MedAI Session 30: GANs in Medical Image Synthesis, Translation, and Augmentation | Jason Jeong
Title: Applications of Generative Adversarial Networks (GANs) in Medical Image Synthesis, Translation, and Augmentation
Speaker: Jiwoong Jason Jeong
Abstract:
Medical imaging is a source of crucial information in modern healthcare. Deep learning models have been developed for various modalities such as CT, MRI, Ultrasound, and PET for automatic or semi-automatic diagnosis or assessment of diseases. While deep learning models have been proven to be very powerful, training such models sufficiently requires large, well-annotated but expensive datasets. However, medical images, especially those containing diseases, are rare. While there are a variety of solutions to improve models with limited and imbalanced datasets, one solution is generating these rare images through generative adversarial networks (GANs). In this presentation, I will present a quick review on the use of GANs in medical imaging tasks, specifically classification and segmentation. Then I will present and discuss our recent work on using GANs for generating synthetic dual energy CT (sDECT) from single energy CT (SECT). Finally, some interesting challenges and possible future directions of GANs in medical imaging will be discussed.
Speaker Bio:
Jiwoong Jason Jeong is a Ph.D. student in ASU’s Data Science, Analytics, and Engineering program. His research interest involves applying GANs into the medical workflow with a focus on solving medical data imbalance and scarcity. Previously, he completed his Master’s in Medical Physics at Georgia Institute of Technology.
------
The MedAI Group Exchange Sessions are a platform where we can critically examine key topics in AI and medicine, generate fresh ideas and discussion around their intersection and most importantly, learn from each other.
We will be having weekly sessions where invited speakers will give a talk presenting their work followed by an interactive discussion and Q&A. Our sessions are held every Thursday from 1pm-2pm PST.
To get notifications about upcoming sessions, please join our mailing list: https://mailman.stanford.edu/mailman/...
For more details about MedAI, check out our website: https://medai.stanford.edu. You can follow us on Twitter @MedaiStanford
Organized by members of the Rubin Lab (http://rubinlab.stanford.edu)
- Nandita Bhaskhar (https://www.stanford.edu/~nanbhas)
- Siyi Tang (https://siyitang.me)
Видео MedAI Session 30: GANs in Medical Image Synthesis, Translation, and Augmentation | Jason Jeong канала Stanford MedAI
Speaker: Jiwoong Jason Jeong
Abstract:
Medical imaging is a source of crucial information in modern healthcare. Deep learning models have been developed for various modalities such as CT, MRI, Ultrasound, and PET for automatic or semi-automatic diagnosis or assessment of diseases. While deep learning models have been proven to be very powerful, training such models sufficiently requires large, well-annotated but expensive datasets. However, medical images, especially those containing diseases, are rare. While there are a variety of solutions to improve models with limited and imbalanced datasets, one solution is generating these rare images through generative adversarial networks (GANs). In this presentation, I will present a quick review on the use of GANs in medical imaging tasks, specifically classification and segmentation. Then I will present and discuss our recent work on using GANs for generating synthetic dual energy CT (sDECT) from single energy CT (SECT). Finally, some interesting challenges and possible future directions of GANs in medical imaging will be discussed.
Speaker Bio:
Jiwoong Jason Jeong is a Ph.D. student in ASU’s Data Science, Analytics, and Engineering program. His research interest involves applying GANs into the medical workflow with a focus on solving medical data imbalance and scarcity. Previously, he completed his Master’s in Medical Physics at Georgia Institute of Technology.
------
The MedAI Group Exchange Sessions are a platform where we can critically examine key topics in AI and medicine, generate fresh ideas and discussion around their intersection and most importantly, learn from each other.
We will be having weekly sessions where invited speakers will give a talk presenting their work followed by an interactive discussion and Q&A. Our sessions are held every Thursday from 1pm-2pm PST.
To get notifications about upcoming sessions, please join our mailing list: https://mailman.stanford.edu/mailman/...
For more details about MedAI, check out our website: https://medai.stanford.edu. You can follow us on Twitter @MedaiStanford
Organized by members of the Rubin Lab (http://rubinlab.stanford.edu)
- Nandita Bhaskhar (https://www.stanford.edu/~nanbhas)
- Siyi Tang (https://siyitang.me)
Видео MedAI Session 30: GANs in Medical Image Synthesis, Translation, and Augmentation | Jason Jeong канала Stanford MedAI
Показать
Комментарии отсутствуют
Информация о видео
Другие видео канала

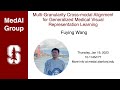
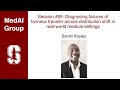
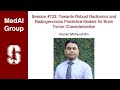

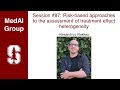
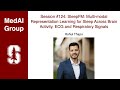
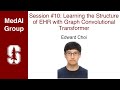
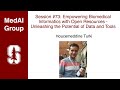


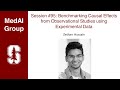
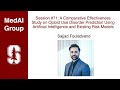
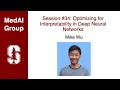
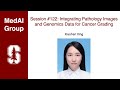
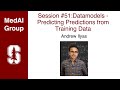
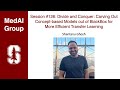
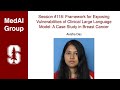

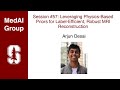