IML17: Grid vs random search for hyperparameter tuning?
In this video I review the benefits of random search over grid search when tuning the hyperparameters of a machine learning model.
I specifically discuss the paper by Bergstra and Bengio, called Random Search for Hyper-Parameter Optimization.
J. Bergstra and Y. Bengio. `Random search for hyper-parameter optimization'. In:
Journal of Machine Learning Research 13 (2012), pp. 281-305.
Видео IML17: Grid vs random search for hyperparameter tuning? канала Bevan Smith 2
I specifically discuss the paper by Bergstra and Bengio, called Random Search for Hyper-Parameter Optimization.
J. Bergstra and Y. Bengio. `Random search for hyper-parameter optimization'. In:
Journal of Machine Learning Research 13 (2012), pp. 281-305.
Видео IML17: Grid vs random search for hyperparameter tuning? канала Bevan Smith 2
Показать
Комментарии отсутствуют
Информация о видео
Другие видео канала
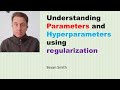
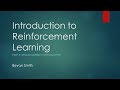
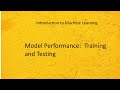
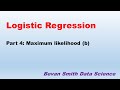
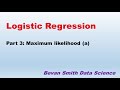


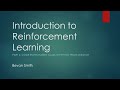
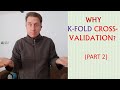
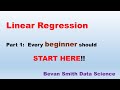
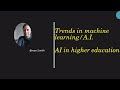

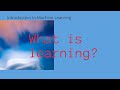
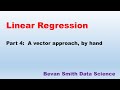
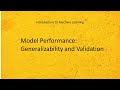
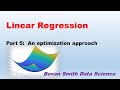
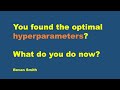

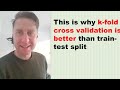
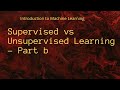
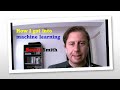