Mastering Fuzzy Name Matching in R: From Vectors to Data Frames
Learn how to effectively match two long character vectors in R using fuzzy string matching techniques with practical examples to manage variations in names.
---
This video is based on the question https://stackoverflow.com/q/68985944/ asked by the user 'Dominic Gohla' ( https://stackoverflow.com/u/11476777/ ) and on the answer https://stackoverflow.com/a/68987698/ provided by the user 'phiver' ( https://stackoverflow.com/u/4985176/ ) at 'Stack Overflow' website. Thanks to these great users and Stackexchange community for their contributions.
Visit these links for original content and any more details, such as alternate solutions, latest updates/developments on topic, comments, revision history etc. For example, the original title of the Question was: Fuzzy matching two long character vectors in R
Also, Content (except music) licensed under CC BY-SA https://meta.stackexchange.com/help/licensing
The original Question post is licensed under the 'CC BY-SA 4.0' ( https://creativecommons.org/licenses/by-sa/4.0/ ) license, and the original Answer post is licensed under the 'CC BY-SA 4.0' ( https://creativecommons.org/licenses/by-sa/4.0/ ) license.
If anything seems off to you, please feel free to write me at vlogize [AT] gmail [DOT] com.
---
Mastering Fuzzy Name Matching in R: From Vectors to Data Frames
When working with large character vectors in R, it's common to face the challenge of fuzzy matching names that may vary slightly due to differences in formatting, including middle names, titles, or even spelling variations. In this guide, we will explore a practical solution to match two datasets containing the names of electoral candidates and incumbents while accounting for these discrepancies.
The Problem
You have two data frames in R:
Candidates containing roughly 45,000 names of electoral candidates.
Incumbents featuring about 7,600 names of members of parliament.
Your goal is to check whether each name in the Candidates data frame exists in the Incumbents data frame, creating a new column called incumbent that indicates with a 1 (for yes) or 0 (for no) if a match is found. However, direct name matching does not yield reliable results due to the variations in how names are presented.
Example Vectors
Here's an illustration of the problem using smaller datasets:
[[See Video to Reveal this Text or Code Snippet]]
Expected Result
Your output should look something like this:
[[See Video to Reveal this Text or Code Snippet]]
The Solution
Fortunately, R provides a useful package called fuzzyjoin that can handle the fuzzy matching of character vectors seamlessly. Below, let's dive into the process step-by-step.
Step 1: Install Required Packages
First, ensure you have the necessary packages installed and loaded. Use the following commands to install and load fuzzyjoin and dplyr:
[[See Video to Reveal this Text or Code Snippet]]
Step 2: Perform the Fuzzy Matching
Now, let's use the stringdist_left_join() function from fuzzyjoin to match the names from both data frames. We will specify the method of comparison and the maximum distance allowed for matching:
[[See Video to Reveal this Text or Code Snippet]]
In this code:
method = "jw" utilizes the Jaro-Winkler distance, which is suitable for matching names with minor mistakes.
max_dist = 0.2 allows for a maximum distance of 20%, which can be adjusted based on your observations and requirements.
Step 3: Cleaning Up Names
In certain cases, you may need to clean up names, such as removing titles (e.g., "Sir"). You can do this using the gsub() function or stringr::str_remove() before performing the join to ensure better matching.
Step 4: Including Additional Variables
If your datasets have additional identifying information, such as party affiliations, you can include those in the matching process. Here's an updated example for handling that:
[[See Video to Reveal this Text or Code Snippet]]
Then perform the join, taking both name and party into account:
[[See Video to Reveal this Text or Code Snippet]]
Final Output
This will yield an output DataFrame showing which candidates are incumbents based on both name and party affiliation, providing a more refined match.
Conclusion
Fuzzy matching in R is a powerful technique that allows us to connect datasets even when names are not exactly the same. The fuzzyjoin package greatly simplifies the process, enabling you to provide better insights from your data. Whether you are working with political candidates, record linking, or any other field requiring such comparisons, mastering these techniques can save you a lot of time and ensure the accuracy of your results.
Remember to adjust your matching criteria based on your specific data characteristics and to verify results meticulously. Happy coding!
Видео Mastering Fuzzy Name Matching in R: From Vectors to Data Frames канала vlogize
---
This video is based on the question https://stackoverflow.com/q/68985944/ asked by the user 'Dominic Gohla' ( https://stackoverflow.com/u/11476777/ ) and on the answer https://stackoverflow.com/a/68987698/ provided by the user 'phiver' ( https://stackoverflow.com/u/4985176/ ) at 'Stack Overflow' website. Thanks to these great users and Stackexchange community for their contributions.
