Learning Explanatory Rules from Noisy Data - Richard Evans, DeepMind
Artificial Neural Networks are powerful function approximators capable of modelling solutions to a wide variety of problems, both supervised and unsupervised. As their size and expressivity increases, so too does the variance of the model, yielding a nearly ubiquitous overfitting problem. Although mitigated by a variety of model regularisation methods, the common cure is to seek large amounts of training data—which is not necessarily easily obtained—that sufficiently approximates the data distribution of the domain we wish to test on. In contrast, logic programming methods such as Inductive Logic Programming offer an extremely data-efficient process by which models can be trained to reason on symbolic domains. However, these methods are unable to deal with the variety of domains neural networks can be applied to: they are not robust to noise in or mislabelling of inputs, and perhaps more importantly, cannot be applied to non-symbolic domains where the data is ambiguous, such as operating on raw pixels. In this paper, we propose a Differentiable Inductive Logic framework (∂ILP), which can not only solve tasks which traditional ILP systems are suited for, but shows a robustness to noise and error in the training data which ILP cannot cope with. Furthermore, as it is trained by back-propagation against a likelihood objective, it can be hybridised by connecting it with neural networks over ambiguous data in order to be applied to domains which ILP cannot address, while providing data efficiency and generalisation beyond what neural networks on their own can achieve.
The workshop was held on January 11th and 12th.
Logic has proved in the last decades a powerful tool in understanding complex systems. It is instrumental in the development of formal methods, which are mathematically based techniques obsessing on hard guarantees. Learning is a pervasive paradigm which has seen tremendous success recently. The use of statistical approaches yields practical solutions to problems which yesterday seemed out of reach. These two mindsets should not be kept apart, and many efforts have been made recently to combine the formal reasoning offered by logic and the power of learning.
The goal of this workshop is to bring together expertise from various areas to try and understand the opportunities offered by combining logic and learning.
There are 12 invited speakers and a light programme (less than 5h per day) so as to give enough time for discussions.
Видео Learning Explanatory Rules from Noisy Data - Richard Evans, DeepMind канала The Alan Turing Institute
The workshop was held on January 11th and 12th.
Logic has proved in the last decades a powerful tool in understanding complex systems. It is instrumental in the development of formal methods, which are mathematically based techniques obsessing on hard guarantees. Learning is a pervasive paradigm which has seen tremendous success recently. The use of statistical approaches yields practical solutions to problems which yesterday seemed out of reach. These two mindsets should not be kept apart, and many efforts have been made recently to combine the formal reasoning offered by logic and the power of learning.
The goal of this workshop is to bring together expertise from various areas to try and understand the opportunities offered by combining logic and learning.
There are 12 invited speakers and a light programme (less than 5h per day) so as to give enough time for discussions.
Видео Learning Explanatory Rules from Noisy Data - Richard Evans, DeepMind канала The Alan Turing Institute
Показать
Комментарии отсутствуют
Информация о видео
Другие видео канала

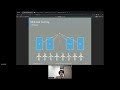
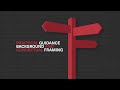
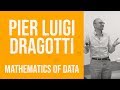
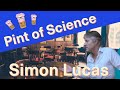
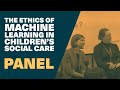
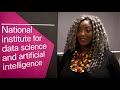
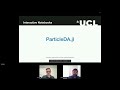
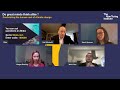
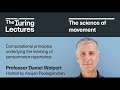
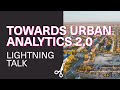


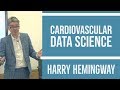
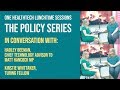
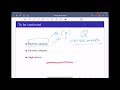
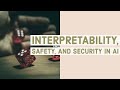
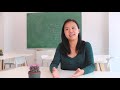

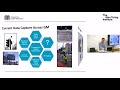
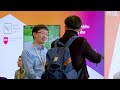