Mastering Hyperparameter Tuning in AI Models
Unlock the full potential of your AI models with our comprehensive guide on Mastering Hyperparameter Tuning. In this video, we delve into various techniques to optimize AI models, from basic concepts to advanced strategies, enhancing both performance and efficiency.
We begin with an introduction to hyperparameters, emphasizing their critical role in AI models and their impact on performance. Learn about different tuning methods and set clear learning objectives.
Explore Grid Search with a detailed explanation of its implementation, advantages, and limitations. Discover how Random Search can offer efficiency in high-dimensional spaces, comparing its pros and cons with Grid Search.
Get acquainted with Bayesian Optimization, understanding surrogate models and acquisition functions, and see how it provides an efficient tuning framework. Hyperband is introduced as a method for optimizing resource allocation, with strategies for implementation and successful examples.
We also cover practical tools for hyperparameter tuning, highlighting features of Optuna and Hyperopt, and their integration with popular frameworks to help you choose the right tool for your needs.
Address common challenges in hyperparameter tuning, offering solutions to overcome obstacles, balance performance and resources, and avoid overfitting.
Learn from real-world examples of successful hyperparameter tuning applications, exploring case studies and industry impacts.
Conclude with a recap of tuning techniques, emphasizing the importance of continual learning and experimentation. Gain insights into future trends in hyperparameter tuning and take action towards mastering these essential skills.
Видео Mastering Hyperparameter Tuning in AI Models канала NextGen AI & Tech Explorer
We begin with an introduction to hyperparameters, emphasizing their critical role in AI models and their impact on performance. Learn about different tuning methods and set clear learning objectives.
Explore Grid Search with a detailed explanation of its implementation, advantages, and limitations. Discover how Random Search can offer efficiency in high-dimensional spaces, comparing its pros and cons with Grid Search.
Get acquainted with Bayesian Optimization, understanding surrogate models and acquisition functions, and see how it provides an efficient tuning framework. Hyperband is introduced as a method for optimizing resource allocation, with strategies for implementation and successful examples.
We also cover practical tools for hyperparameter tuning, highlighting features of Optuna and Hyperopt, and their integration with popular frameworks to help you choose the right tool for your needs.
Address common challenges in hyperparameter tuning, offering solutions to overcome obstacles, balance performance and resources, and avoid overfitting.
Learn from real-world examples of successful hyperparameter tuning applications, exploring case studies and industry impacts.
Conclude with a recap of tuning techniques, emphasizing the importance of continual learning and experimentation. Gain insights into future trends in hyperparameter tuning and take action towards mastering these essential skills.
Видео Mastering Hyperparameter Tuning in AI Models канала NextGen AI & Tech Explorer
Комментарии отсутствуют
Информация о видео
14 марта 2025 г. 18:24:38
00:07:27
Другие видео канала
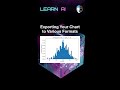



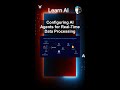
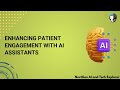

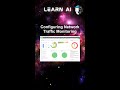


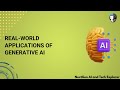
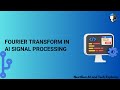
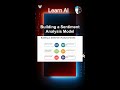
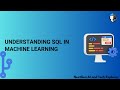
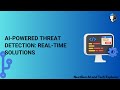
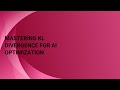
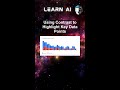
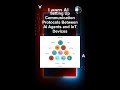
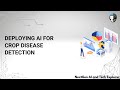
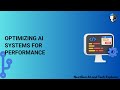
