Deep image reconstruction from human brain activity (Paper Explained)
Can you peek into people's brains? Reading human thoughts is a long-standing dream of the AI field. This paper reads fMRI signals from a person and then reconstructs what that person's eyes currently see. This is achieved by translating the fMRI signal to features of a Deep Neural Network and then iteratively optimizing the input of the network to match those features. The results are impressive.
OUTLINE:
0:00 - Overview
1:35 - Pipeline
4:00 - Training
5:20 - Image Reconstruction
7:00 - Deep Generator Network
8:15 - Results
Paper: https://journals.plos.org/ploscompbiol/article?id=10.1371/journal.pcbi.1006633
My Video on OpenAI Microscope (what I called Atlas): https://youtu.be/Ok44otx90D4
Abstract:
The mental contents of perception and imagery are thought to be encoded in hierarchical representations in the brain, but previous attempts to visualize perceptual contents have failed to capitalize on multiple levels of the hierarchy, leaving it challenging to reconstruct internal imagery. Recent work showed that visual cortical activity measured by functional magnetic resonance imaging (fMRI) can be decoded (translated) into the hierarchical features of a pre-trained deep neural network (DNN) for the same input image, providing a way to make use of the information from hierarchical visual features. Here, we present a novel image reconstruction method, in which the pixel values of an image are optimized to make its DNN features similar to those decoded from human brain activity at multiple layers. We found that our method was able to reliably produce reconstructions that resembled the viewed natural images. A natural image prior introduced by a deep generator neural network effectively rendered semantically meaningful details to the reconstructions. Human judgment of the reconstructions supported the effectiveness of combining multiple DNN layers to enhance the visual quality of generated images. While our model was solely trained with natural images, it successfully generalized to artificial shapes, indicating that our model was not simply matching to exemplars. The same analysis applied to mental imagery demonstrated rudimentary reconstructions of the subjective content. Our results suggest that our method can effectively combine hierarchical neural representations to reconstruct perceptual and subjective images, providing a new window into the internal contents of the brain.
Authors: Guohua Shen, Tomoyasu Horikawa, Kei Majima, Yukiyasu Kamitani
Links:
YouTube: https://www.youtube.com/c/yannickilcher
Twitter: https://twitter.com/ykilcher
BitChute: https://www.bitchute.com/channel/yannic-kilcher
Minds: https://www.minds.com/ykilcher
Видео Deep image reconstruction from human brain activity (Paper Explained) канала Yannic Kilcher
OUTLINE:
0:00 - Overview
1:35 - Pipeline
4:00 - Training
5:20 - Image Reconstruction
7:00 - Deep Generator Network
8:15 - Results
Paper: https://journals.plos.org/ploscompbiol/article?id=10.1371/journal.pcbi.1006633
My Video on OpenAI Microscope (what I called Atlas): https://youtu.be/Ok44otx90D4
Abstract:
The mental contents of perception and imagery are thought to be encoded in hierarchical representations in the brain, but previous attempts to visualize perceptual contents have failed to capitalize on multiple levels of the hierarchy, leaving it challenging to reconstruct internal imagery. Recent work showed that visual cortical activity measured by functional magnetic resonance imaging (fMRI) can be decoded (translated) into the hierarchical features of a pre-trained deep neural network (DNN) for the same input image, providing a way to make use of the information from hierarchical visual features. Here, we present a novel image reconstruction method, in which the pixel values of an image are optimized to make its DNN features similar to those decoded from human brain activity at multiple layers. We found that our method was able to reliably produce reconstructions that resembled the viewed natural images. A natural image prior introduced by a deep generator neural network effectively rendered semantically meaningful details to the reconstructions. Human judgment of the reconstructions supported the effectiveness of combining multiple DNN layers to enhance the visual quality of generated images. While our model was solely trained with natural images, it successfully generalized to artificial shapes, indicating that our model was not simply matching to exemplars. The same analysis applied to mental imagery demonstrated rudimentary reconstructions of the subjective content. Our results suggest that our method can effectively combine hierarchical neural representations to reconstruct perceptual and subjective images, providing a new window into the internal contents of the brain.
Authors: Guohua Shen, Tomoyasu Horikawa, Kei Majima, Yukiyasu Kamitani
Links:
YouTube: https://www.youtube.com/c/yannickilcher
Twitter: https://twitter.com/ykilcher
BitChute: https://www.bitchute.com/channel/yannic-kilcher
Minds: https://www.minds.com/ykilcher
Видео Deep image reconstruction from human brain activity (Paper Explained) канала Yannic Kilcher
Показать
Комментарии отсутствуют
Информация о видео
Другие видео канала
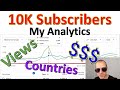
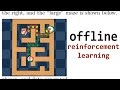
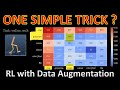
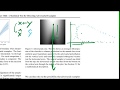
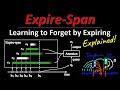
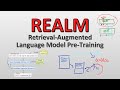
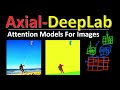
![[Classic] Playing Atari with Deep Reinforcement Learning (Paper Explained)](https://i.ytimg.com/vi/rFwQDDbYTm4/default.jpg)
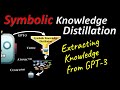
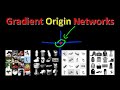
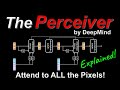
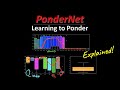
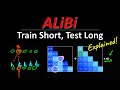
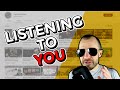
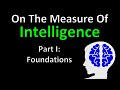
![[ML News] Uber: Deep Learning for ETA | MuZero Video Compression | Block-NeRF | EfficientNet-X](https://i.ytimg.com/vi/fEKZC9mta8w/default.jpg)
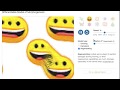
![[ML News] DeepMind's Flamingo Image-Text model | Locked-Image Tuning | Jurassic X & MRKL](https://i.ytimg.com/vi/smUHQndcmOY/default.jpg)
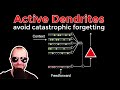
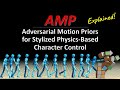
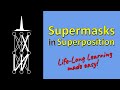