Full-Resolution Residual Networks for Semantic Segmentation in Street Scenes
Tobias Pohlen, Alexander Hermans, Markus Mathias, Bastian Leibe
Semantic image segmentation is an essential component of modern autonomous driving systems, as an accurate understanding of the surrounding scene is crucial to navigation and action planning. Current state-of-the-art approaches in semantic image segmentation rely on pre-trained networks that were initially developed for classifying images as a whole. While these networks exhibit outstanding recognition performance (i.e., what is visible?), they lack localization accuracy (i.e., where precisely is something located?). Therefore, additional processing steps have to be performed in order to obtain pixel-accurate segmentation masks at the full image resolution. To alleviate this problem we propose a novel ResNet-like architecture that exhibits strong localization and recognition performance. We combine multi-scale context with pixel-level accuracy by using two processing streams within our network: One stream carries information at the full image resolution, enabling precise adherence to segment boundaries. The other stream undergoes a sequence of pooling operations to obtain robust features for recognition. The two streams are coupled at the full image resolution using residuals. Without additional processing steps and without pre-training, our approach achieves an intersection-over-union score of 71.8% on the Cityscapes dataset.
Видео Full-Resolution Residual Networks for Semantic Segmentation in Street Scenes канала ComputerVisionFoundation Videos
Semantic image segmentation is an essential component of modern autonomous driving systems, as an accurate understanding of the surrounding scene is crucial to navigation and action planning. Current state-of-the-art approaches in semantic image segmentation rely on pre-trained networks that were initially developed for classifying images as a whole. While these networks exhibit outstanding recognition performance (i.e., what is visible?), they lack localization accuracy (i.e., where precisely is something located?). Therefore, additional processing steps have to be performed in order to obtain pixel-accurate segmentation masks at the full image resolution. To alleviate this problem we propose a novel ResNet-like architecture that exhibits strong localization and recognition performance. We combine multi-scale context with pixel-level accuracy by using two processing streams within our network: One stream carries information at the full image resolution, enabling precise adherence to segment boundaries. The other stream undergoes a sequence of pooling operations to obtain robust features for recognition. The two streams are coupled at the full image resolution using residuals. Without additional processing steps and without pre-training, our approach achieves an intersection-over-union score of 71.8% on the Cityscapes dataset.
Видео Full-Resolution Residual Networks for Semantic Segmentation in Street Scenes канала ComputerVisionFoundation Videos
Показать
Комментарии отсутствуют
Информация о видео
26 июля 2017 г. 2:14:27
00:11:28
Другие видео канала
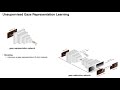

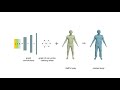
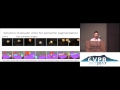
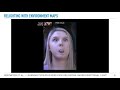
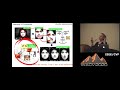
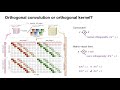
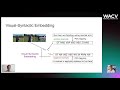

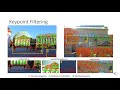

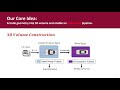
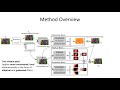
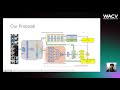
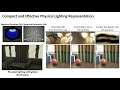
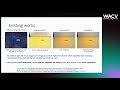
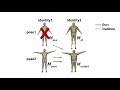
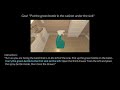
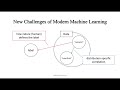
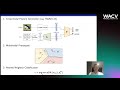
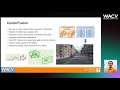