Visit these links for original content and any more details, such as alternate solutions, latest updates/developments on topic, comments, revision history etc. For example, the original title of the Question was: Fuzzy matching two long character vectors in R
Also, Content (except music) licensed under CC BY-SA https://meta.stackexchange.com/help/licensing
The original Question post is licensed under the 'CC BY-SA 4.0' ( https://creativecommons.org/licenses/by-sa/4.0/ ) license, and the original Answer post is licensed under the 'CC BY-SA 4.0' ( https://creativecommons.org/licenses/by-sa/4.0/ ) license.
If anything seems off to you, please feel free to write me at vlogize [AT] gmail [DOT] com.
---
Mastering Fuzzy Name Matching in R: From Vectors to Data Frames
When working with large character vectors in R, it's common to face the challenge of fuzzy matching names that may vary slightly due to differences in formatting, including middle names, titles, or even spelling variations. In this guide, we will explore a practical solution to match two datasets containing the names of electoral candidates and incumbents while accounting for these discrepancies.
The Problem
You have two data frames in R:
Candidates containing roughly 45,000 names of electoral candidates.
Incumbents featuring about 7,600 names of members of parliament.
Your goal is to check whether each name in the Candidates data frame exists in the Incumbents data frame, creating a new column called incumbent that indicates with a 1 (for yes) or 0 (for no) if a match is found. However, direct name matching does not yield reliable results due to the variations in how names are presented.
Example Vectors
Here's an illustration of the problem using smaller datasets:
[[See Video to Reveal this Text or Code Snippet]]
Expected Result
Your output should look something like this:
[[See Video to Reveal this Text or Code Snippet]]
The Solution
Fortunately, R provides a useful package called fuzzyjoin that can handle the fuzzy matching of character vectors seamlessly. Below, let's dive into the process step-by-step.
Step 1: Install Required Packages
First, ensure you have the necessary packages installed and loaded. Use the following commands to install and load fuzzyjoin and dplyr:
[[See Video to Reveal this Text or Code Snippet]]
Step 2: Perform the Fuzzy Matching
Now, let's use the stringdist_left_join() function from fuzzyjoin to match the names from both data frames. We will specify the method of comparison and the maximum distance allowed for matching:
[[See Video to Reveal this Text or Code Snippet]]
In this code:
method = "jw" utilizes the Jaro-Winkler distance, which is suitable for matching names with minor mistakes.
max_dist = 0.2 allows for a maximum distance of 20%, which can be adjusted based on your observations and requirements.
Step 3: Cleaning Up Names
In certain cases, you may need to clean up names, such as removing titles (e.g., "Sir"). You can do this using the gsub() function or stringr::str_remove() before performing the join to ensure better matching.
Step 4: Including Additional Variables
If your datasets have additional identifying information, such as party affiliations, you can include those in the matching process. Here's an updated example for handling that:
[[See Video to Reveal this Text or Code Snippet]]
Then perform the join, taking both name and party into account:
[[See Video to Reveal this Text or Code Snippet]]
Final Output
This will yield an output DataFrame showing which candidates are incumbents based on both name and party affiliation, providing a more refined match.
Conclusion
Fuzzy matching in R is a powerful technique that allows us to connect datasets even when names are not exactly the same. The fuzzyjoin package greatly simplifies the process, enabling you to provide better insights from your data. Whether you are working with political candidates, record linking, or any other field requiring such comparisons, mastering these techniques can save you a lot of time and ensure the accuracy of your results.
Remember to adjust your matching criteria based on your specific data characteristics and to verify results meticulously. Happy coding!
Видео Mastering Fuzzy Name Matching in R: From Vectors to Data Frames канала vlogize
Комментарии отсутствуют
Информация о видео
5 апреля 2025 г. 9:59:51
00:02:52
Другие видео канала

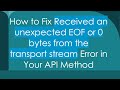
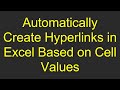
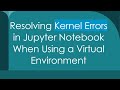

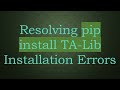

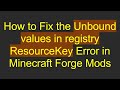
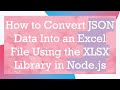

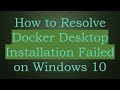


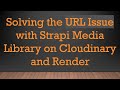
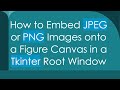

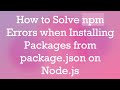
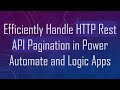
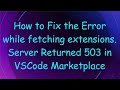

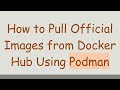