Vitaly Shmatikov, How to Salvage Federated Learning
Virtual Seminar, Interest Group on Privacy and Machine Learning
Видео Vitaly Shmatikov, How to Salvage Federated Learning канала Privacy and Security in ML Interest Group
Видео Vitaly Shmatikov, How to Salvage Federated Learning канала Privacy and Security in ML Interest Group
Показать
Комментарии отсутствуют
Информация о видео
22 января 2021 г. 20:07:40
00:42:46
Другие видео канала
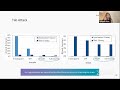

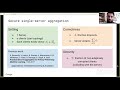
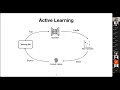
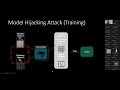

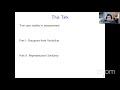
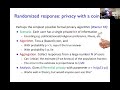
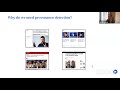
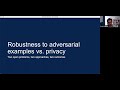
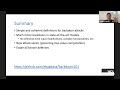

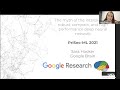
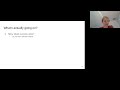
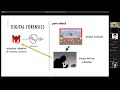
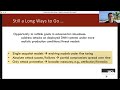
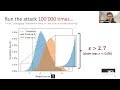
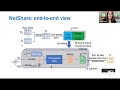
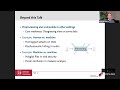
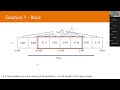