Getting the best results from your ML models | Youden's Index
In this video, we dive into Youden's Index, a valuable metric in classification problems. But first, let's discuss the basic concept of classification, where a class is predicted by comparing the predicted probabilities with a threshold, typically set at 0.5 in many sklearn algorithms. 📊
Pre-requisite videos:
Confusion Matrix - https://youtu.be/b83gwi7IyK4
ROC Curve - https://youtu.be/ExJCi6aj1cM
Imagine a scenario where we have a binary classification problem. On the ROC curve, we have the True Positive Rate (TPR) on the y-axis and the False Positive Rate (FPR) on the x-axis. By varying the threshold, we can plot different points on this curve, each representing a different trade-off between TPR and FPR. 🔄
Now, let's consider the extreme cases. When the model is perfect i.e. FP and FN both are zeros, our model is perfectly accurate and Youden's index is +1. Conversely, when the TP and TN both are zeros, our model is perfectly erratic. These extremes help us understand the behavior of the J statistic. 📈
Maximizing the J statistic helps us find the optimal threshold. This can be visualized as the height of the difference between the threshold and the line of the random classifier on the ROC curve. By finding this optimal threshold, we can improve the performance of our classification model. 🔝
Join us in this video as we uncover the intricacies of Youden's Index and its role in enhancing the effectiveness of classification models. Don't forget to like, share, and subscribe for more insightful content! 🚀
Видео Getting the best results from your ML models | Youden's Index канала Six Sigma Pro SMART
Pre-requisite videos:
Confusion Matrix - https://youtu.be/b83gwi7IyK4
ROC Curve - https://youtu.be/ExJCi6aj1cM
Imagine a scenario where we have a binary classification problem. On the ROC curve, we have the True Positive Rate (TPR) on the y-axis and the False Positive Rate (FPR) on the x-axis. By varying the threshold, we can plot different points on this curve, each representing a different trade-off between TPR and FPR. 🔄
Now, let's consider the extreme cases. When the model is perfect i.e. FP and FN both are zeros, our model is perfectly accurate and Youden's index is +1. Conversely, when the TP and TN both are zeros, our model is perfectly erratic. These extremes help us understand the behavior of the J statistic. 📈
Maximizing the J statistic helps us find the optimal threshold. This can be visualized as the height of the difference between the threshold and the line of the random classifier on the ROC curve. By finding this optimal threshold, we can improve the performance of our classification model. 🔝
Join us in this video as we uncover the intricacies of Youden's Index and its role in enhancing the effectiveness of classification models. Don't forget to like, share, and subscribe for more insightful content! 🚀
Видео Getting the best results from your ML models | Youden's Index канала Six Sigma Pro SMART
Показать
Комментарии отсутствуют
Информация о видео
Другие видео канала
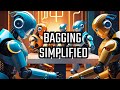
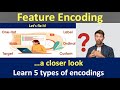
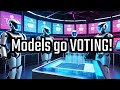
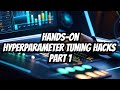

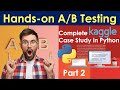

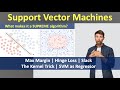
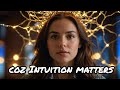
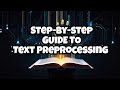
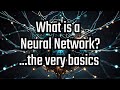
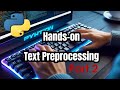

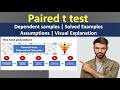

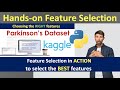
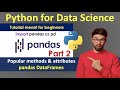
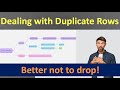
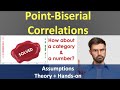
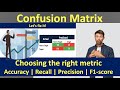