Machine Learning for Healthcare, part 2 - Mihaela van der Schaar - MLSS 2020, Tübingen
Table of Contents (powered by https://videoken.com)
0:00:00 Machine Learning for Healthcare
0:00:45 http://www.vanderschaar-lab.com
0:01:24 Part 3: Dynamic Forecasting
0:01:33 One Success Story: Forecast ICU
0:02:36 Results (at UCLA Ronald Reagan Hospital): Precision-Recall (TPR vs PPV)
0:04:10 Goal: Design General Framework for Dynamic Forecast in in Various Settings (Office, Clinic, Hospital)
0:05:48 Dynamic Forecasting: A Challenge Problem
0:07:38 Current Disease Progression Models
0:08:34 Hidden Markov Models (HMMs)
0:10:55 Models for Health and Disease Trajectories - Requirement
0:12:08 HASMM: Hidden Absorbing Semi-Markov
0:13:47 HASMM State-space
0:14:54 Informative Observation Times and Censoring
0:16:12 Informative Observation Times
0:17:24 HASMM-A versatile model!
0:19:39 Learning HASMM Models
0:20:24 Inference
0:21:01 Inference: Formulation
0:21:46 Inference: Challenges
0:22:41 Generality of the HASMM
0:23:36 Markov models?
0:24:44 Two Central Goals of Longitudinal Models
0:25:27 Accuracy-interpretability Trade-off
0:25:49 Deep Learning Models?
0:27:11 Different Forms of Interpretability
0:28:34 Attentive state space models
0:29:20 Attentional State-Space!
0:29:46 Going Beyond Markov
0:31:13 Overcomes shortcoming of Markov Models
0:32:09 Generality of the Attentive State-Space Models
0:33:00 ASSM: A General, Versatile and Clinically Actionable Models
0:35:19 Morbidity networks: Personalized and dynamic
0:36:49 Learning Dynamic and Personalized Comorbidity Network Event Data using Deep Diffusion Processes
0:38:12 A tool for decision support and discovery
0:39:01 Part 4: Personalized Monitoring and Screening
0:40:36 Personalized Screening/Monitoring: Who to Screen? When to Screen? What to Screen?
0:41:48 Deep Sensing: Active Sensing Using Multi-directional Recurrent Neural Networks
0:44:35 Deep Sensing Architecture
0:46:58 Temporal Phenotyping using Deep Predicting clustering of Disease Progression [Lee, vdS, ICML 2020]
0:50:10 Part 5: Personalized medicine needs to go beyond risk predictions-Individualized Treatment Recommendations
0:50:40 RCTs do not support Personalized Medicine
0:51:55 Delivering Personalized (Individualized) Treatments
0:52:17 Goal: Learning Individualized Treatment/Causal Effect from Observational Data
0:52:58 Our Goal
0:53:12 Potential outcomes framework
0:54:33 Assumptions
0:55:38 The learning problem
0:56:05 Beyond supervised learning...
0:56:24 Causal modeling not equal to predictive modeling
0:57:29 Very active area of research - Many works on treatment effects
0:57:36 Modeling ITEs: Key questions
0:58:01 ITE models: A classification
0:59:22 Many ITE estimation models!
0:59:59 A first theory for causal inference - individualized treatment
1:00:40 Bayesian non parametric ITE estimation
1:02:42 Minimax Rate for ITE Estimation
1:05:19 Should we care about selection bias?
1:06:29 Theory guides model design
1:06:42 Multi-task Gaussian processes
1:07:06 ITE using Non-stationary Gaussian Processes
1:08:21 Multiple Treatments: GANITE
1:09:36 How to select the best model?
1:10:38 Does it matter?
1:11:21 When to treat? How to treat? When to stop?
1:11:48 Counterfactual Recurrent Network
1:12:43 Q&A
1:20:11 Part 6?: Integration: Can we have a unified end-to-end pipeline?
1:20:16 Clairvoyance: A unified, end-to-end pipeline for clinical decision
1:21:13 Part 7: How do we get data? Synthetic Data
1:21:47 Why do we care? We need data!
1:22:11 What can be done?
1:22:34 Why differential privacy?
1:23:18 NeurIPS 2020: Hide-and-Seek Privacy Challenge
1:24:52 A Revolution in Healthcare is taking place
1:25:36 Machine Learning & Healthcare: Vision
1:26:17 Acknowledgements
1:26:54 Q&A
Видео Machine Learning for Healthcare, part 2 - Mihaela van der Schaar - MLSS 2020, Tübingen канала virtual mlss2020
0:00:00 Machine Learning for Healthcare
0:00:45 http://www.vanderschaar-lab.com
0:01:24 Part 3: Dynamic Forecasting
0:01:33 One Success Story: Forecast ICU
0:02:36 Results (at UCLA Ronald Reagan Hospital): Precision-Recall (TPR vs PPV)
0:04:10 Goal: Design General Framework for Dynamic Forecast in in Various Settings (Office, Clinic, Hospital)
