MIA: Bo Xia, In silico screening for genome structure; Ruochi Zhang, graph representation learning
Models. Inference and Algorithms
February 8, 2023
Broad Institute of MIT and Harvard
Meeting (53:45): High-throughput in silico genetic screen for discovering novel 3D genome organization regulation
Bo Xia
Gene Regulation Observatory, Broad Institute; Society of Fellows, Harvard University
For decades, genetic screen has been central in identifying functional genes and beyond. Traditional genetic screen typically requires a large-scale experimental setup, and is limited by the available resource and experimental feasibility. In the 3D genome organization field, experimental methods, such as Hi-C, are generally costly and bear strong technical limitations, thus restricting their widespread application particularly in high-throughput genetic screen for biological discoveries. To solve this challenge, we recently proposed and developed in silico genetic screen (ISGS) research framework as the next-generation approach to genetic discoveries. Similar to the experimental genetic screen, the ISGS framework interrogates the effect of genetic perturbation through accurate computational modeling in an ultra-high-throughput scenario. To demonstrate the ISGS framework in the genome organization field, we developed C.Origami, a deep neural network that performs accurate de novo prediction of cell type-specific chromatin organization. C.Origami enables high-throughput ISGS to discover regulatory DNA elements on chromatin organization. Applying this approach to leukemia cells and normal T cells, we demonstrate that cell-type-specific in silico genetic screen, enabled by C.Origami, can be used to systematically discover novel chromatin regulation circuits in both normal and disease-related biological systems.
Primer (00:32): Charting the Landscape of 3D Genome Organization with Graph Representation Learning
Ruochi Zhang
Eric and Wendy Schmidt Center
The three-dimensional (3D) organization of chromosomes in eukaryotic cells is key to understanding cellular functions such as DNA replication and gene transcription.
Recent advances in (single-cell) high-throughput 3D genome mapping methods have ushered in a new era of unveiling spatial chromatin organization at an unprecedented resolution. However, there is a lack of algorithms that can effectively utilize these emerging data types and integrate them with other multimodal data. In this talk, I will introduce a series of new algorithms developed based on graph representation learning for the analysis of multiscale 3D genome organization. In particular, I will describe a generic hypergraph representation learning scheme called Hyper-SAGNN, which was one of the first methods that focus on hyperedge predictions for heterogeneous non-uniform hypergraphs. This framework was further extended to Higashi, an end-to-end machine learning solution for single-cell Hi-C analysis. I will demonstrate how Higashi, as the most systematic approach to date, reveals cell-to-cell variability of 3D genome features and the connections between 3D chromatin structure and cell-type specific gene regulation in complex brain tissues. Finally, I will highlight a more efficient and interpretable framework for the next generation single-cell 3D epigenome studies called Fast-Higashi based on scalable tensor decomposition. Together, these new methods have the potential to provide key insights into the structure and function connections of genome organization and will be of high value to a diverse group of biomedical researchers.
For more information visit: https://www.broadinstitute.org/talks/fall-2022/mia
Copyright Broad Institute, 2023. All rights reserved.
Видео MIA: Bo Xia, In silico screening for genome structure; Ruochi Zhang, graph representation learning канала Broad Institute
February 8, 2023
Broad Institute of MIT and Harvard
Meeting (53:45): High-throughput in silico genetic screen for discovering novel 3D genome organization regulation
Bo Xia
Gene Regulation Observatory, Broad Institute; Society of Fellows, Harvard University
For decades, genetic screen has been central in identifying functional genes and beyond. Traditional genetic screen typically requires a large-scale experimental setup, and is limited by the available resource and experimental feasibility. In the 3D genome organization field, experimental methods, such as Hi-C, are generally costly and bear strong technical limitations, thus restricting their widespread application particularly in high-throughput genetic screen for biological discoveries. To solve this challenge, we recently proposed and developed in silico genetic screen (ISGS) research framework as the next-generation approach to genetic discoveries. Similar to the experimental genetic screen, the ISGS framework interrogates the effect of genetic perturbation through accurate computational modeling in an ultra-high-throughput scenario. To demonstrate the ISGS framework in the genome organization field, we developed C.Origami, a deep neural network that performs accurate de novo prediction of cell type-specific chromatin organization. C.Origami enables high-throughput ISGS to discover regulatory DNA elements on chromatin organization. Applying this approach to leukemia cells and normal T cells, we demonstrate that cell-type-specific in silico genetic screen, enabled by C.Origami, can be used to systematically discover novel chromatin regulation circuits in both normal and disease-related biological systems.
Primer (00:32): Charting the Landscape of 3D Genome Organization with Graph Representation Learning
Ruochi Zhang
Eric and Wendy Schmidt Center
The three-dimensional (3D) organization of chromosomes in eukaryotic cells is key to understanding cellular functions such as DNA replication and gene transcription.
Recent advances in (single-cell) high-throughput 3D genome mapping methods have ushered in a new era of unveiling spatial chromatin organization at an unprecedented resolution. However, there is a lack of algorithms that can effectively utilize these emerging data types and integrate them with other multimodal data. In this talk, I will introduce a series of new algorithms developed based on graph representation learning for the analysis of multiscale 3D genome organization. In particular, I will describe a generic hypergraph representation learning scheme called Hyper-SAGNN, which was one of the first methods that focus on hyperedge predictions for heterogeneous non-uniform hypergraphs. This framework was further extended to Higashi, an end-to-end machine learning solution for single-cell Hi-C analysis. I will demonstrate how Higashi, as the most systematic approach to date, reveals cell-to-cell variability of 3D genome features and the connections between 3D chromatin structure and cell-type specific gene regulation in complex brain tissues. Finally, I will highlight a more efficient and interpretable framework for the next generation single-cell 3D epigenome studies called Fast-Higashi based on scalable tensor decomposition. Together, these new methods have the potential to provide key insights into the structure and function connections of genome organization and will be of high value to a diverse group of biomedical researchers.
For more information visit: https://www.broadinstitute.org/talks/fall-2022/mia
Copyright Broad Institute, 2023. All rights reserved.
Видео MIA: Bo Xia, In silico screening for genome structure; Ruochi Zhang, graph representation learning канала Broad Institute
Показать
Комментарии отсутствуют
Информация о видео
Другие видео канала
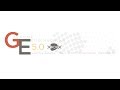

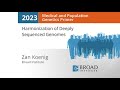
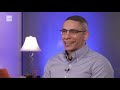

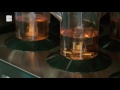

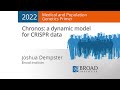
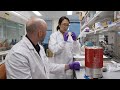
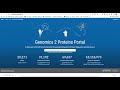
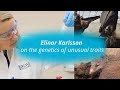
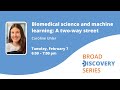
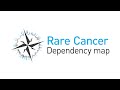

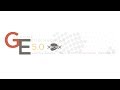
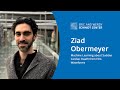
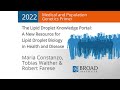
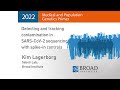
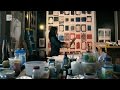
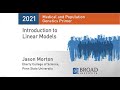