From Parity to Preference-based Notions of Fairness in Classification
Author:
Muhammad Bilal Zafar, Max Planck Institute for Software Systems, Max Planck Institute
Abstract:
Many notions of fairness in data-driven decision making are inspired by the concept of discrimination in social sciences and law, and focus on ensuring parity (equality) in treatment or outcomes for different social groups. In this paper, we propose preference-based notions of fairness with the goals of avoiding potential ‘reverse-discrimination’ and enabling high decision accuracy. We introduce tractable proxies to design convex boundary-based classifiers that satisfy these new notions of fairness and show on the ProPublica COMPAS dataset that these notions allow for greater decision accuracy than parity-based fairness.
More on http://www.kdd.org/kdd2017/
KDD2017 Conference is published on http://videolectures.net/
Видео From Parity to Preference-based Notions of Fairness in Classification канала KDD2017 video
Muhammad Bilal Zafar, Max Planck Institute for Software Systems, Max Planck Institute
Abstract:
Many notions of fairness in data-driven decision making are inspired by the concept of discrimination in social sciences and law, and focus on ensuring parity (equality) in treatment or outcomes for different social groups. In this paper, we propose preference-based notions of fairness with the goals of avoiding potential ‘reverse-discrimination’ and enabling high decision accuracy. We introduce tractable proxies to design convex boundary-based classifiers that satisfy these new notions of fairness and show on the ProPublica COMPAS dataset that these notions allow for greater decision accuracy than parity-based fairness.
More on http://www.kdd.org/kdd2017/
KDD2017 Conference is published on http://videolectures.net/
Видео From Parity to Preference-based Notions of Fairness in Classification канала KDD2017 video
Показать
Комментарии отсутствуют
Информация о видео
Другие видео канала
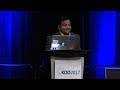
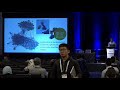
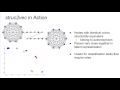
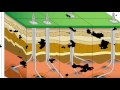
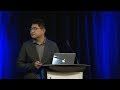

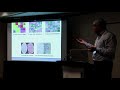
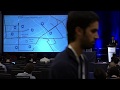

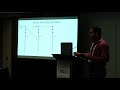
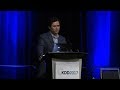
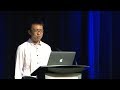
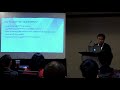
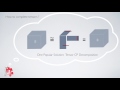
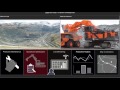
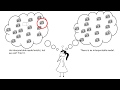
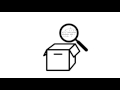
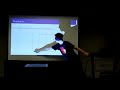

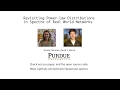
