Symbols and Rules with Deep Learning - Ellie Pavlick | Stanford MLSys #54
Episode 54 of the Stanford MLSys Seminar Series!
Implementing Symbols and Rules with Neural Networks
Speaker: Ellie Pavlick
Abstract:
Many aspects of human language and reasoning are well explained in terms of symbols and rules. However, state-of-the-art computational models are based on large neural networks which lack explicit symbolic representations of the type frequently used in cognitive theories. One response has been the development of neuro-symbolic models which introduce explicit representations of symbols into neural network architectures or loss functions. In terms of Marr's levels of analysis, such approaches achieve symbolic reasoning at the computational level ("what the system does and why") by introducing symbols and rules at the implementation and algorithmic levels. In this talk, I will consider an alternative: can neural networks (without any explicit symbolic components) nonetheless implement symbolic reasoning at the computational level? I will describe several diagnostic tests of "symbolic" and "rule-governed" behavior and use these tests to analyze neural models of visual and language processing. Our results show that on many counts, neural models appear to encode symbol-like concepts (e.g., conceptual representations that are abstract, systematic, and modular), but not perfectly so. Analysis of the failure cases reveals that future work is needed on methodological tools for analyzing neural networks, as well as refinement of models of hybrid neuro-symbolic reasoning in humans, in order to determine whether neural networks' deviations from the symbolic paradigm are a feature or a bug.
Bio:
Ellie Pavlick is an Assistant Professor of Computer Science at Brown University, where she leads the Language Understanding and Representation (LUNAR) Lab, and a Research Scientist at Google. Her research focuses on building computational models of language that are inspired by and/or informative of language processing in humans. Currently, her lab is investigating the inner-workings of neural networks in order to "reverse engineer" the conceptual structures and reasoning strategies that these models use, as well as exploring the role of grounded (non-linguistic) signals for word and concept learning. Ellie's work is supported by DARPA, IARPA, NSF, and Google.
--
0:00 Presentation
33:17 Discussion
Stanford MLSys Seminar hosts: Dan Fu, Karan Goel, Fiodar Kazhamiaka, and Piero Molino
Executive Producers: Matei Zaharia, Chris Ré
Twitter:
https://twitter.com/realDanFu
https://twitter.com/krandiash
https://twitter.com/w4nderlus7
--
Check out our website for the schedule: http://mlsys.stanford.edu
Join our mailing list to get weekly updates: https://groups.google.com/forum/#!forum/stanford-mlsys-seminars/join
#machinelearning #ai #artificialintelligence #systems #mlsys #computerscience #stanford #brown #lunarlab #google #symbols #rules #deeplearning
Видео Symbols and Rules with Deep Learning - Ellie Pavlick | Stanford MLSys #54 канала Stanford MLSys Seminars
Implementing Symbols and Rules with Neural Networks
Speaker: Ellie Pavlick
Abstract:
Many aspects of human language and reasoning are well explained in terms of symbols and rules. However, state-of-the-art computational models are based on large neural networks which lack explicit symbolic representations of the type frequently used in cognitive theories. One response has been the development of neuro-symbolic models which introduce explicit representations of symbols into neural network architectures or loss functions. In terms of Marr's levels of analysis, such approaches achieve symbolic reasoning at the computational level ("what the system does and why") by introducing symbols and rules at the implementation and algorithmic levels. In this talk, I will consider an alternative: can neural networks (without any explicit symbolic components) nonetheless implement symbolic reasoning at the computational level? I will describe several diagnostic tests of "symbolic" and "rule-governed" behavior and use these tests to analyze neural models of visual and language processing. Our results show that on many counts, neural models appear to encode symbol-like concepts (e.g., conceptual representations that are abstract, systematic, and modular), but not perfectly so. Analysis of the failure cases reveals that future work is needed on methodological tools for analyzing neural networks, as well as refinement of models of hybrid neuro-symbolic reasoning in humans, in order to determine whether neural networks' deviations from the symbolic paradigm are a feature or a bug.
Bio:
Ellie Pavlick is an Assistant Professor of Computer Science at Brown University, where she leads the Language Understanding and Representation (LUNAR) Lab, and a Research Scientist at Google. Her research focuses on building computational models of language that are inspired by and/or informative of language processing in humans. Currently, her lab is investigating the inner-workings of neural networks in order to "reverse engineer" the conceptual structures and reasoning strategies that these models use, as well as exploring the role of grounded (non-linguistic) signals for word and concept learning. Ellie's work is supported by DARPA, IARPA, NSF, and Google.
--
0:00 Presentation
33:17 Discussion
Stanford MLSys Seminar hosts: Dan Fu, Karan Goel, Fiodar Kazhamiaka, and Piero Molino
Executive Producers: Matei Zaharia, Chris Ré
Twitter:
https://twitter.com/realDanFu
https://twitter.com/krandiash
https://twitter.com/w4nderlus7
--
Check out our website for the schedule: http://mlsys.stanford.edu
Join our mailing list to get weekly updates: https://groups.google.com/forum/#!forum/stanford-mlsys-seminars/join
#machinelearning #ai #artificialintelligence #systems #mlsys #computerscience #stanford #brown #lunarlab #google #symbols #rules #deeplearning
Видео Symbols and Rules with Deep Learning - Ellie Pavlick | Stanford MLSys #54 канала Stanford MLSys Seminars
Показать
Комментарии отсутствуют
Информация о видео
Другие видео канала
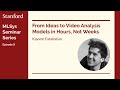
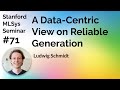

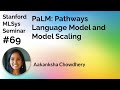
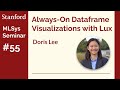
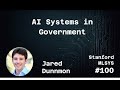
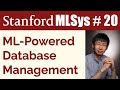
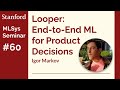
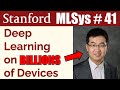

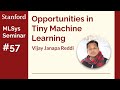
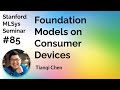
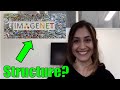

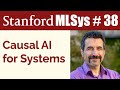
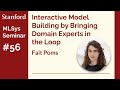
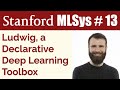
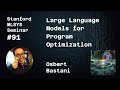
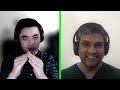
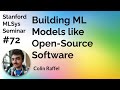
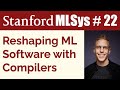