Dan Foreman-Mackey -TimeSeriesAnalysis Using Gaussian Processes in Python & the Search for Earth 2.0
View slide presentation here:
https://speakerdeck.com/dfm/pydata-time-series-analysis-gps-and-exoplanets
PyData NYC 2014
Thousands of planets outside the solar system have been discovered using time series data from NASA's Kepler mission but not a single one is a true Earth twin. I'm working to discover Earth 2.0 using an open dataset from NASA and custom-built, high-performance tools in Python. I will sketch the problem and introduce the resulting Python module (called George; http://dfm.io/george) for doing time series analysis with Gaussian Processes (GPs). The core algorithm (developed in collaboration with applied mathematicians at NYU) allows this code to compute GPs on general large datasets that were previously intractable. This method isn't just applicable in astronomy so I'll demonstrate how this package can be incorporated into the standard scientific Python stack and compare it to other GP implementations. 00:00 Welcome!
00:10 Help us add time stamps or captions to this video! See the description for details.
Want to help add timestamps to our YouTube videos to help with discoverability? Find out more here: https://github.com/numfocus/YouTubeVideoTimestamps
Видео Dan Foreman-Mackey -TimeSeriesAnalysis Using Gaussian Processes in Python & the Search for Earth 2.0 канала PyData
https://speakerdeck.com/dfm/pydata-time-series-analysis-gps-and-exoplanets
PyData NYC 2014
Thousands of planets outside the solar system have been discovered using time series data from NASA's Kepler mission but not a single one is a true Earth twin. I'm working to discover Earth 2.0 using an open dataset from NASA and custom-built, high-performance tools in Python. I will sketch the problem and introduce the resulting Python module (called George; http://dfm.io/george) for doing time series analysis with Gaussian Processes (GPs). The core algorithm (developed in collaboration with applied mathematicians at NYU) allows this code to compute GPs on general large datasets that were previously intractable. This method isn't just applicable in astronomy so I'll demonstrate how this package can be incorporated into the standard scientific Python stack and compare it to other GP implementations. 00:00 Welcome!
00:10 Help us add time stamps or captions to this video! See the description for details.
Want to help add timestamps to our YouTube videos to help with discoverability? Find out more here: https://github.com/numfocus/YouTubeVideoTimestamps
Видео Dan Foreman-Mackey -TimeSeriesAnalysis Using Gaussian Processes in Python & the Search for Earth 2.0 канала PyData
Показать
Комментарии отсутствуют
Информация о видео
Другие видео канала
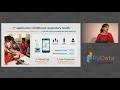
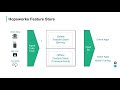
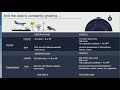
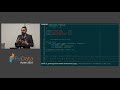
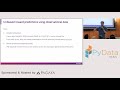
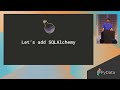
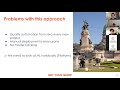
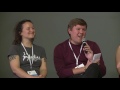
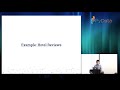
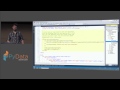
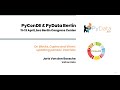

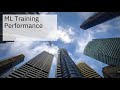

![Allen Downey: Bayesian Decision Analysis [Tutorial] | PyData Global 2022](https://i.ytimg.com/vi/fsdbneHgi58/default.jpg)
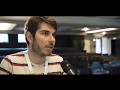
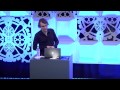


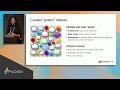
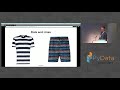