Solving Inverse Problems with Deep Learning by Lexing Ying
Lexing Ying (Stanford), Solving Inverse Problems with Deep Learning.
http://web.stanford.edu/~lexing/
Abstract
This talk is about some recent progress on solving inverse problems using deep learning. Compared to traditional machine learning problems, inverse problems are often limited by the size of the training data set. We show how to overcome this issue by incorporating mathematical analysis and physics into the design of neural network architectures. We first describe neural network representations of pseudodifferential operators and Fourier integral operators. We then continue to discuss applications including electric impedance tomography, optical tomography, inverse acoustic/EM scattering, seismic imaging, and travel-time tomography.
AAAI 2020 Spring Symposium on Combining Artificial Intelligence and Machine Learning with Physics Sciences, March 23-25, 2020 (https://sites.google.com/view/aaai-mlps)
Papers: https://sites.google.com/view/aaai-mlps/proceedings
Slides: https://sites.google.com/view/aaai-mlps/program
Видео Solving Inverse Problems with Deep Learning by Lexing Ying канала MLPS - Combining AI and ML with Physics Sciences
http://web.stanford.edu/~lexing/
Abstract
This talk is about some recent progress on solving inverse problems using deep learning. Compared to traditional machine learning problems, inverse problems are often limited by the size of the training data set. We show how to overcome this issue by incorporating mathematical analysis and physics into the design of neural network architectures. We first describe neural network representations of pseudodifferential operators and Fourier integral operators. We then continue to discuss applications including electric impedance tomography, optical tomography, inverse acoustic/EM scattering, seismic imaging, and travel-time tomography.
AAAI 2020 Spring Symposium on Combining Artificial Intelligence and Machine Learning with Physics Sciences, March 23-25, 2020 (https://sites.google.com/view/aaai-mlps)
Papers: https://sites.google.com/view/aaai-mlps/proceedings
Slides: https://sites.google.com/view/aaai-mlps/program
Видео Solving Inverse Problems with Deep Learning by Lexing Ying канала MLPS - Combining AI and ML with Physics Sciences
Показать
Комментарии отсутствуют
Информация о видео
30 марта 2020 г. 20:43:13
00:45:26
Другие видео канала
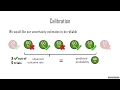
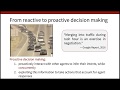
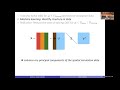
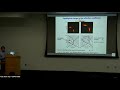
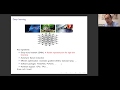
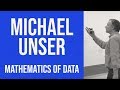
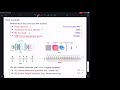
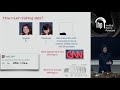
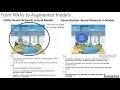
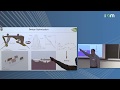
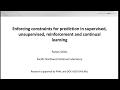
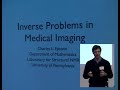
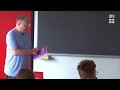
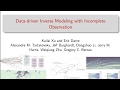

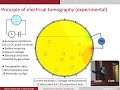
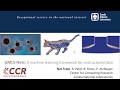
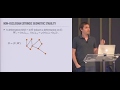
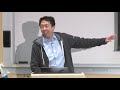
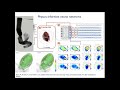