0:05:48 Dynamic Forecasting: A Challenge Problem
0:07:38 Current Disease Progression Models
0:08:34 Hidden Markov Models (HMMs)
0:10:55 Models for Health and Disease Trajectories - Requirement
0:12:08 HASMM: Hidden Absorbing Semi-Markov
0:13:47 HASMM State-space
0:14:54 Informative Observation Times and Censoring
0:16:12 Informative Observation Times
0:17:24 HASMM-A versatile model!
0:19:39 Learning HASMM Models
0:20:24 Inference
0:21:01 Inference: Formulation
0:21:46 Inference: Challenges
0:22:41 Generality of the HASMM
0:23:36 Markov models?
0:24:44 Two Central Goals of Longitudinal Models
0:25:27 Accuracy-interpretability Trade-off
0:25:49 Deep Learning Models?
0:27:11 Different Forms of Interpretability
0:28:34 Attentive state space models
0:29:20 Attentional State-Space!
0:29:46 Going Beyond Markov
0:31:13 Overcomes shortcoming of Markov Models
0:32:09 Generality of the Attentive State-Space Models
0:33:00 ASSM: A General, Versatile and Clinically Actionable Models
0:35:19 Morbidity networks: Personalized and dynamic
0:36:49 Learning Dynamic and Personalized Comorbidity Network Event Data using Deep Diffusion Processes
0:38:12 A tool for decision support and discovery
0:39:01 Part 4: Personalized Monitoring and Screening
0:40:36 Personalized Screening/Monitoring: Who to Screen? When to Screen? What to Screen?
0:41:48 Deep Sensing: Active Sensing Using Multi-directional Recurrent Neural Networks
0:44:35 Deep Sensing Architecture
0:46:58 Temporal Phenotyping using Deep Predicting clustering of Disease Progression [Lee, vdS, ICML 2020]
0:50:10 Part 5: Personalized medicine needs to go beyond risk predictions-Individualized Treatment Recommendations
0:50:40 RCTs do not support Personalized Medicine
0:51:55 Delivering Personalized (Individualized) Treatments
0:52:17 Goal: Learning Individualized Treatment/Causal Effect from Observational Data
0:52:58 Our Goal
0:53:12 Potential outcomes framework
0:54:33 Assumptions
0:55:38 The learning problem
0:56:05 Beyond supervised learning...
0:56:24 Causal modeling not equal to predictive modeling
0:57:29 Very active area of research - Many works on treatment effects
0:57:36 Modeling ITEs: Key questions
0:58:01 ITE models: A classification
0:59:22 Many ITE estimation models!
0:59:59 A first theory for causal inference - individualized treatment
1:00:40 Bayesian non parametric ITE estimation
1:02:42 Minimax Rate for ITE Estimation
1:05:19 Should we care about selection bias?
1:06:29 Theory guides model design
1:06:42 Multi-task Gaussian processes
1:07:06 ITE using Non-stationary Gaussian Processes
1:08:21 Multiple Treatments: GANITE
1:09:36 How to select the best model?
1:10:38 Does it matter?
1:11:21 When to treat? How to treat? When to stop?
1:11:48 Counterfactual Recurrent Network
1:12:43 Q&A
1:20:11 Part 6?: Integration: Can we have a unified end-to-end pipeline?
1:20:16 Clairvoyance: A unified, end-to-end pipeline for clinical decision
1:21:13 Part 7: How do we get data? Synthetic Data
1:21:47 Why do we care? We need data!
1:22:11 What can be done?
1:22:34 Why differential privacy?
1:23:18 NeurIPS 2020: Hide-and-Seek Privacy Challenge
1:24:52 A Revolution in Healthcare is taking place
1:25:36 Machine Learning & Healthcare: Vision
1:26:17 Acknowledgements
1:26:54 Q&A
Видео Machine Learning for Healthcare, part 2 - Mihaela van der Schaar - MLSS 2020, Tübingen канала virtual mlss2020
Показать
Комментарии отсутствуют
Информация о видео
Другие видео канала
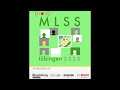
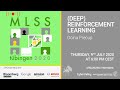
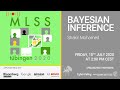

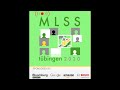
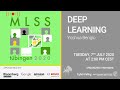


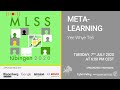
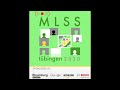
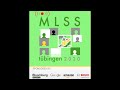

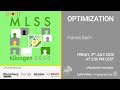
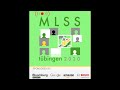
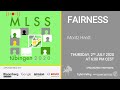
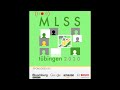
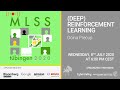
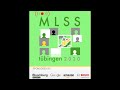
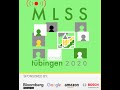
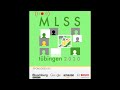
