, combining the approaches of both genetic algorithms and gradient estimation to solve the task. We achieve a 89.25% targeted attack similarity, with 35% targeted attack success rate, after 3000 generations while maintaining 94.6% audio file similarity.
Видео Targeted Adversarial Examples for Black Box Audio Systems канала IEEE Symposium on Security and Privacy
Amog Kamsetty (UC Berkeley)
Presented at the
2nd Deep Learning and Security Workshop
May 23, 2019
at the 2019 IEEE Symposium on Security & Privacy
San Francisco, CA
https://www.ieee-security.org/TC/SP2019/
https://www.ieee-security.org/TC/SPW2019/DLS/
ABSTRACT
The application of deep recurrent networks to audio transcription has led to impressive gains in automatic speech recognition (ASR) systems. Many have demonstrated that small adversarial perturbations can fool deep neural networks into incorrectly predicting a specified target with high confidence. Current work on fooling ASR systems have focused on white-box attacks, in which the model architecture and parameters are known. In this paper, we adopt a black-box approach to adversarial generation, combining the approaches of both genetic algorithms and gradient estimation to solve the task. We achieve a 89.25% targeted attack similarity, with 35% targeted attack success rate, after 3000 generations while maintaining 94.6% audio file similarity.
Видео Targeted Adversarial Examples for Black Box Audio Systems канала IEEE Symposium on Security and Privacy
Показать
Комментарии отсутствуют
Информация о видео
26 сентября 2019 г. 22:22:29
00:21:55
Другие видео канала
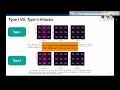
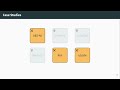
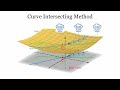
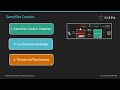
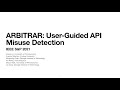


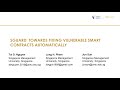
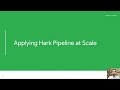
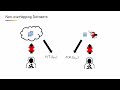
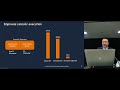
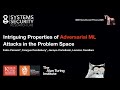
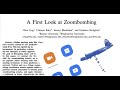

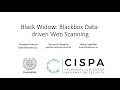
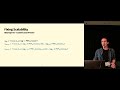
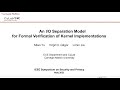
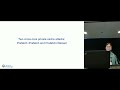
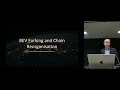
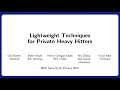
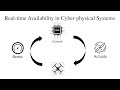