Football Analytics Using Hierarchical Bayesian Models in PyMC | PyData Global 2021
Football Analytics Using Hierarchical Bayesian Models in PyMC
Speaker: Meenal Jhajharia
Summary
We deconstruct the basics of Bayesian hierarchical modeling and then use Fantasy Premier League statistics to construct a model in PyMC that tries to guess the remaining season's results. Along the way, we talk about crucial modeling choices,
Description
Sports analytics is a growing arena for data analysts nowadays, and obviously, the Bayesians are coming for it! We will take a good look at data and figure out what matters for predicting points. No worries if you are not a football fan: we will take a quick look at the rules. In this talk we demystify partial pooling, priors, and other fancy terms statisticians use. Once we have some imperfect but somewhat functional predictive outcomes, we try to understand our results and takeaways. If you want to get started with (or build a bit on) Bayesian statistics or if you are just excited about football, this will be fun.
Meenal Jhajharia's Bio
Meenal is a 19 year old CS and Math Undergrad. She spent this summer extending time series modeling capabilities in PyMC under the Google Summer of Code, she is also a core contributor of the PyMC project
GitHub: https://github.com/mjhajharia/
Twitter: https://twitter.com/meenaljhajharia/
LinkedIn: https://www.linkedin.com/in/meenal-jhajharia-a95185190/
Website: https://mjhajharia.com//
PyData Global 2021
Website: https://pydata.org/global2021/
LinkedIn: https://www.linkedin.com/company/pydata-global
Twitter: https://twitter.com/PyData
www.pydata.org
PyData is an educational program of NumFOCUS, a 501(c)3 non-profit organization in the United States. PyData provides a forum for the international community of users and developers of data analysis tools to share ideas and learn from each other. The global PyData network promotes discussion of best practices, new approaches, and emerging technologies for data management, processing, analytics, and visualization. PyData communities approach data science using many languages, including (but not limited to) Python, Julia, and R.
PyData conferences aim to be accessible and community-driven, with novice to advanced level presentations. PyData tutorials and talks bring attendees the latest project features along with cutting-edge use cases.
00:00 Welcome!
00:10 Help us add time stamps or captions to this video! See the description for details.
Want to help add timestamps to our YouTube videos to help with discoverability? Find out more here: https://github.com/numfocus/YouTubeVideoTimestamps
Видео Football Analytics Using Hierarchical Bayesian Models in PyMC | PyData Global 2021 канала PyData
Speaker: Meenal Jhajharia
Summary
We deconstruct the basics of Bayesian hierarchical modeling and then use Fantasy Premier League statistics to construct a model in PyMC that tries to guess the remaining season's results. Along the way, we talk about crucial modeling choices,
Description
Sports analytics is a growing arena for data analysts nowadays, and obviously, the Bayesians are coming for it! We will take a good look at data and figure out what matters for predicting points. No worries if you are not a football fan: we will take a quick look at the rules. In this talk we demystify partial pooling, priors, and other fancy terms statisticians use. Once we have some imperfect but somewhat functional predictive outcomes, we try to understand our results and takeaways. If you want to get started with (or build a bit on) Bayesian statistics or if you are just excited about football, this will be fun.
Meenal Jhajharia's Bio
Meenal is a 19 year old CS and Math Undergrad. She spent this summer extending time series modeling capabilities in PyMC under the Google Summer of Code, she is also a core contributor of the PyMC project
GitHub: https://github.com/mjhajharia/
Twitter: https://twitter.com/meenaljhajharia/
LinkedIn: https://www.linkedin.com/in/meenal-jhajharia-a95185190/
Website: https://mjhajharia.com//
PyData Global 2021
Website: https://pydata.org/global2021/
LinkedIn: https://www.linkedin.com/company/pydata-global
Twitter: https://twitter.com/PyData
www.pydata.org
PyData is an educational program of NumFOCUS, a 501(c)3 non-profit organization in the United States. PyData provides a forum for the international community of users and developers of data analysis tools to share ideas and learn from each other. The global PyData network promotes discussion of best practices, new approaches, and emerging technologies for data management, processing, analytics, and visualization. PyData communities approach data science using many languages, including (but not limited to) Python, Julia, and R.
PyData conferences aim to be accessible and community-driven, with novice to advanced level presentations. PyData tutorials and talks bring attendees the latest project features along with cutting-edge use cases.
00:00 Welcome!
00:10 Help us add time stamps or captions to this video! See the description for details.
Want to help add timestamps to our YouTube videos to help with discoverability? Find out more here: https://github.com/numfocus/YouTubeVideoTimestamps
Видео Football Analytics Using Hierarchical Bayesian Models in PyMC | PyData Global 2021 канала PyData
Показать
Комментарии отсутствуют
Информация о видео
Другие видео канала
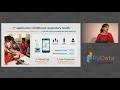
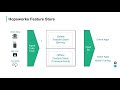
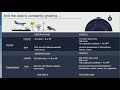
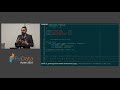
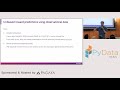
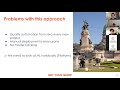
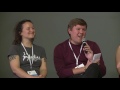
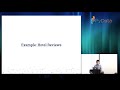
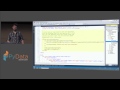

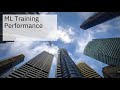

![Allen Downey: Bayesian Decision Analysis [Tutorial] | PyData Global 2022](https://i.ytimg.com/vi/fsdbneHgi58/default.jpg)
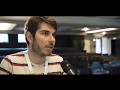
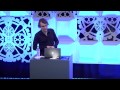


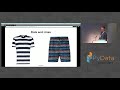
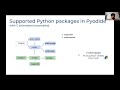
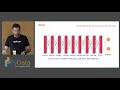
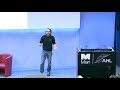