FAST '15 - Design Tradeoffs for Data Deduplication Performance in Backup Workloads
Design Tradeoffs for Data Deduplication Performance in Backup Workloads
Min Fu, Dan Feng, and Yu Hua, Huazhong University of Science and Technology; Xubin He, Virginia Commonwealth University; Zuoning Chen, National Engineering Research Center for Parallel Computer; Wen Xia and Yucheng Zhang, Huazhong University of Science and Technology; Yujuan Tan, Chongqing University
Data deduplication has become a standard component in modern backup systems. In order to understand the fundamental tradeoffs in each of its design choices (such as prefetching and sampling), we disassemble data deduplication into a large N-dimensional parameter space. Each point in the space is of various parameter settings, and performs a tradeoff among backup and restore performance, memory footprint, and storage cost. Existing and potential solutions can be considered as specific points in the space. Then, we propose a general-purpose frame- work to evaluate various deduplication solutions in the space. Given that no single solution is perfect in all metrics, our goal is to find some reasonable solutions that have sustained backup performance and perform a suitable tradeoff between deduplication ratio, memory footprints, and restore performance. Our findings from extensive experiments using real-world workloads provide a detailed guide to make efficient design decisions according to the desired tradeoff.
View the full FAST '15 Program at https://www.usenix.org/conference/fast15/technical-sessions
Видео FAST '15 - Design Tradeoffs for Data Deduplication Performance in Backup Workloads канала USENIX
Min Fu, Dan Feng, and Yu Hua, Huazhong University of Science and Technology; Xubin He, Virginia Commonwealth University; Zuoning Chen, National Engineering Research Center for Parallel Computer; Wen Xia and Yucheng Zhang, Huazhong University of Science and Technology; Yujuan Tan, Chongqing University
Data deduplication has become a standard component in modern backup systems. In order to understand the fundamental tradeoffs in each of its design choices (such as prefetching and sampling), we disassemble data deduplication into a large N-dimensional parameter space. Each point in the space is of various parameter settings, and performs a tradeoff among backup and restore performance, memory footprint, and storage cost. Existing and potential solutions can be considered as specific points in the space. Then, we propose a general-purpose frame- work to evaluate various deduplication solutions in the space. Given that no single solution is perfect in all metrics, our goal is to find some reasonable solutions that have sustained backup performance and perform a suitable tradeoff between deduplication ratio, memory footprints, and restore performance. Our findings from extensive experiments using real-world workloads provide a detailed guide to make efficient design decisions according to the desired tradeoff.
View the full FAST '15 Program at https://www.usenix.org/conference/fast15/technical-sessions
Видео FAST '15 - Design Tradeoffs for Data Deduplication Performance in Backup Workloads канала USENIX
Показать
Комментарии отсутствуют
Информация о видео
Другие видео канала
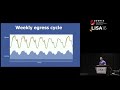
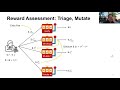
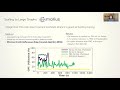
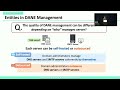
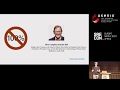
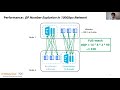

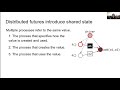
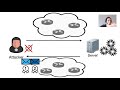
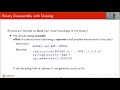
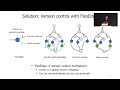
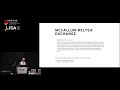
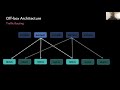
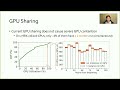
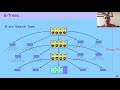
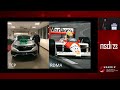
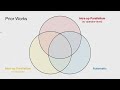
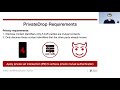
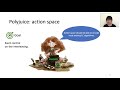
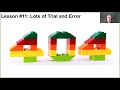
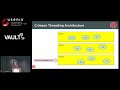