"Introduction to physics-informed neural networks" Liu Yang (Brown) - CFPU SMLI
Center for the Fundamental Physics of the Universe (CFPU) Student Machine Learning Initiative (SMLI) - Recorded October 27, 2020
https://cfpu.brown.edu
Introduction to physics-informed neural networks
Physics-informed neural networks (PINNs)[1] were proposed to solve supervised learning tasks while respecting any given laws of physics described by general nonlinear partial differential equations (PDEs), and have achieved great success in data-driven PDE solving (forward problems) as well as data-driven PDE discovery (inverse problems). In this talk, we will give a brief overview of physics-informed neural networks and its applications in fluid mechanics[2]. We will also introduce the Bayesian PINNs[3] where the aleatoric uncertainty arising from the noisy data is quantified in the Bayesian framework.
Reference:
[1] Raissi, M., Perdikaris, P., & Karniadakis, G. E. (2019). Physics-informed neural networks: A deep learning framework for solving forward and inverse problems involving nonlinear partial differential equations. Journal of Computational Physics, 378, 686-707.
[2] Raissi, M., Yazdani, A., & Karniadakis, G. E. (2020). Hidden fluid mechanics: Learning velocity and pressure fields from flow visualizations. Science, 367(6481), 1026-1030.
[3] Yang, L., Meng, X., & Karniadakis, G. E. (2020). B-pinns: Bayesian physics-informed neural networks for forward and inverse pde problems with noisy data. arXiv preprint arXiv:2003.06097.
Видео "Introduction to physics-informed neural networks" Liu Yang (Brown) - CFPU SMLI канала Brown University Department of Physics
https://cfpu.brown.edu
Introduction to physics-informed neural networks
Physics-informed neural networks (PINNs)[1] were proposed to solve supervised learning tasks while respecting any given laws of physics described by general nonlinear partial differential equations (PDEs), and have achieved great success in data-driven PDE solving (forward problems) as well as data-driven PDE discovery (inverse problems). In this talk, we will give a brief overview of physics-informed neural networks and its applications in fluid mechanics[2]. We will also introduce the Bayesian PINNs[3] where the aleatoric uncertainty arising from the noisy data is quantified in the Bayesian framework.
Reference:
[1] Raissi, M., Perdikaris, P., & Karniadakis, G. E. (2019). Physics-informed neural networks: A deep learning framework for solving forward and inverse problems involving nonlinear partial differential equations. Journal of Computational Physics, 378, 686-707.
[2] Raissi, M., Yazdani, A., & Karniadakis, G. E. (2020). Hidden fluid mechanics: Learning velocity and pressure fields from flow visualizations. Science, 367(6481), 1026-1030.
[3] Yang, L., Meng, X., & Karniadakis, G. E. (2020). B-pinns: Bayesian physics-informed neural networks for forward and inverse pde problems with noisy data. arXiv preprint arXiv:2003.06097.
Видео "Introduction to physics-informed neural networks" Liu Yang (Brown) - CFPU SMLI канала Brown University Department of Physics
Показать
Комментарии отсутствуют
Информация о видео
27 октября 2020 г. 23:46:10
00:38:47
Другие видео канала

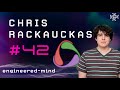
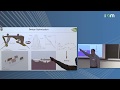
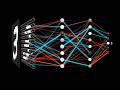
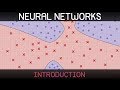
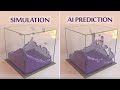

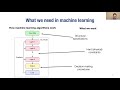
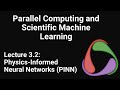
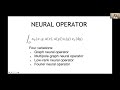
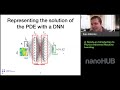
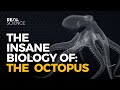
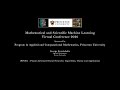

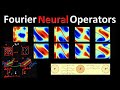
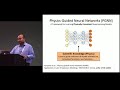
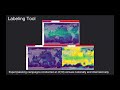
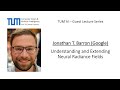
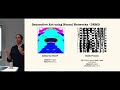
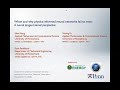