Multitask Prompted Training Enables Zero-shot Task Generalization (Explained)
Can zero-shot generalization instead be directly induced by explicit multitask learning? Watch the video to find out!
0:00 - Intro
2:14 - Prompted training format
5:52 - Measuring generalization to unseen tasks
8:45 - Held-out tasks
10:45 - The future of NLP
11:48 - Model
12:17 - Experiment results
Connect
Linkedin https://www.linkedin.com/in/xue-yong-fu-955723a6/
Twitter https://twitter.com/home
email edwindeeplearning@gmail.com
Paper
https://arxiv.org/abs/2110.08207
Code
https://github.com/bigscience-workshop/promptsource/
Abstract
Large language models have recently been shown to attain reasonable zero-shot generalization on a diverse set of tasks. It has been hypothesized that this is a consequence of implicit multitask learning in language model training. Can zero-shot generalization instead be directly induced by explicit multitask learning? To test this question at scale, we develop a system for easily mapping general natural language tasks into a human-readable prompted form. We convert a large set of supervised datasets, each with multiple prompts using varying natural language. These prompted datasets allow for benchmarking the ability of a model to perform completely unseen tasks specified in natural language. We fine-tune a pretrained encoder-decoder model on this multitask mixture covering a wide variety of tasks. The model attains strong zero-shot performance on several standard datasets, often outperforming models 16x its size. Further, our approach attains strong performance on a subset of tasks from the BIG-Bench benchmark, outperforming models 6x its size.
Видео Multitask Prompted Training Enables Zero-shot Task Generalization (Explained) канала Deep Learning Explainer
0:00 - Intro
2:14 - Prompted training format
5:52 - Measuring generalization to unseen tasks
8:45 - Held-out tasks
10:45 - The future of NLP
11:48 - Model
12:17 - Experiment results
Connect
Linkedin https://www.linkedin.com/in/xue-yong-fu-955723a6/
Twitter https://twitter.com/home
email edwindeeplearning@gmail.com
Paper
https://arxiv.org/abs/2110.08207
Code
https://github.com/bigscience-workshop/promptsource/
Abstract
Large language models have recently been shown to attain reasonable zero-shot generalization on a diverse set of tasks. It has been hypothesized that this is a consequence of implicit multitask learning in language model training. Can zero-shot generalization instead be directly induced by explicit multitask learning? To test this question at scale, we develop a system for easily mapping general natural language tasks into a human-readable prompted form. We convert a large set of supervised datasets, each with multiple prompts using varying natural language. These prompted datasets allow for benchmarking the ability of a model to perform completely unseen tasks specified in natural language. We fine-tune a pretrained encoder-decoder model on this multitask mixture covering a wide variety of tasks. The model attains strong zero-shot performance on several standard datasets, often outperforming models 16x its size. Further, our approach attains strong performance on a subset of tasks from the BIG-Bench benchmark, outperforming models 6x its size.
Видео Multitask Prompted Training Enables Zero-shot Task Generalization (Explained) канала Deep Learning Explainer
Показать
Комментарии отсутствуют
Информация о видео
25 октября 2021 г. 4:05:29
00:16:36
Другие видео канала
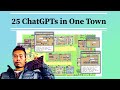
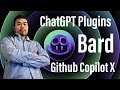
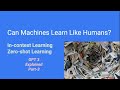
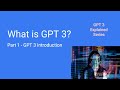
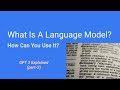

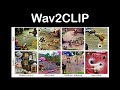
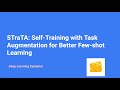
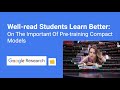

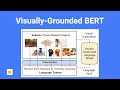
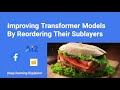
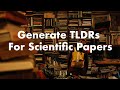
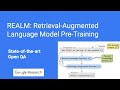
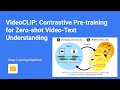
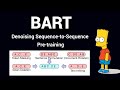
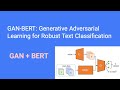
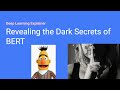
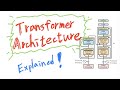
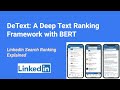