StanCon 2020. Talk 12: Ben Goodrich. Bayesian Inference without Probability Density Functions
https://mc-stan.org
The Stan Conference 2020. August 13, 2020. #stancon2020
------------------------------------------------------------
Ben Goodrich, Columbia University
Bayesian Inference without Probability Density Functions
------------------------------------------------------------
There are too many probability distributions, too many parameterizations of the same probability distribution, and too many differences in notation even for the same parameterization. Worse, the common probability distributions were derived in the pre-computer era to have explicit expressions for their central moments, without considering whether they would also be suitable for use in Stan where everything is estimated using simulations from a posterior distribution rather than analytically. Worse still, the Markov Chain Monte Carlo algorithm in Stan relies on the Probability Density Function (PDF) for a probability distribution, which is an unintuitive and poorly understood concept. All of these factors combine to make it difficult to teach Bayesian inference and difficult for Stan users to specify priors over unknown parameters.
Although the algorithm in Stan relies on logarithms of PDFs, the Stan language does not require PDFs at all, despite what its documentation might seem to suggest. This talk will demonstrate how prior beliefs about the unknown parameters can instead be conveyed through transformations via the quantile or inverse Cumulative Distribution Function of a probability distribution. Not only does this eliminate the need for PDFs, it allows Stan users to express their prior beliefs through functions of quantiles (such as the median) that always exist, rather than functions of central moments (such as the mean) that may not exist or be finite and are otherwise difficult to think about because they are defined by integrals. Finally, we can use this opportunity to move toward Keelin and Powley's (2011) "quantile parameterized distributions", which are essentially probability distributions that have explicit quantile functions with several hyperparameters that are, in turn, linear functions of user-specified quantiles. Doing so allows teachers and researchers to focus on a repeatable process for specifying prior beliefs without having to consider so many probability distributions.
Documentation: https://github.com/bgoodri/StanCon2020
Видео StanCon 2020. Talk 12: Ben Goodrich. Bayesian Inference without Probability Density Functions канала Stan
The Stan Conference 2020. August 13, 2020. #stancon2020
------------------------------------------------------------
Ben Goodrich, Columbia University
Bayesian Inference without Probability Density Functions
------------------------------------------------------------
There are too many probability distributions, too many parameterizations of the same probability distribution, and too many differences in notation even for the same parameterization. Worse, the common probability distributions were derived in the pre-computer era to have explicit expressions for their central moments, without considering whether they would also be suitable for use in Stan where everything is estimated using simulations from a posterior distribution rather than analytically. Worse still, the Markov Chain Monte Carlo algorithm in Stan relies on the Probability Density Function (PDF) for a probability distribution, which is an unintuitive and poorly understood concept. All of these factors combine to make it difficult to teach Bayesian inference and difficult for Stan users to specify priors over unknown parameters.
Although the algorithm in Stan relies on logarithms of PDFs, the Stan language does not require PDFs at all, despite what its documentation might seem to suggest. This talk will demonstrate how prior beliefs about the unknown parameters can instead be conveyed through transformations via the quantile or inverse Cumulative Distribution Function of a probability distribution. Not only does this eliminate the need for PDFs, it allows Stan users to express their prior beliefs through functions of quantiles (such as the median) that always exist, rather than functions of central moments (such as the mean) that may not exist or be finite and are otherwise difficult to think about because they are defined by integrals. Finally, we can use this opportunity to move toward Keelin and Powley's (2011) "quantile parameterized distributions", which are essentially probability distributions that have explicit quantile functions with several hyperparameters that are, in turn, linear functions of user-specified quantiles. Doing so allows teachers and researchers to focus on a repeatable process for specifying prior beliefs without having to consider so many probability distributions.
Documentation: https://github.com/bgoodri/StanCon2020
Видео StanCon 2020. Talk 12: Ben Goodrich. Bayesian Inference without Probability Density Functions канала Stan
Показать
Комментарии отсутствуют
Информация о видео
Другие видео канала
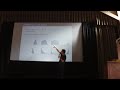
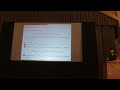

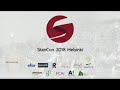
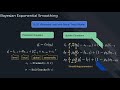
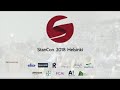
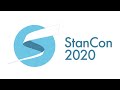
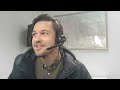
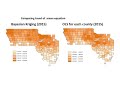
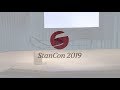

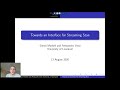
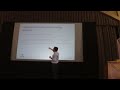

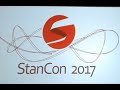
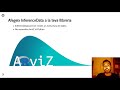
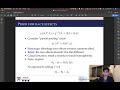
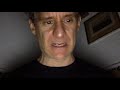
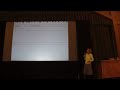

